artificial intelligence has grown significantly with the integration of vision and language, allowing systems to interpret and generate information in multiple data modalities. This capacity improves applications such as natural language processing, computer vision and human-computer interaction by allowing IA models to process textual, visual and video entries. However, there are challenges to ensure that these systems provide precise, significant and aligned results, particularly as multimodal models become more complex.
The main difficulty in building great vision language models is to achieve the results produced by them aligning with human preferences. Most existing systems fail due to the production of hallucinated responses and inconsistency in the interaction process within multiple modes, as well as their dependence on the domain of the application. In addition, such high quality data sets are scarce and vary in various types and tasks such as mathematical reasoning, video analysis or following instructions. LVLM cannot deliver the necessary subtlety in real world applications without adequate alignment mechanisms.
Current solutions to these challenges are mainly limited to rewards only text or generative models of narrow scope. These models generally trust manual annotations or patented systems, which are not scalable and non -transparent. In addition, current methods have a limitation with respect to static data sets and predefined indications that cannot capture all real world variability. This results in a great gap between the ability to develop integral reward models that can guide LVLM effectively.
Researchers at the Shanghai artificial intelligence Laboratory, the Chinese University of Hong Kong, the University of Shanghai Jiao Tong, the University of Nanjing, the University of Fudan and the Technological University of Nanyang introduced Internlm-Xcomposer2.5-Reward (IXC-2.5 -Reward). The model is a significant step in the development of multimodal reward models, providing a robust framework to align LVLM results with human