Generative ai (Gen ai), capable of producing robust content based on input data, is set to impact various sectors such as science, economy, education and environment. Extensive sociotechnical research aims to understand the broad implications, recognizing risks and opportunities. There is debate surrounding the openness of Gen ai models, with some advocating for an open release to benefit everyone. Regulatory developments, in particular the EU ai Law and the US Executive Order, highlight the need to assess risks and opportunities while questions remain about governance and systemic risks.
The discourse around open source generative ai is complex, examining broader impacts and specific debates. The research delves into the benefits and risks in areas such as science and education along with the implications of capacity changes. Discussions focus on categorizing systems according to disclosure levels and addressing ai security. While closed source models still outperform open ones, the gap is narrowing.
Researchers from the University of Oxford, the University of California, Berkeley and other institutes advocate for the responsible development and deployment of open source Gen ai, drawing parallels with the success of open source in traditional software. The study outlines the development stages of Gen ai models and presents a taxonomy for openness, classifying models into fully closed, semi-open, and fully open categories. The discussion assesses risks and opportunities at near-medium and long-term stages, emphasizing benefits such as research empowerment and technical alignment while addressing existential and non-existential risks. Recommendations are provided for policymakers and developers to balance risks and opportunities, promoting appropriate legislation without stifling open source development.
The researchers introduced a rating scale to evaluate the openness of components in generative ai processes. Components are classified as fully closed, semi-open or fully open based on accessibility. A points-based system evaluates licenses, distinguishing between highly restrictive and unrestricted ones. The analysis applies this framework to 45 high-impact large language models (LLMs), revealing a balance between open and closed source components. The findings highlight the need for responsible open source development to leverage the benefits and mitigate the risks effectively. Furthermore, they emphasized the importance of reproducibility in model development.
The study takes a sociotechnical approach, contrasting the impacts of open-source and closed-source independent generative ai models in key areas. Researchers conduct a contrastive analysis, followed by a holistic examination of relative risks. The short to medium term phase is defined, excluding dramatic changes in capacity. Challenges in assessing risks and benefits are discussed along with possible solutions. Sociotechnical analysis considers research, innovation, development, security, equity, access, usability and broader social aspects. The benefits of open source include advancing research, affordability, flexibility, and empowering developers, encouraging innovation.
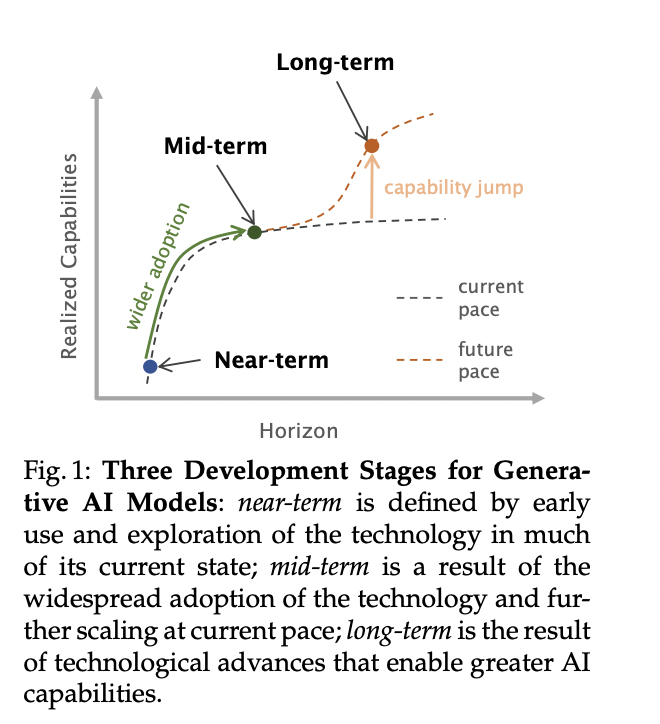
The researchers also discussed existential risk and open sourcing of AGI. The concept of existential risk in ai refers to the potential for AGI to cause human extinction or an irreversible global catastrophe. Previous work suggests several causes, including automated warfare, bioterrorism, rogue ai agents, and cyberwarfare. The speculative nature of AGI makes it impossible to prove or disprove its likelihood of causing human extinction. While existential risk has attracted significant attention, some experts have revised their views on its likelihood. They explore how open source ai could influence the existential risk of AGI in different development scenarios.
In short, the narrowing performance gap between closed source and open source Gen ai models fuels discussions about best practices for open releases to mitigate risks. Discussions focus on categorizing systems according to willingness to disclose and differentiating them to achieve regulatory clarity. Concerns about ai security are intensifying, emphasizing the need for open models to mitigate centralization risks while recognizing greater potential for misuse. The authors propose a robust taxonomy and offer nuanced insights into short-, medium-, and long-term risks, expanding on previous research with comprehensive recommendations for developers.
Review the Paper. All credit for this research goes to the researchers of this project. Also, don't forget to follow us on twitter.com/Marktechpost”>twitter. Join our Telegram channel, Discord channeland LinkedIn Grabove.
If you like our work, you will love our Newsletter..
Don't forget to join our 42k+ ML SubReddit
<figure class="wp-block-embed is-type-rich is-provider-twitter wp-block-embed-twitter“>
Asjad is an internal consultant at Marktechpost. He is pursuing B.tech in Mechanical Engineering at Indian Institute of technology, Kharagpur. Asjad is a machine learning and deep learning enthusiast who is always researching applications of machine learning in healthcare.
<script async src="//platform.twitter.com/widgets.js” charset=”utf-8″>