Introduction
You know how we always hear about “diverse” datasets in machine learning? Well, it turns out there’s been a problem with that. But don’t worry: a brilliant team of researchers just published a game-changing paper that has the entire ML community buzzing. In the paper that recently won the ICML 2024 Best Paper Award, researchers Dora Zhao, Jerone TA Andrews, Orestis Papakyriakopoulos, and Alice Xiang address a critical problem in machine learning (ML): the often vague and unsubstantiated claims about “diversity” in datasets. Their paper, titled “Measure Dataset Diversity, Don’t Just Claim It,” proposes a structured approach to conceptualizing, operationalizing, and assessing diversity in ML datasets using principles from measurement theory.
Now, I know what you’re thinking. “Another paper on dataset diversity? Haven’t we heard that before?” But trust me, this one is different. These researchers have taken a deep look at how we use terms like “diversity,” “quality,” and “bias” without really backing them up. We’ve been playing around with these concepts in a loose way, and we’re getting called out for it.
But best of all, they're not just pointing out the problem, they've developed a solid framework to help us measure and validate diversity claims. They're giving us a toolbox to fix this messy situation.
Buckle up because I’m about to take you on a deep dive into this groundbreaking research. We’ll explore how we can move from claiming diversity to measuring it. Trust me, by the end of this article, you’ll never look at an ML dataset the same way again!
The problem with diversity claims
The authors highlight a widespread problem in the machine learning community: dataset curators frequently use terms like “diversity,” “bias,” and “quality” without clear definitions or validation methods. This lack of precision hinders reproducibility and perpetuates the misconception that datasets are neutral entities rather than value-laden artifacts shaped by the perspectives and social contexts of their creators.
A framework for measuring diversity
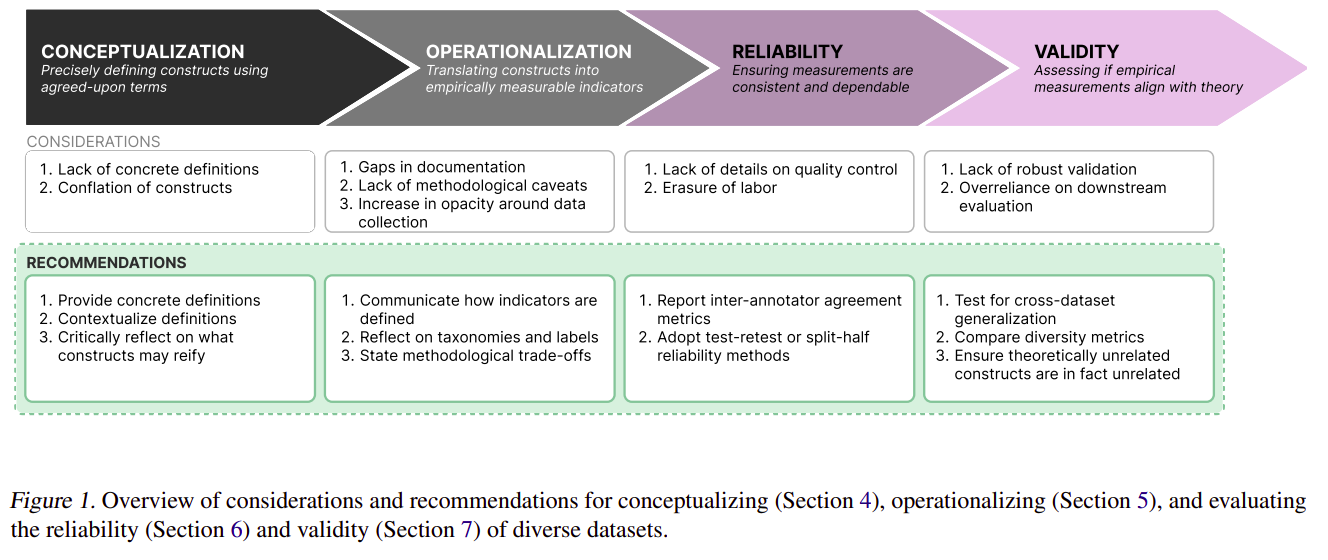
Drawing on social science, particularly measurement theory, the researchers present a framework for transforming abstract notions of diversity into measurable constructs. This approach involves three key steps:
- Conceptualization: Clearly define what “diversity” means in the context of a specific data set.