preferences. Unlike other solutions, the IXC-2.5-Reward can process different forms, including text, images and videos, and has the potential to function well in varied applications. Therefore, this approach is a great improvement on current tools, taking into account a lack of domain and scalability coverage.
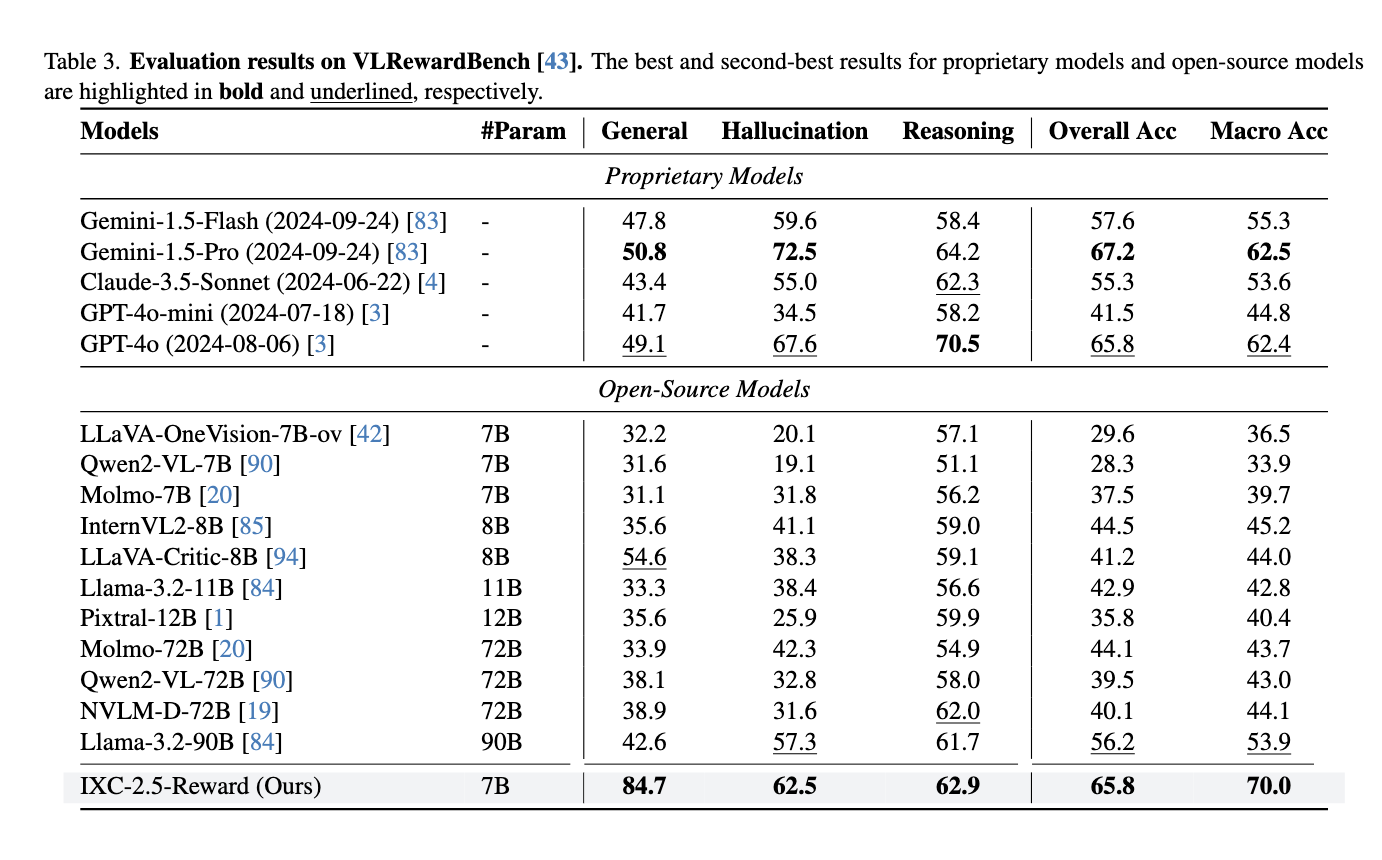
According to the researcher, IXC-2.5-Reward was designed through a set of comprehensive preferences data and includes various domains such as texts, general reasoning and video understanding. The model has a score head that predicts reward scores for indications and responses given. The team used reinforcement learning algorithms such as the optimization of proximal policies (PPO) to train a chat model, IXC-2.5-chaat, to provide high quality responses and aligned by humans. The training was accompanied by open source data and recently collected, which guarantees wide applicability. In addition, the model does not suffer from the common traps of length biases, since it uses restrictions in response lengths to guarantee quality and conciseness in the generated outputs.
The IXC-2.5-Reward performance establishes a new reference point in multimodal. In VL-Rewardbench, the model achieved a general precision of 70.0%, surpassing prominent generative models such as Gemini-1.5-PRO (62.5%) and GPT-4O (62.4%). The system also produced competitive results at only text reference points, obtaining 88.6% in the rewards bank and 68.8% in RM Bank. These results showed that the model could maintain strong language processing capabilities even while performing extremely well in multimodal tasks, and also, incorporating IXC-2.5-Reward in the Chat IXC-2.5-chath model produced great profits in the follow-up of The instructions and configuration of multimodal dialogue, validating the applicability of the reward model in real world scenarios.
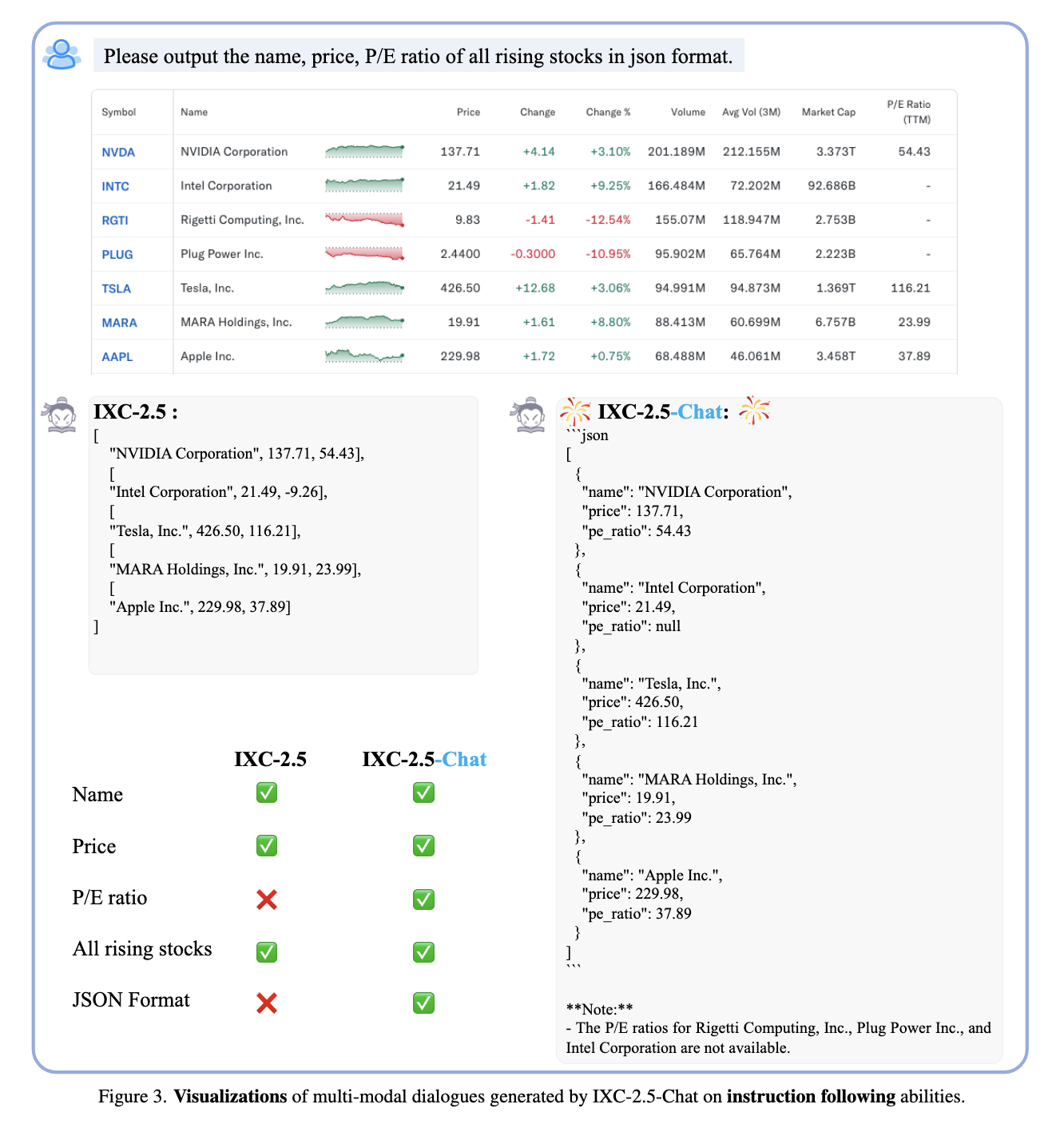
