Introduction
In the business arena, financial reporting and analysis are critical to strategic decision-making and operational oversight. These processes provide senior management and stakeholders with key insights into a company’s performance, financial health, and future prospects. Traditionally, financial reporting and analysis was time-consuming and required specialized skills to interpret complex data and generate actionable business intelligence. As businesses grow and data volumes increase, there is a growing need for more efficient, accurate, and accessible financial reporting methods.
The emergence of artificial intelligence (ai) in finance has radically changed this landscape. ai has evolved from automating routine tasks to enabling sophisticated predictive analytics, transforming financial processes. Natural language generation (NLG), a specialized branch of ai, has proven particularly innovative. NLG generates human-like text from data, turning raw financial numbers into clear and coherent narrative reports. This advancement streamlines reporting and improves the interpretability of financial data, making it easier for decision makers—even those without deep financial expertise—to understand and act on key insights.
This article explores the impact of NLG on financial analysis and reporting. We examine how it transforms complex financial data into clear narratives, improving accessibility for senior management. Our goal is to showcase the strategic value of NLG by providing leaders with actionable insights. Ultimately, we demonstrate how NLG supports more informed decision making and strategic planning in the financial domain.
Overview
- Financial analysis and reporting are crucial to strategic decision making and traditionally require expertise to interpret complex data and generate useful insights.
- The rise of ai in finance, particularly NLG, transforms data into human-like narrative reports, improving accessibility and decision-making for stakeholders.
- NLG automates the generation of financial narratives, ensuring efficiency, accuracy and scalability in reporting complex financial data.
- Case studies demonstrate the application of NLG in automating profit and loss reporting, providing executives with timely insights for strategic planning.
- Despite its benefits, NLG in financial reporting faces challenges such as data security, ethical considerations, and limitations in nuanced analysis.
<h2 class="wp-block-heading" id="h-transforming-financial-reporting-with-ai“>Transforming financial reporting with ai
Natural Language Generation (NLG) is a significant advancement in ai that converts structured data into coherent, human-like text. Unlike ai, which interprets language, NLG creates narrative content. This capability produces clear reports and explanations from complex data, making it a powerful business intelligence tool.
NLG has evolved from early computer experiments to sophisticated systems powered by deep learning and neural networks. These systems now produce texts that closely resemble human writing and adapt their output based on context, audience, and specific needs.
Read also: Build a Natural Language Generation (NLG) System with PyTorch
Understanding and Mechanism of NLG in Financial Reporting
In financial reporting, NLG transforms raw data into actionable information. The process begins with analyzing financial data, using statistical analysis and trend detection to identify key patterns. This analysis forms the basis for narratives that reflect the financial health of the company. NLG systems then use linguistic models to produce accurate and understandable text. Advanced NLG systems go beyond data presentation, offering contextual explanations and deeper insights into trends and their future implications. This customization aligns the generated reports with the needs of senior management, making NLG crucial for strategic decision-making.
Natural Language Generation (NLG) offers significant advantages in financial commentary, transforming the communication of financial information. Key benefits include:
- Efficiency: NLG automates the generation of financial narratives, dramatically reducing the time and human effort required, enabling faster decision making based on timely information.
- Accuracy: By processing data directly, NLG minimizes the risk of human error, ensuring financial reporting is accurate and reliable.
- Scalability: NLG can handle increasing data complexities, enabling organizations to efficiently manage and process information from multiple sources without sacrificing quality.
- Personalization: NLG customizes financial reporting to meet the specific needs of senior management, highlighting the financial metrics most relevant to strategic objectives.
- Accessibility: NLG turns complex financial data into understandable narratives, making financial information accessible to all stakeholders, regardless of their financial experience.
