Endogeneity presents a significant challenge when making causal inferences in observational settings. Researchers in social sciences, statistics, and related fields have developed several identification strategies to overcome this obstacle by recreating the natural conditions of experiments. The instrumental variables (IV) method has become a leading approach as researchers discover IVs in diverse settings and justify their compliance with exclusion restrictions. However, these exclusion restrictions are fundamentally untestable assumptions, often based on context-specific rhetorical arguments. The process of identifying possible IVs requires counterfactual reasoning, creativity, and sometimes luck from researchers, which contributes to the heuristic nature of human-led research. This subjective, non-statistical approach to IV selection and justification highlights the need for more rigorous and systematic methods in causal inference.
Large language models (LLMs) have become a promising tool for discovering new IVs in causal inference research. A researcher at the University of Bristol shows that these ai systems, with their advanced language processing capabilities, can help search for valid IVs and provide rhetorical justifications, similar to human researchers, but at an exponentially faster rate. LLMs can explore a vast search space, conduct systematic hypothesis searches, and engage in counterfactual reasoning, making them well suited for causal inference tasks. This ai-assisted approach offers several benefits: it enables rapid and systematic searches adaptable to specific research environments, increases the likelihood of obtaining multiple IVs for formal validity testing, and improves the chances of finding or guiding the construction of relevant data containing IVs. The proposed method involves carefully constructing prompts that guide LLMs in searching for valid IV candidates, incorporating verbal translations of exclusion constraints and employing role-playing techniques to mimic agents' decision-making processes.
The proposed methodology employs OpenAI's ChatGPT-4 (GPT4) to discover IVs in three well-known examples from empirical economics: returns to schooling, production functions, and peer effects. The approach involves constructing specific cues that guide GPT4 in searching for valid IV candidates, incorporating verbal translations of exclusion constraints, and using role-playing techniques to simulate agents' decision-making processes. This method has successfully generated lists of candidate IVs, including unique suggestions and variables popularly used in the literature, along with rationales for their validity. The concept extends beyond discovery IV to other methods of causal inference, such as the search for control variables in regression and difference-in-differences methods and the identification of running variables in regression discontinuity designs. While the lists generated are not definitive, they serve as valuable reference points to inspire researchers about possible variables and domains to explore. Dialogue with GPT4 can also help researchers refine arguments for the validity of variables, emphasizing the potential for collaboration between human researchers and ai to improve causal inference methodologies.
