Key points:
In today's changing educational landscape, school leaders and educators face increasingly complex challenges. The infusion of data analytics and the rise of generative artificial intelligence (ai) have given rise to new opportunities for more efficient and effective decision making. While these tools are promising, they come with some critical considerations.
As we move toward an era where ai can quickly process and analyze large sets of data, school leaders must adopt a data-driven, rather than data-driven, mindset. This distinction is key to ensuring that human action remains at the forefront of decision making. The goal should be to use data not as a sole determinant but as a supporting tool that enhances the judgment, creativity, and expertise of educational leaders.
One of the fundamental principles of data-driven decision making is the validity and reliability of the data being used. Leaders must be vigilant in examining data sources to ensure they are free of bias and accurately represent the contexts in which decisions will be applied. In educational settings, data can be derived from a variety of sources: student assessments, attendance records, teacher evaluations, behavioral data, etc.
For example, consider a scenario in which a district uses standardized test scores to evaluate teacher performance. If the data is skewed by socioeconomic factors or if the test itself is biased against certain groups, it could lead to unfair evaluations. To mitigate this, leaders should look for various data points, including qualitative feedback from students and teachers, classroom observations, and contextual factors such as community resources. The key is to ensure that decisions made with this data are equitable and reflect the real needs and abilities of students and educators. The potential efficiencies of quantitative data can encourage the use of such data sets to drive strategic and operational decisions, but qualitative data can help nuance numerical data and provide leaders with valuable context.
In the context of machine-interpreted data, educators should be cautious. ai systems trained on biased data can perpetuate or even exacerbate inequalities. For example, if an ai-powered tool is used to recommend personalized learning paths for students based on historical data, it could disproportionately suggest lower-level material to students from underserved communities if past data reflects past systemic biases. Therefore, leaders must prioritize equity and inclusion by ensuring that the data they feed into ai systems is vetted for bias.
When considering how data informs leadership, a useful framework is Bolman and Deal's four leadership frameworks: structural, human resources, political, and symbolic. These frameworks provide a lens through which educational leaders can view and manage their schools, making it easier to incorporate data into decisions without losing sight of broader organizational needs and challenges.
The structural framework focuses on the organizational mission. Data can be used to streamline operations and optimize resource allocation. For example, an analysis of enrollment trends and demographic data could help a district decide where to build new schools or allocate more funds. ai can improve these processes by quickly analyzing large amounts of demographic and logistics data, allowing leaders to make informed decisions faster.
The human resources framework emphasizes the needs of people within the organization. In education, this means paying attention to the well-being and professional growth of teachers and staff. Data from staff surveys, career development assessments, and retention rates can guide decisions about hiring practices, mentoring programs, and wellness initiatives. Leaders must ensure that ai-enhanced systems increase human well-being rather than reduce it to mere efficiency metrics. For example, data from ai tools can help identify teachers who may need additional support or highlight areas for collaborative development within teaching teams.
The political framework deals with power dynamics and conflicting interests and needs. In the educational context, decisions often involve multiple stakeholders: parents, teachers, administrators, unions, and community members. Data can play a critical role in navigating these relationships, helping leaders understand the diverse needs and priorities of different groups. For example, data from community surveys or student performance metrics could help school boards justify allocating funds to specific programs. ai could be used to model potential outcomes based on different budget decisions, providing leaders with data-backed evidence to present to stakeholders.
The symbolic framework emphasizes organizational and potentially community culture, meaning, and inspiration. Educational leaders must ensure that the data they use and the decisions they make align with the values and mission of the school or district. For example, a school that prides itself on inclusivity could analyze data to ensure that extracurricular programs are accessible to all students, regardless of their background. artificial intelligence tools can help by analyzing participation data and identifying any patterns of exclusion, allowing leaders to make symbolic decisions that uphold the values of the institution.
A fundamental concept in the realm of ai-enhanced decision making is the difference between being data-driven and data-driven. Being data-driven involves making decisions based solely on data, often reducing complex human behaviors and needs to mere numbers or variables. In contrast, a data-driven approach uses data as one of many tools to guide decisions, allowing human judgment, experience, and creativity to play a vital role.
For example, consider a school that decides whether to implement a new curriculum based on student performance data. A data-driven approach might focus solely on test results, ignoring factors such as teacher input, student engagement, and resource availability. In contrast, a data-driven approach would incorporate these additional factors, using the data to guide but not dictate the final decision. This approach ensures that educational decisions are made with a comprehensive understanding of the context, not based solely on numbers.
In the era of generative ai, maintaining human agency becomes crucial. ai systems can offer powerful insights, but they should not be considered infallible. Educators and leaders must critically evaluate recommendations generated by ai tools, ensuring they align with educational values and the unique needs of students. For example, an ai tool might suggest placing a student in a lower-level math class based on historical performance data, but a teacher might know that the student has recently shown significant improvement or has external factors affecting performance. that ai cannot explain.
As ai becomes more integrated into educational decision-making, the ethics of the process should be a primary consideration. Leaders must ensure that the ai tools they use are transparent, that data is protected, and that decisions made using ai are fair and equitable. For example, if an ai system is used to monitor student behavior or engagement, leaders should ensure that it does not infringe on student privacy or disproportionately target certain groups of students. Additionally, leaders must be transparent about how ai is used in their schools, providing students, parents, and teachers with clear information about how decisions are made and what data is used.
Educational leaders must also be proactive in providing professional development opportunities for teachers and staff to understand how ai works and how it can be used responsibly. This ensures that educators at all levels are aware of the potential benefits of ai and its limitations.
Data-driven decision making, particularly when complemented by ai, offers enormous potential for educational leadership. Leaders must approach these tools with a critical eye, ensuring that data is valid, unbiased, and used to enhance, rather than replace, human judgment. By adopting frameworks such as Bolman and Deal's Four Leadership Frameworks, educators can ensure that data informs decisions in a way that aligns with the values and mission of their institution, while maintaining the well-being of students and the staff in the foreground. In this way, the education sector can harness the power of ai responsibly, creating learning environments that are both innovative and inclusive.
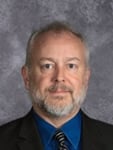
!function(f,b,e,v,n,t,s)
{if(f.fbq)return;n=f.fbq=function(){n.callMethod?
n.callMethod.apply(n,arguments):n.queue.push(arguments)};
if(!f._fbq)f._fbq=n;n.push=n;n.loaded=!0;n.version=’2.0′;
n.queue=();t=b.createElement(e);t.async=!0;
t.src=v;s=b.getElementsByTagName(e)(0);
s.parentNode.insertBefore(t,s)}(window, document,’script’,
‘https://connect.facebook.net/en_US/fbevents.js’);
fbq(‘init’, ‘6079750752134785’);
fbq(‘track’, ‘PageView’);