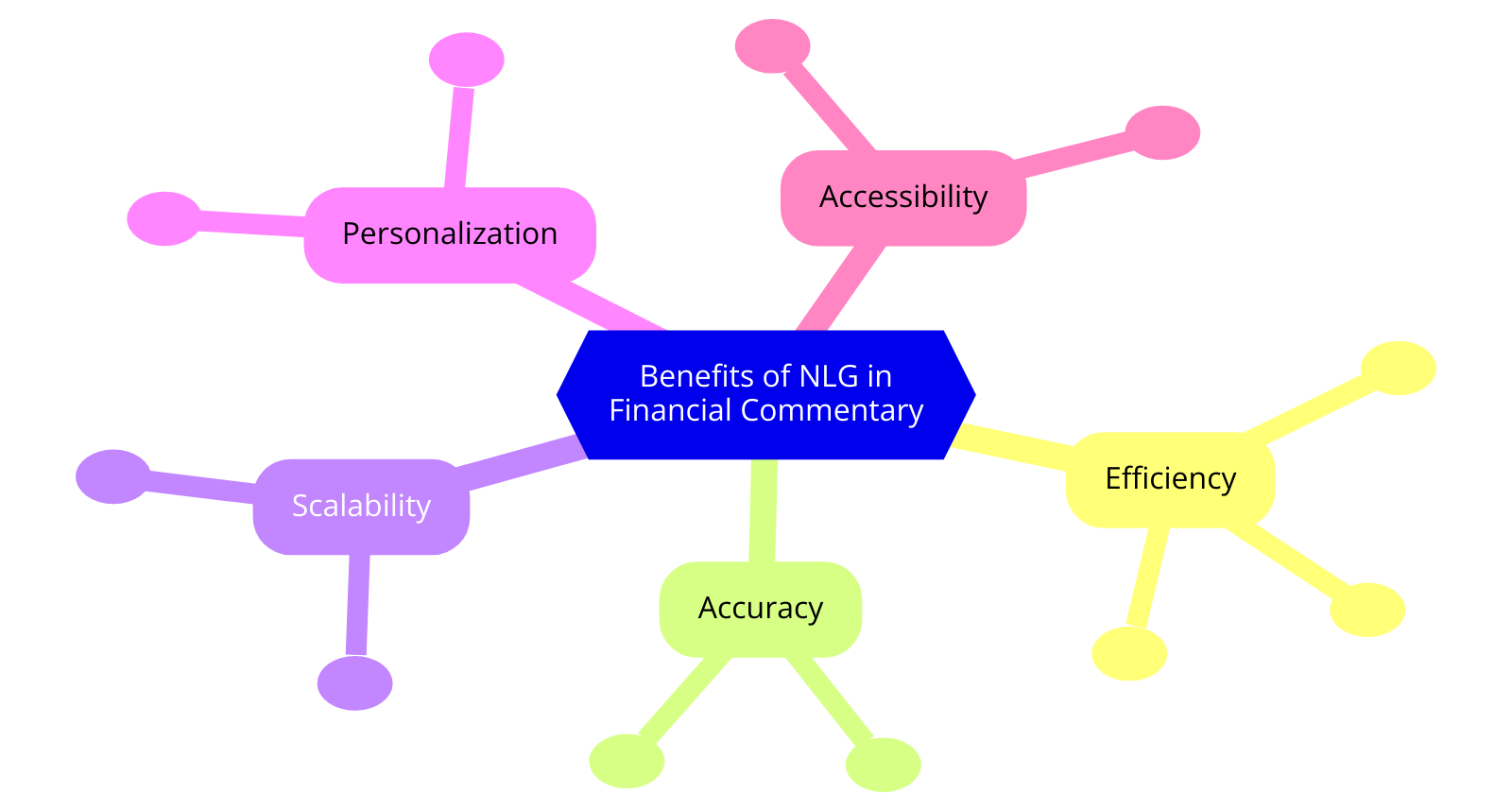
Practical cases and applications in financial reporting
Finance units rely heavily on data-driven insights to generate accurate performance reports. Departments such as Planning and Performance Management are tasked with reviewing monthly forecasts, comparing actuals to plans, and documenting deviations. Natural Language Generation (NLG) can significantly improve this process by automating predictions based on extensive historical data.
Consider a scenario where a finance unit aims to automate the generation and publication of profit and loss (P&L) reports with variance analysis for executive reporting. Key metrics include business revenue, cost of sales, and total expenses, which are crucial for calculating net profit, a vital indicator for executives monitoring financial trends.