The proposed methodology employs a two-step approach for IV discovery using LLM. In Step 1, the LLM is asked to find IVs that satisfy the verbal descriptions of exclusion restriction (i) and relevance condition. Step 2 refines the search by selecting IVs from Step 1 that meet the verbal description of exclusion restriction (ii). Both steps involve counterfactual statements and require the LLM to provide rationale for their answers. This two-step approach offers several advantages: it improves LLM performance by breaking down complex tasks, allows the user to inspect intermediate results, and provides valuable information through these intermediate results. Indications are initially constructed without covariates for simplicity, and more realistic indications incorporating covariates are later introduced. This method creates a flexible framework for IV discovery, allowing for adjustments and adaptation to specific research contexts, while maintaining a systematic approach to causal inference.
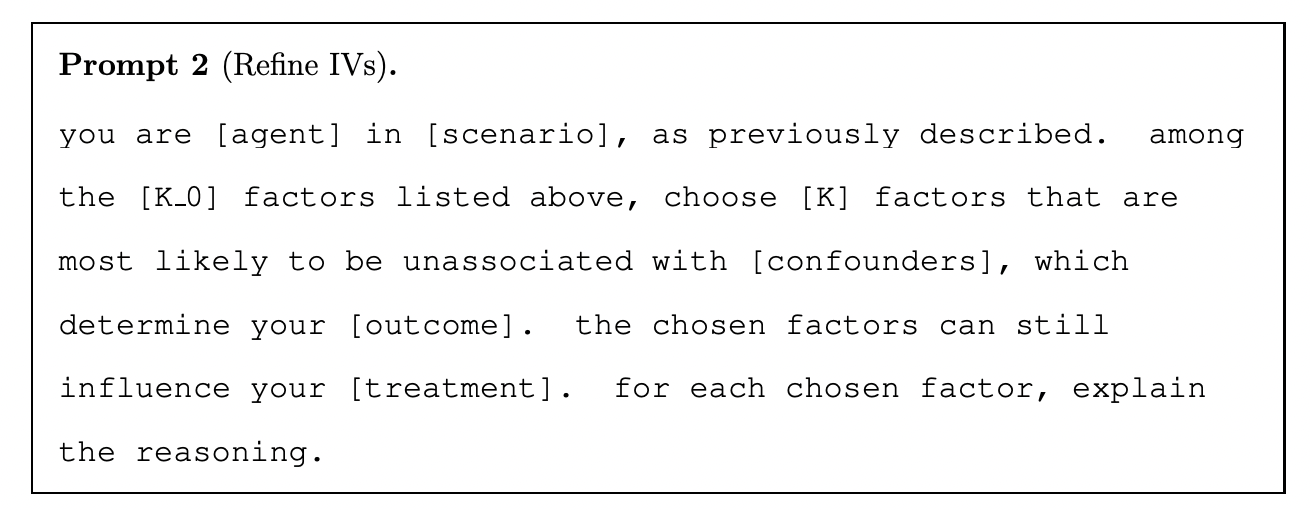
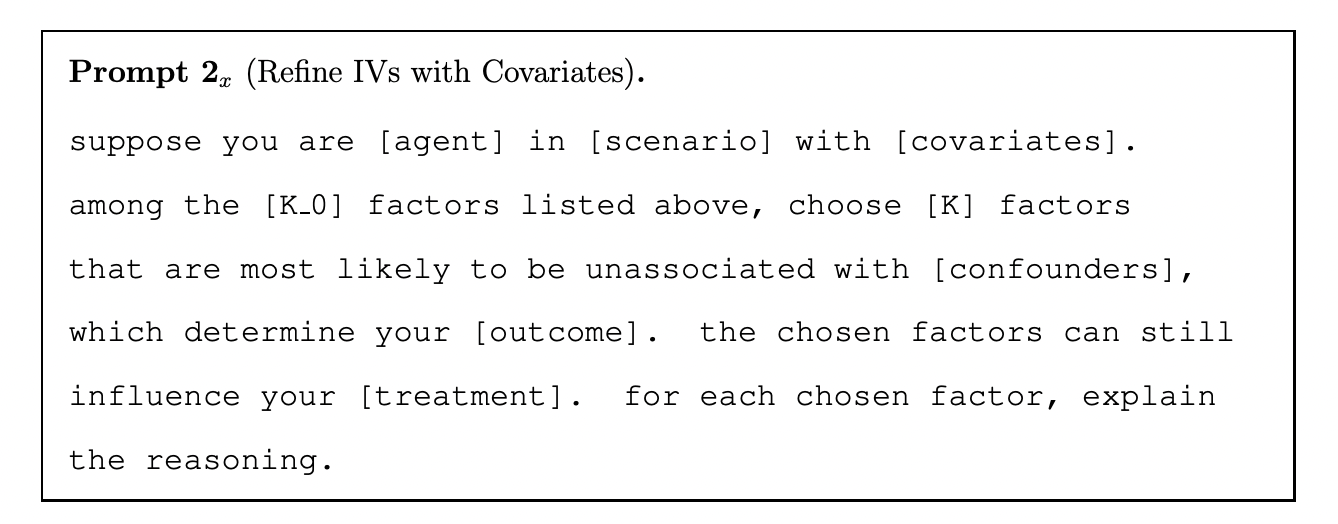
This research serves as a basis for integrating LLM in the discovery of instrumental variables in causal inference. Future directions for sophistication include incorporating known IVs from the literature to guide LLMs in discovering new ones, potentially using learning from few opportunities to improve performance. Furthermore, exploring methods for aggregating results across multiple LLM sessions could take into account and exploit the inherent randomness in LLM results. These advances could lead to more robust and comprehensive IV discovery processes. As ai continues to evolve, collaboration between human researchers and ai systems on causal inference methodologies promises to open new avenues for more efficient and insightful empirical research in economics and related fields.
look at the Paper. All credit for this research goes to the researchers of this project. Also, don't forget to follow us on twitter.com/Marktechpost”>twitter and join our Telegram channel and LinkedIn Grabove. If you like our work, you will love our information sheet.. Don't forget to join our SubReddit over 50,000ml
Are you interested in promoting your company, product, service or event to over 1 million ai developers and researchers? Let's collaborate!
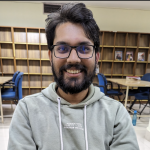
Asjad is an internal consultant at Marktechpost. He is pursuing B.tech in Mechanical Engineering from Indian Institute of technology, Kharagpur. Asjad is a machine learning and deep learning enthusiast who is always researching applications of machine learning in healthcare.
<script async src="//platform.twitter.com/widgets.js” charset=”utf-8″>