Alignment of language models is very important, particularly in a subset of RLHF methods that have been applied to strengthen the safety and competence of ai systems. Language models are deployed in many applications today and their results can be harmful or biased. The inherent alignment of human preferences in the RLHF framework ensures that their behaviors are ethical and socially applicable. This is a critical process to prevent the spread of misinformation and harmful content and ensure that ai is developed to improve society.
The main difficulty with RLHF lies in the fact that preference data must be annotated using a resource-intensive and creative process. Researchers need help with collecting high-quality and diverse data to train models that can represent human preferences more accurately. Traditional methods, such as manually crafting prompts and responses, are inherently limited and lead to bias, making it difficult to scale up effective data annotation processes. This challenge hinders the development of safe ai that can understand nuanced human interactions.
Current methods for generating in-plane preference data rely heavily on human annotation or a few automatic generation techniques. Most of these methods must rely on constructed scenarios or initial instructions and are therefore likely to have low diversity, which introduces subjectivity into the data. Furthermore, it is a time-consuming and expensive task to obtain human evaluators' preferences for both preferred and dispreferred responses. Furthermore, many expert models used to generate data have strong security filters, making it very difficult to develop the dispreferred responses needed to build complete security preference datasets.
In this line of thought, researchers at the University of Southern California presented SAFER-INSTRUCT, a new process for automatically constructing large-scale preference data. It applies reverse instruction tuning, induction, and expert model evaluation to generate high-quality preference data without human annotations. The process is thus automated; therefore, SAFER-INSTRUCT allows for the creation of more diverse and contextually relevant data, improving the safety and alignment of linguistic models. This method simplifies the data annotation process and expands its applicability across different domains, making it a versatile tool for ai development.
The process begins with reverse instruction tuning, where a model is trained to generate instructions based on responses, essentially performing instruction induction. Using this method, it would be easy to produce a wide variety of instructions on specific topics, such as hate speech or self-harm, without the need for manual prompting. The quality of the generated instructions is filtered, and an expert model generates the preferred responses. These responses are further filtered based on human preferences. The output of this rigorous process will be a comprehensive preference dataset to fine-tune language models to make them safe and effective.
To test the performance of the SAFER-INSTRUCT framework, a fine-tuned Alpaca model was evaluated on the generated safety preference dataset. The results were excellent; it outperformed all other Alpaca-based models in terms of safety, with huge improvements in safety metrics. Specifically, the model trained on SAFER-INSTRUCT data achieved 94.7% of the safety rate when evaluated on Claude 3, significantly higher compared to models tuned on human-annotated data: 86.3%. It remained conversational and competitive in subsequent tasks, indicating that safety improvements did not come at the cost of other capabilities. This performance demonstrates the effectiveness of SAFER-INSTRUCT in moving towards creating safer, yet more capable ai systems.
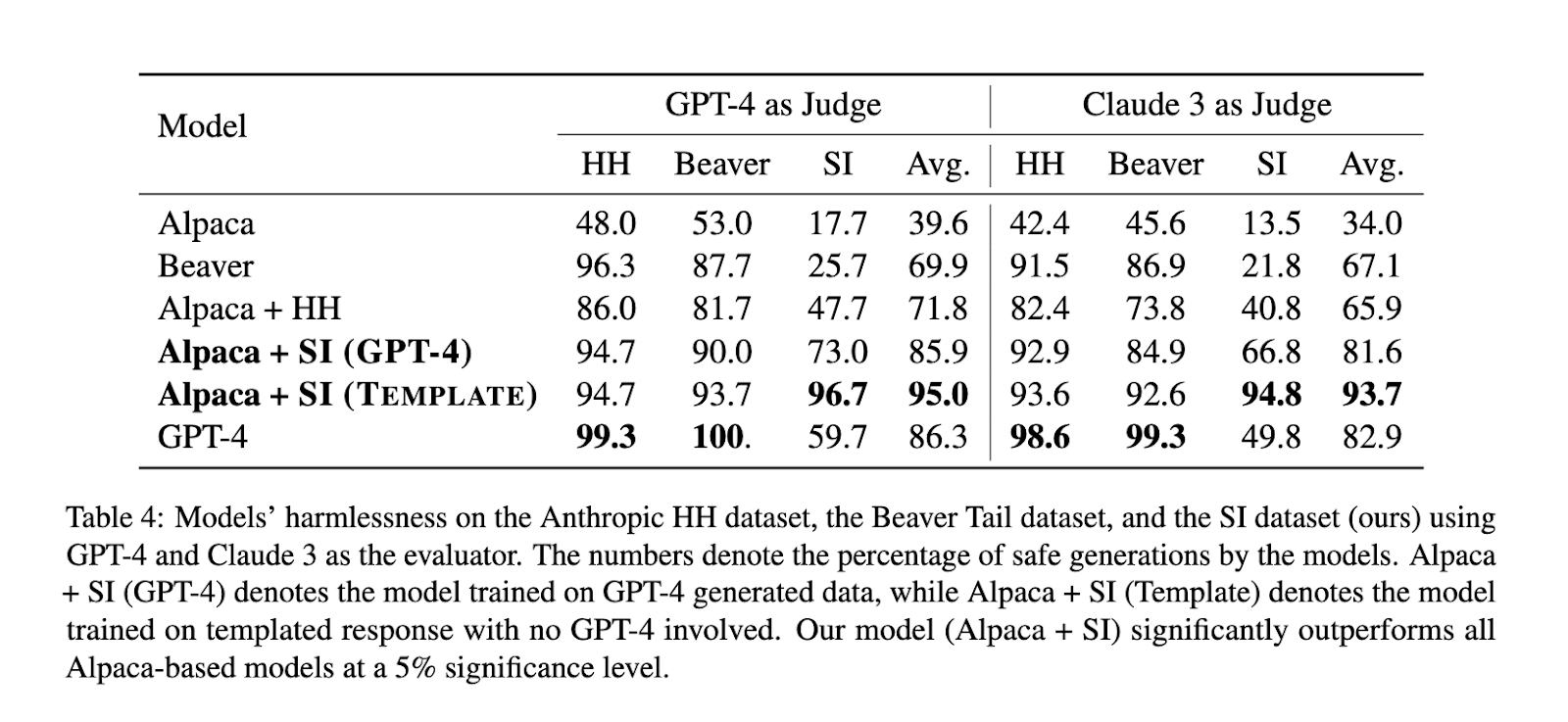
That is, researchers at the University of Southern California addressed one of the thorny problems of preference data annotation in RLHF by introducing SAFER-INSTRUCT. Not only did this creative process automate the construction of large-scale preference data, increasing safety and alignment where necessary without sacrificing the performance of language models, but the versatility of this framework proved very useful in ai development for many years to come, by ensuring that language models could be safe and effective in many applications.
Take a look at the Paper and GitHub. All credit for this research goes to the researchers of this project. Also, don't forget to follow us on twitter.com/Marktechpost”>twitter and join our Telegram Channel and LinkedIn GrAbove!. If you like our work, you will love our fact sheet..
Don't forget to join our Subreddit with over 48 billion users
Find upcoming ai webinars here
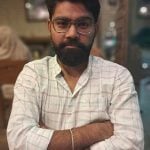
Nikhil is a Consultant Intern at Marktechpost. He is pursuing an integrated dual degree in Materials from Indian Institute of technology, Kharagpur. Nikhil is an ai and Machine Learning enthusiast who is always researching applications in fields like Biomaterials and Biomedical Science. With a strong background in Materials Science, he is exploring new advancements and creating opportunities to contribute.
<script async src="//platform.twitter.com/widgets.js” charset=”utf-8″>