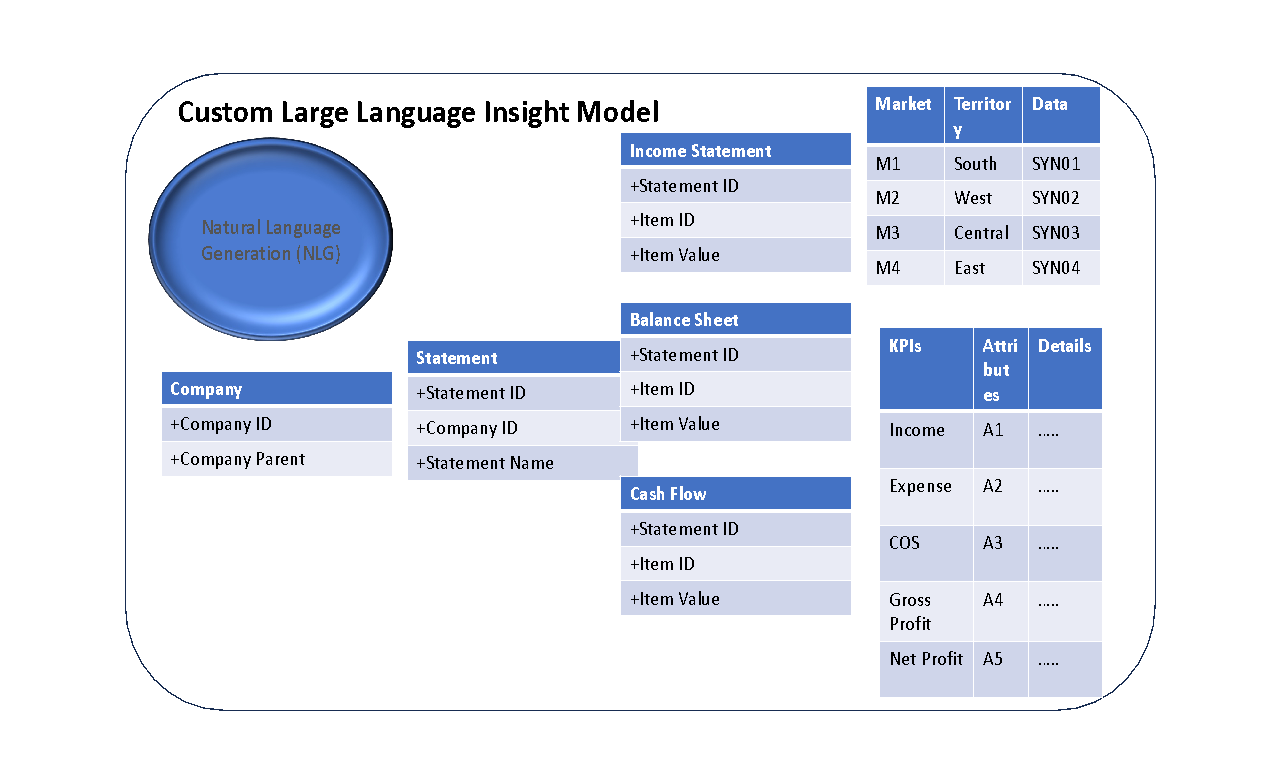
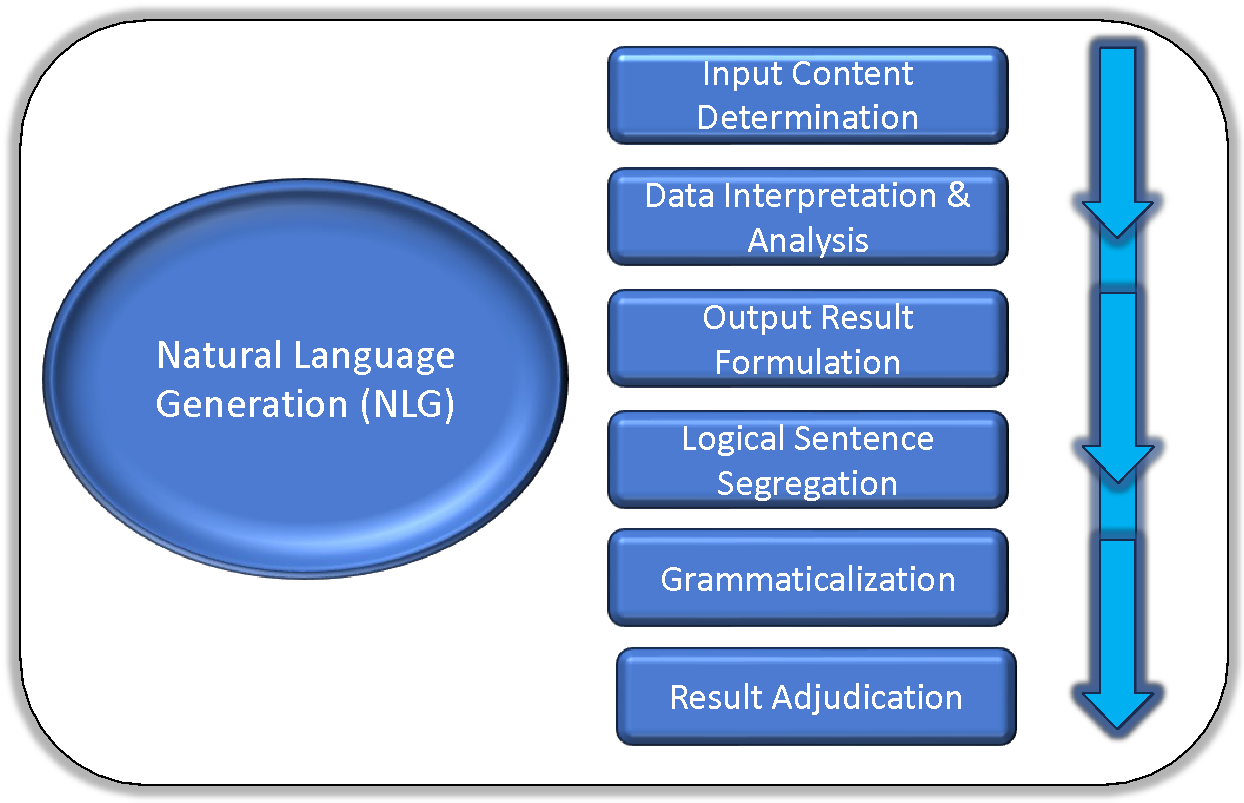
To achieve this, a rich, data-centric model is developed that incorporates at least five years of historical data. This model serves as the foundation for NLG, which leverages ai and machine learning to interpret data, recognize patterns, and generate human-like text. The process includes determining input content, interpreting data, formulating results, structuring sentences, and grammaticalizing. The end result is an accurate and well-organized financial report that includes a narrative explaining deviations and trends, providing valuable insights for executive decision-making.
This approach not only improves efficiency and accuracy, but also enables scalability and customization in financial reporting.
<h2 class="wp-block-heading" id="h-challenges-and-limitations-of-financial-reporting-with-ai“>Challenges and Limitations of ai-Powered Financial Reporting
While NLG improves financial reporting, it faces several challenges and limitations. Technical complexities involve protecting sensitive financial data, which requires strong encryption, secure storage, and strict access controls. Ethical concerns include ensuring transparency and avoiding bias in the narratives generated by NLG to maintain accurate representations of financial health.
NLG also struggles to understand complex financial nuances, such as the impact of geopolitical events or non-quantifiable factors such as brand value. This limitation requires human oversight to ensure contextualized and nuanced analysis. In addition, NLG systems can produce homogenized views, without the diverse interpretations offered by human analysts.
Read also: How to become a financial analyst?
Conclusion