- Operationalization: Develop concrete methods to measure defined aspects of diversity.
- Assessment: Evaluating the reliability and validity of diversity measures.
In summary, this position paper advocates for clearer definitions and more robust validation methods for creating diverse data sets and proposes measurement theory as a scaffolding framework for this process.
Key findings and recommendations
Through an analysis of 135 image and text datasets, the authors discovered several important insights:
- Lack of clear definitions: Only 52.9% of the datasets explicitly justified the need for diverse data. The article highlights the importance of providing concrete and contextualized definitions of diversity.
- Gaps in documentation: Many articles presenting datasets do not provide detailed information on collection strategies or methodological choices. Authors advocate for greater transparency in dataset documentation.
- Reliability concerns: Only 56.3% of the datasets covered quality control processes. The paper recommends using inter-annotator agreement and test-retest reliability to assess the consistency of the datasets.
- Validity challenges: Claims about diversity often lack robust validation. The authors suggest using construct validity techniques, such as convergent and discriminant validity, to assess whether data sets actually capture the intended diversity of constructs.
Practical Application: The Segment Anything Dataset
To illustrate their framework, the paper includes a case study of the Segment Anything (SA-1B) dataset. While praising certain aspects of SA-1B’s approach to diversity, the authors also identify areas for improvement, such as improving transparency around the data collection process and providing more robust validation of geographic diversity claims.
Broader implications
This research has important implications for the ML community:
- Challenging “thinking at scale”: The article argues against the notion that diversity automatically arises with larger data sets and emphasizes the need for intentional curation.
- Uploading documentation: While advocating for greater transparency, the authors acknowledge the substantial effort required and call for systemic changes in how data work is valued in ML research.
- Temporal considerations: The article highlights the need to take into account how diversity constructs may change over time, affecting the relevance and interpretation of the data set.
You can read the article here: Position: Measure data setGood Diversity, don't just claim to be one
Conclusion
This ICML 2024 paper offers a path toward more rigorous, transparent, and reproducible research by applying measurement theory principles to the creation of ML datasets. As the field grapples with issues of bias and representation, the framework presented here provides valuable tools to ensure that claims of diversity in ML datasets are not just rhetoric, but measurable and meaningful contributions to the development of fair and robust ai systems.
This groundbreaking work serves as a call to action for the ML community to raise standards for dataset curation and documentation, ultimately leading to more trustworthy and equitable machine learning models.
I have to admit, when I first saw the authors’ recommendations for documenting and validating datasets, part of me thought, “Ugh, that sounds like a lot of work.” And yes, it is. But you know what? It’s work that needs to be done. We can’t keep building ai systems on shaky foundations and just hope for the best. But here’s what got me excited: This paper isn’t just about improving our datasets. It’s about making our entire field more rigorous, transparent, and trustworthy. In a world where ai is becoming increasingly influential, that’s huge.
So what do you think? Are you ready to get your hands dirty and start measuring the diversity of your datasets? Let’s chat in the comments. I’d love to hear your thoughts on this groundbreaking research!
You can read other articles from the ICML 2024 Best Paper'is here: ICML 2024 Featured Articles: What's New in Machine Learning.
Frequent questions
Answer: Measuring dataset diversity is crucial because it ensures that the datasets used to train machine learning models represent diverse demographics and scenarios. This helps reduce bias, improve model generalization, and promote fairness and equity in ai systems.
Answer: Diverse datasets can improve the performance of machine learning models by exposing them to a wide range of scenarios and reducing overfitting to any particular group or scenario. This results in more robust and accurate models that perform well across different populations and conditions.
Answer: Common challenges include defining what constitutes diversity, translating these definitions into measurable constructs, and validating diversity claims. Furthermore, ensuring transparency and reproducibility in documenting diversity in datasets can be a time-consuming and complex task.
Answer: Practical steps include:
a. Clearly define the project’s specific diversity objectives and criteria.
b. Collect data from a variety of sources to cover different demographic groups and scenarios.
c. Use standardized methods to measure and document diversity in data sets.
d. Continually evaluate and update data sets to maintain diversity over time.
e.Implement robust validation techniques to ensure that data sets genuinely reflect the intended diversity.