LLMs are advancing healthcare by offering new possibilities in clinical support, especially through tools like Microsoft's BioGPT and Google's Med-PaLM. Despite these innovations, LLMs in healthcare face a significant challenge: aligning with the professionalism and accuracy required for real-world diagnoses. This gap is particularly crucial under FDA regulations for software as a medical device (SaMD), where LLMs must demonstrate specialized expertise. Current models, designed for general tasks, often need to meet the clinical standards required for life-critical healthcare settings, making their professional integration an ongoing challenge.
LLMs have made progress in processing unstructured medical data. However, concerns about their domain-specific expertise in critical clinical settings should be addressed. Recent works, such as ZODIAC, aim to address these limitations by focusing on cardiological diagnosis. Multi-agent frameworks, widely used in healthcare to manage complex workflows, show promise in optimizing tasks such as patient care coordination. However, cardiology diagnostic systems have mainly relied on rule-based or single-agent models, and deep learning models are recently advancing. The incorporation of LLM in cardiology remains an underexplored area in which this work seeks to advance.
Researchers from ZBeats Inc., New York University and other institutions introduce ZODIAC, an LLM-powered system designed to achieve cardiologist-level professionalism in cardiology diagnosis. ZODIAC helps by extracting key patient data, detecting arrhythmias, and generating preliminary reports for expert review. Built on a multi-agent framework, ZODIAC processes multimodal data and is fine-tuned with real-world inputs verified by cardiologists. Rigorous clinical validation shows that ZODIAC outperforms leading models such as GPT-4o and BioGPT. Successfully integrated into electrocardiography devices, ZODIAC sets a new standard for aligning LLMs with SaMD regulations, ensuring safety and accuracy in medical practice.
The ZODIAC framework is designed for cardiologist-level diagnostics using a multi-agent system that processes multi-modal patient data. It collects biostatistics, tabular metrics, and ECG tracings, analyzing different agents. One agent interprets tabular metrics, while another evaluates ECG images and generates clinical findings. A third agent synthesizes these findings with clinical guidelines to create a diagnostic report. The process, validated by cardiologists, aligns with real-world medical practices and meets regulatory standards for SaMD, ensuring accuracy and professional compliance during hospital implementations.
Clinical validation experiments follow real-world settings and focus on eight evaluation metrics. Five metrics evaluate the quality of clinical outcomes, while three focus on safety. Cardiologists were hired to evaluate the ZODIAC framework, rating it on a scale of one to five using anonymous models to avoid bias. ZODIAC outperformed the general and physician-specialist models, excelling in clinical professionalism and safety. Subgroup analysis revealed consistent diagnostic performance of ZODIAC across diverse populations. An ablation study confirmed the importance of adjustment and learning in context, and ZODIAC also demonstrated high stability in repeated diagnostic results.
In conclusion, the study presents ZODIAC, an advanced LLM-driven framework for cardiology diagnosis, aimed at improving collaboration between physicians and LLM. Using data validated by cardiologists, ZODIAC employs instructional tuning, in-context learning, and fact-checking to provide diagnoses comparable to those of human specialists. Clinical validation reveals ZODIAC's superior performance across diverse patient demographics and arrhythmia types, outperforming leading models such as OpenAI's GPT-4o and Microsoft's BioGPT. The framework's multi-agent collaboration processes diverse patient data, leading to accurate arrhythmia detection and preliminary report generation, marking a significant advancement in the integration of LLM into medical devices, including electrocardiography equipment.
look at the Paper. All credit for this research goes to the researchers of this project. Also, don't forget to follow us on twitter.com/Marktechpost”>twitter and join our Telegram channel and LinkedIn Grabove. If you like our work, you will love our information sheet.. Don't forget to join our SubReddit over 50,000ml
(Next Event: Oct 17, 202) RetrieveX – The GenAI Data Recovery Conference (Promoted)
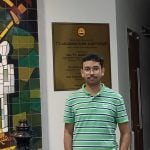
Sana Hassan, a consulting intern at Marktechpost and a dual degree student at IIT Madras, is passionate about applying technology and artificial intelligence to address real-world challenges. With a strong interest in solving practical problems, he brings a new perspective to the intersection of ai and real-life solutions.
<script async src="//platform.twitter.com/widgets.js” charset=”utf-8″>