NLG has reimagined financial reporting, turning complex data into meaningful narratives that are easier to understand and action. By automating feedback, it brings a new level of efficiency and accuracy, making financial analysis more personalized and accessible. This technology provides senior management with timely, personalized information that guides decisions. As ai evolves, NLG will play an even more important role, delivering deeper insights that support more thoughtful and informed decisions across organizations.
References
- Kasula, BY (2016). Advances and applications of artificial intelligence: A comprehensive review. International Journal of Statistical Computing and Simulation, 8(1), 1-7.
- Bindra, P., Kshirsagar, M., Ryan, C., Vaidya, G., Gupt, K.K., & Kshirsagar, V. (2021). Perspectives on advances in artificial intelligence and machine learning, current state of the art and future prospects: Seven decades of digital revolution. In Intelligent Computing Techniques and Applications: Proceedings of the Fourth International Conference on Intelligent Computing and Informatics, Volume 1 (pp. 609–621). Springer Singapore
- Shyam Patel, “Service Virtualization in SAP ERP: A Comprehensive Approach to Improve Business Operations and Sustainability,” International Journal of Computer Trends and technology, Vol. 71, No. 5, pp. 53–56, 2023. Crossref, https://doi.org/10.14445/22312803/IJCTT-V71I5P109
- Ravi Dave, Bidyut Sarkar, Gaurav Singh, “Revolutionizing Business Processes with SAP technology: A Buyer’s Perspective”, International Journal of Computer Trends and technology, Vol. 71, No. 4, pp. 1–7, 2023. Crossref, https://doi.org/10.14445/22312803/IJCTT-V71I4P101
Frequently Asked Questions
A. ai is revolutionizing financial services by automating routine tasks, improving fraud detection, and personalizing customer experiences through predictive analytics.
A. The impact of ai on financial reporting includes automating data analysis, improving accuracy in financial statements, and enhancing transparency through the generation of a clear and consistent narrative.