In the rapidly evolving field of natural language processing, the capabilities of large language models have grown exponentially. Researchers and organizations around the world are continually pushing the boundaries of these models to improve their performance in various natural language generation and understanding tasks. A critical aspect of the advancement of these models is the quality of the training data on which they are based. In this article, we delve into a research paper that addresses the challenge of improving open source language models using mixed quality data. This research explores the proposed method, technology, and implications for natural language processing.
Mixed quality data, including expert-generated and suboptimal data, poses a significant challenge in training language models. The expert data generated by state-of-the-art models like GPT-4 are typically of high quality and serve as a gold standard for training. On the other hand, suboptimal data originating from older models like GPT-3.5 can be of lower quality and present challenges during training. This research under discussion recognizes this mixed quality data scenario and aims to improve the instruction tracing capabilities of open source language models.
Before delving into the proposed method, let us briefly mention the current methods and tools used in training language models. A common approach to improve these models is supervised fine tuning (SFT). In SFT, models are trained on instruction-following tasks using high-quality data generated by experts, which guides the generation of correct responses. Additionally, reinforcement learning fine-tuning (RLFT) methods have gained popularity. RLFT involves collecting preference feedback from humans and training models to maximize rewards based on these preferences.
Tsinghua University proposed an innovative method in its research paper: OpenChat. OpenChat is an innovative framework that improves open source language models using mixed quality data. At its core is conditioned reinforcement learning fine-tuning (C-RLFT), a novel training method that simplifies the training process and reduces reliance on reward models.
C-RLFT enriches the input information for language models by distinguishing between different data sources based on their quality. This distinction is achieved through the implementation of a class-conditioned policy. The policy helps the model differentiate between expert-generated data (high quality) and suboptimal data (lower quality). By doing so, C-RLFT provides explicit signals to the model, allowing it to improve its instruction following capabilities.
The performance of OpenChat, specifically the open chat-13 b model, has been evaluated on several benchmarks. One of the most prominent benchmarks used is AlpacaEval, where the model’s abilities to follow instructions are tested. Openchat-13b shows remarkable results, outperforming other 13 billion parameter open source models such as LLaMA-2. It achieves higher success rates and superior performance in instruction-following tasks, demonstrating the effectiveness of the C-RLFT method.

The importance of data quality is an important aspect highlighted by the research team. Despite its limited amount, expert data plays a crucial role in improving the performance of language models. The ability to differentiate between expert and suboptimal data, coupled with the C-RLFT method, leads to substantial improvements in model performance. This finding underscores the importance of retaining high-quality training data to ensure the success of language model training.
Implications and future research
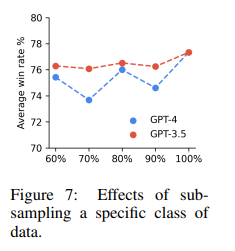
The OpenChat framework and the C-RLFT method are promising for the future of natural language processing. This approach opens new avenues for research and development by simplifying the training process and reducing reliance on complex reward models. It also addresses the challenge of mixed quality data, making it more accessible to leverage diverse training data sets effectively.
In conclusion, OpenChat presents an innovative solution to enhance open source language models with mixed quality data. By introducing the C-RLFT method, this approach achieves superior instruction-following capabilities, as demonstrated by its performance on benchmarks. As natural language processing continues to evolve, innovative techniques like OpenChat pave the way for more efficient and effective language model training.
Review the Paper. All credit for this research goes to the researchers of this project. Also, don’t forget to join. our SubReddit of more than 30,000 ml, Facebook community of more than 40,000 people, Discord Channel, and Electronic newsletterwhere we share the latest news on ai research, interesting ai projects and more.
If you like our work, you’ll love our newsletter.
Madhur Garg is a consulting intern at MarktechPost. He is currently pursuing his Bachelor’s degree in Civil and Environmental Engineering from the Indian Institute of technology (IIT), Patna. He shares a great passion for machine learning and enjoys exploring the latest advances in technologies and their practical applications. With a keen interest in artificial intelligence and its various applications, Madhur is determined to contribute to the field of data science and harness the potential impact of it in various industries.
<!– ai CONTENT END 2 –>