The researchers also showed three applications of IXC-2.5-Reward that underline their versatility. First, it serves as a supervision signal for reinforcement learning, which allows politics optimization techniques such as PPO to train models effectively. Second, the model's proof time scale capabilities allow optimal responses from multiple candidates to be selected, further improving performance. Finally, IXC-2.5-Reward was essential to clean the data and find noise or problems in the data sets, which leaked from training data and, therefore, improved the quality of training data for LVLM .
This work is a great leap forward in multimodal rewards and critical gaps models regarding scalability, versatility and alignment with human preferences. The authors have established the basis of additional advances in this field through various data sets and the application of latest generation reinforcement learning techniques. IXC-2.5-Reward is ready to revolutionize multimodal ai systems and provide more robustness and effectiveness to real world applications.
Verify he Paper and Girub. All credit for this investigation goes to the researchers of this project. Besides, don't forget to follow us <a target="_blank" href="https://x.com/intent/follow?screen_name=marktechpost” target=”_blank” rel=”noreferrer noopener”>twitter and join our Telegram channel and LINKEDIN GRsplash. Do not forget to join our 70k+ ml of submen.
<a target="_blank" href="https://nebius.com/blog/posts/studio-embeddings-vision-and-language-models?utm_medium=newsletter&utm_source=marktechpost&utm_campaign=embedding-post-ai-studio” target=”_blank” rel=”noreferrer noopener”> (Recommended Read) Nebius ai Studio expands with vision models, new language models, inlays and Lora (Promoted)
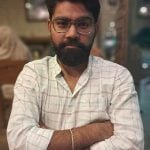
Nikhil is an internal consultant at Marktechpost. He is looking for a double degree integrated into materials at the Indian Institute of technology, Kharagpur. Nikhil is an ai/ML enthusiast who is always investigating applications in fields such as biomaterials and biomedical sciences. With a solid experience in material science, it is exploring new advances and creating opportunities to contribute.