Base models show promise in medicine, especially for assisting in complex tasks such as medical decision making (MDM). MDM is a nuanced process that requires doctors to analyze diverse data sources, such as images, electronic medical records, and genetic information, while adapting to new medical research. LLMs could support MDM by synthesizing clinical data and enabling probabilistic and causal reasoning. However, the application of LLM in healthcare remains challenging due to the need for adaptive and multi-tiered approaches. Although multi-agent LLMs show potential in other fields, their current design lacks integration with the collaborative and stepwise decision making essential for effective clinical use.
LLMs are increasingly being applied to medical tasks, such as answering medical exam questions, predicting clinical risks, diagnosing, generating reports, and creating psychiatric evaluations. Improvements in medical LLMs primarily come from training with specialized data or using inference-time methods such as rapid engineering and recall augmented generation (RAG). General-purpose models, such as GPT-4, perform well in medical testing using advanced indications. Multi-agent frameworks improve accuracy, with agents collaborating or debating to solve complex tasks. However, existing static frameworks can limit performance on various tasks, so a dynamic multi-agent approach can better support complex medical decision making.
MIT, Google Research, and Seoul National University Hospital developed Medical Decision-Making Agents (MDAgents), a multi-agent framework designed to dynamically allocate collaboration between LLMs based on the complexity of medical tasks, mimicking decision-making. real-world medical decisions. MDA agents adaptively choose individual or team collaboration tailored to specific tasks, performing well across multiple medical benchmarks. It outperformed previous methods on 7 out of 10 benchmarks, achieving up to a 4.2% improvement in accuracy. Key steps include assessing task complexity, selecting appropriate agents, and synthesizing responses; group reviews improve accuracy by 11.8%. MDAgents also balances performance with efficiency by fine-tuning agent usage.
The MDAgents framework is structured around four key stages in medical decision making. It begins by evaluating the complexity of a medical consultation, classifying it as low, moderate or high. Based on this evaluation, the appropriate experts are hired: a single doctor for the simplest cases or a multidisciplinary team for the most complex ones. The analysis stage then uses different approaches based on the complexity of the case, ranging from individual assessments to collaborative discussions. Finally, the system synthesizes all the knowledge to make a conclusive decision, with accurate results indicating the effectiveness of MDA agents compared to single-agent and other multi-agent configurations at various medical benchmarks.
The study evaluates the framework and reference models in various medical benchmarks under individual, group and adaptive conditions, showing remarkable robustness and efficiency. The adaptive method, MDAgents, effectively adjusts inference based on task complexity and consistently outperforms other configurations on seven out of ten benchmarks. Researchers testing data sets such as MedQA and Path-VQA find that adaptive complexity selection improves decision accuracy. By incorporating MedRAG and moderator review, accuracy improves by up to 11.8%. Furthermore, the framework's resilience to parameter changes, including temperature adjustments, highlights its adaptability for complex medical decision-making tasks.
In conclusion, the study presents MDAgents, a framework that enhances the role of LLMs in medical decision making by structuring their collaboration based on task complexity. Inspired by the dynamics of clinical consultations, MDA agents assign LLMs to individual or group roles as needed, with the goal of improving diagnostic accuracy. Testing on ten medical benchmarks shows that MDA agents outperform other methods on seven tasks, with an accuracy gain of up to 4.2% (p < 0.05). Ablation studies reveal that combining moderator reviews and outside medical knowledge in group settings increases accuracy by an average of 11.8%, underscoring the potential of MDA agents in clinical diagnosis.
look at the Paper. All credit for this research goes to the researchers of this project. Also, don't forget to follow us on twitter.com/Marktechpost”>twitter and join our Telegram channel and LinkedIn Grabove. If you like our work, you will love our information sheet.. Don't forget to join our SubReddit over 55,000ml.
(Sponsorship opportunity with us) Promote your research/product/webinar to over 1 million monthly readers and over 500,000 community members
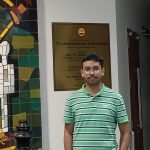
Sana Hassan, a consulting intern at Marktechpost and a dual degree student at IIT Madras, is passionate about applying technology and artificial intelligence to address real-world challenges. With a strong interest in solving practical problems, he brings a new perspective to the intersection of ai and real-life solutions.
<script async src="//platform.twitter.com/widgets.js” charset=”utf-8″>