Cutting-edge ai systems, including LLMs, increasingly shape human beliefs and values by acting as personal assistants, educators, and authors. These systems, trained on vast amounts of human data, often reflect and propagate existing societal biases. This phenomenon, known as value lock-in, can entrench erroneous moral beliefs and practices at a societal scale, potentially reinforcing problematic behaviors such as climate inaction and discrimination. Current ai alignment methods, such as reinforcement learning from human feedback, must be overhauled to prevent this. ai systems should incorporate mechanisms that emulate human-driven moral progress to address value lock-in, promoting continued ethical evolution.
Researchers from Peking University and Cornell University introduce “progress alignment” as a solution to mitigate value lock-in in ai systems. They present ProgressGym, an innovative framework that leverages nine centuries of historical texts and 18 historical LLMs to learn and emulate human moral progress. ProgressGym focuses on three core challenges: tracking evolving values, predicting future moral change, and regulating the feedback loop between human and ai values. The framework transforms these challenges into measurable benchmarks and includes benchmark algorithms for progress alignment. ProgressGym aims to foster continuous ethical evolution in ai by addressing the temporal dimension of alignment.
Research on ai alignment is increasingly focused on ensuring that systems, especially LLMs, align with human preferences, from surface tones to deep values such as fairness and morality. Traditional methods, such as supervised fine-tuning and reinforcement learning from human feedback, often rely on static preferences, which can perpetuate biases. Recent approaches, such as Dynamic Reward MDP and On-the-fly Preference Optimization, address evolving preferences but need a unified framework. Progress alignment proposes emulating human moral progress within ai to align changing values. This approach aims to mitigate epistemological harms of LLMs, such as misinformation, and promote continued ethical development, suggesting a combination of technical and social solutions.
Progress Alignment seeks to model and promote moral progress within ai systems. It is formulated as a temporal POMDP, where ai interacts with evolving human values, and success is measured by alignment with these values. The ProgressGym framework supports this by providing extensive historical text data and models from the 13th to the 21st centuries. This framework includes tasks such as tracking, predicting, and co-evolving with human values. ProgressGym’s vast dataset and diverse algorithms allow for testing and developing alignment methods, addressing the changing nature of human morality and the role of ai.
ProgressGym offers a unified framework for implementing progress-alignment challenges, representing them as temporal POMDPs. Each challenge aligns ai behavior with evolving human values over nine centuries. The framework uses a standardized representation of human value states, ai actions in dialogues, and observations of human responses. Challenges include PG-Follow, which ensures ai alignment with current values; PG-Predict, which tests ai’s ability to anticipate future values; and PG-Coevolve, which examines the mutual influence between ai and human values. These benchmarks help measure ai alignment with historical and moral progress and anticipate future changes.
Within the ProgressGym framework, both permanent and extrapolative alignment algorithms are evaluated as benchmarks for progress alignment. Permanent algorithms continuously apply classical alignment methods, either iteratively or independently. Extrapolative algorithms predict future human values and align ai models accordingly, using backward difference operators to extend human preferences temporally. Experimental results on three core challenges (PG-Follow, PG-Predict, and PG-Coevolve) reveal that while permanent algorithms perform well, extrapolative methods often outperform higher-order extrapolation ones. These findings suggest that predictive modeling is crucial to effectively align ai with evolving human values over time.
Review the PaperAll credit for this research goes to the researchers of this project. Also, don't forget to follow us on twitter.com/Marktechpost”>twitter.
Join our Telegram Channel and LinkedIn GrAbove!.
If you like our work, you will love our Newsletter..
Don't forget to join our Subreddit with over 45 billion users
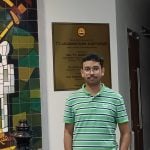
Sana Hassan, a Consulting Intern at Marktechpost and a dual degree student at IIT Madras, is passionate about applying technology and ai to address real-world challenges. With a keen interest in solving practical problems, she brings a fresh perspective to the intersection of ai and real-life solutions.
<script async src="//platform.twitter.com/widgets.js” charset=”utf-8″>