Despite the growing interest in multiple agents (MAS) systems, where multiple LLM -based agents collaborate in complex tasks, their performance profits remain limited compared to single agent frames. While the mass in software engineering, discovery of drugs and scientific simulations are explored, they often fight with coordination inefficiencies, leading to high failure rates. These failures reveal key challenges, including the misalignment of tasks, reasoning action mismatches and ineffective verification mechanisms. Empirical evaluations show that even the last generation open source mass, such as Chatdev, can exhibit low success rates, asking questions about their reliability. Unlike single -agent frames, the dough must address the misalignment between agents, conversation reset and the verification of incomplete tasks, which significantly affect their effectiveness. In addition, current best practices, such as the best s sampling, often exceed the mass, emphasizing the need for a deeper understanding of its limitations.
Existing research has addressed specific challenges in agent systems, such as improving workflow memory, improving state control and refining communication flows. However, these approaches do not offer a holistic strategy to improve more reliability in all domains. Although several reference points evaluate the agent -based agent systems, security and reliability, there is no consensus on how to build a robust mass. Previous studies highlight the risks of complicating agent frames and survive the importance of modular design, however, systematic investigations in the foul failure ways remain scarce. This work contributes to providing a structured taxonomy of more failures and suggesting design principles to improve its reliability, racing the path for LLM systems of multiple more effective agents.
Investigators at UC Berkeley and Intessa Sanpaolo present the first comprehensive study of MAS challenges, analyzing five frames in 150 tasks with expert scorers. They identify 14 failure modes, categorized in system design failures, misalignment between agents and task verification problems, forming the multiple system failure taxonomy (MASft). They develop a LLM-A-A-Jex pipe to facilitate evaluation, achieving a high agreement with human scorers. Despite the interventions such as the improved agent specification and orchestration, MAS failures persist, underlining the need for structural redesign. His work, including data and annotations sets, has an open source to guide the future research and development of MAS.
The study explores the fault patterns in Mas and classifies them in a structured taxonomy. Using the founded theory approach (GT), researchers analyze the execution of more iteratively traces, refining the categories of failures through studies of integrated agreements. They developed a LLM -based scorer for automated fault detection, achieving 94%accuracy. Failures are classified as system design failures, misalignment between agents and inappropriate verification of tasks. Taxonomy is validated through iterative refinement, ensuring reliability. The results highlight the various fault modes among the more architectures, emphasizing the need for better coordination, lighter roles definitions and solid verification mechanisms to improve the performance of more.
Strategies are classified in tactical and structural approaches to improve mass and reduce failures. Tactical methods imply the refining of indications, agents organization, interaction management and improvement of clarity and verification steps. However, its effectiveness varies. Structural strategies focus on improvements throughout the system, such as verification mechanisms, standardized communication, reinforcement learning and memory management. Two case studies, Mathcat and Chatdev, avoid these approaches. MathChat refines the indications and roles of the agents, improving the results inconsistent. Chatdev improves adherence to role and modifies the topology of the frame for iterative verification. While these interventions help, significant improvements require deeper structural modifications, emphasizing the need for greater research in reliability.
In conclusion, the study comprehensively analyzes mass failure modes using LLM. When examining more than 150 traces, the investigation identifies 14 different fault modes: System specification and design, misalignment between agents and verification and termination of tasks. An automated LLM scorer is introduced to analyze more traces, which demonstrates reliability. Case studies reveal that simple solutions often fall short, which requires structural strategies for consistent improvements. Despite the growing interest in the mass, its performance remains limited compared to the systems of unique agents, which underlines the need for a deeper investigation into the coordination of agents, verification and communication strategies.
Verify he Paper. All credit for this investigation goes to the researchers of this project. In addition, feel free to follow us <a target="_blank" href="https://x.com/intent/follow?screen_name=marktechpost” target=”_blank” rel=”noreferrer noopener”>twitter And don't forget to join our 85k+ ml of submen.
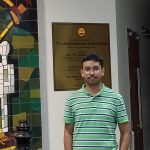
Sana Hassan, a consulting intern in Marktechpost and double grade student in Iit Madras, passionate to apply technology and ai to address real world challenges. With great interest in solving practical problems, it provides a new perspective to the intersection of ai and real -life solutions.