By intertwining the development of artificial intelligence combined with large language models with reinforced learning in high -performance computing, recently developed reasoning language models can go beyond traditional forms of limitation applied to processing by language systems towards Explicit and even structured mechanisms, allowing complex reasoning. solutions in various fields. This achievement in models development is the next important milestone to achieve better knowledge and contextual decisions.
The design and implementation of modern RLM raises many challenges. They are expensive to develop, they have property restrictions and complex architectures that limit their access. In addition, the technical darkness of its operations creates a barrier for organizations and researchers to take advantage of these technologies. The lack of affordable and scalable solutions exacerbates the gap between entities with access to avant -garde models, which limits opportunities for broader innovation and application.
The current RLM implementations are based on complex methodologies to achieve their reasoning capabilities. Techniques such as Monte Carlo Tree Search (MCTS), Beam Search and reinforcement learning concepts such as processes and results -based supervision have been used. However, these methods demand advanced experience and resources, which restricts their usefulness for smaller institutions. While the LLMs such as O1 and O3 of OpenAi provide fundamental capacities, their integration with explicit reasoning frameworks remains limited, which leaves the potential without exploit for a broader implementation.
eth Zurich, BASF SE, Cledar and Cyfronet AGH researchers presented a comprehensive plan to optimize the design and development of RLM. This modular frame unifies various reasoning structures, including chains, trees and graphics, allowing flexible and efficient experimentation. The main innovation of the plan lies in the integration of learning principles by reinforcement with hierarchical reasoning strategies, which allows the construction of scalable and profitable models. As part of this work, the team developed framework X1, a practical implementation tool for researchers and organizations to quickly believe RLM prototypes.
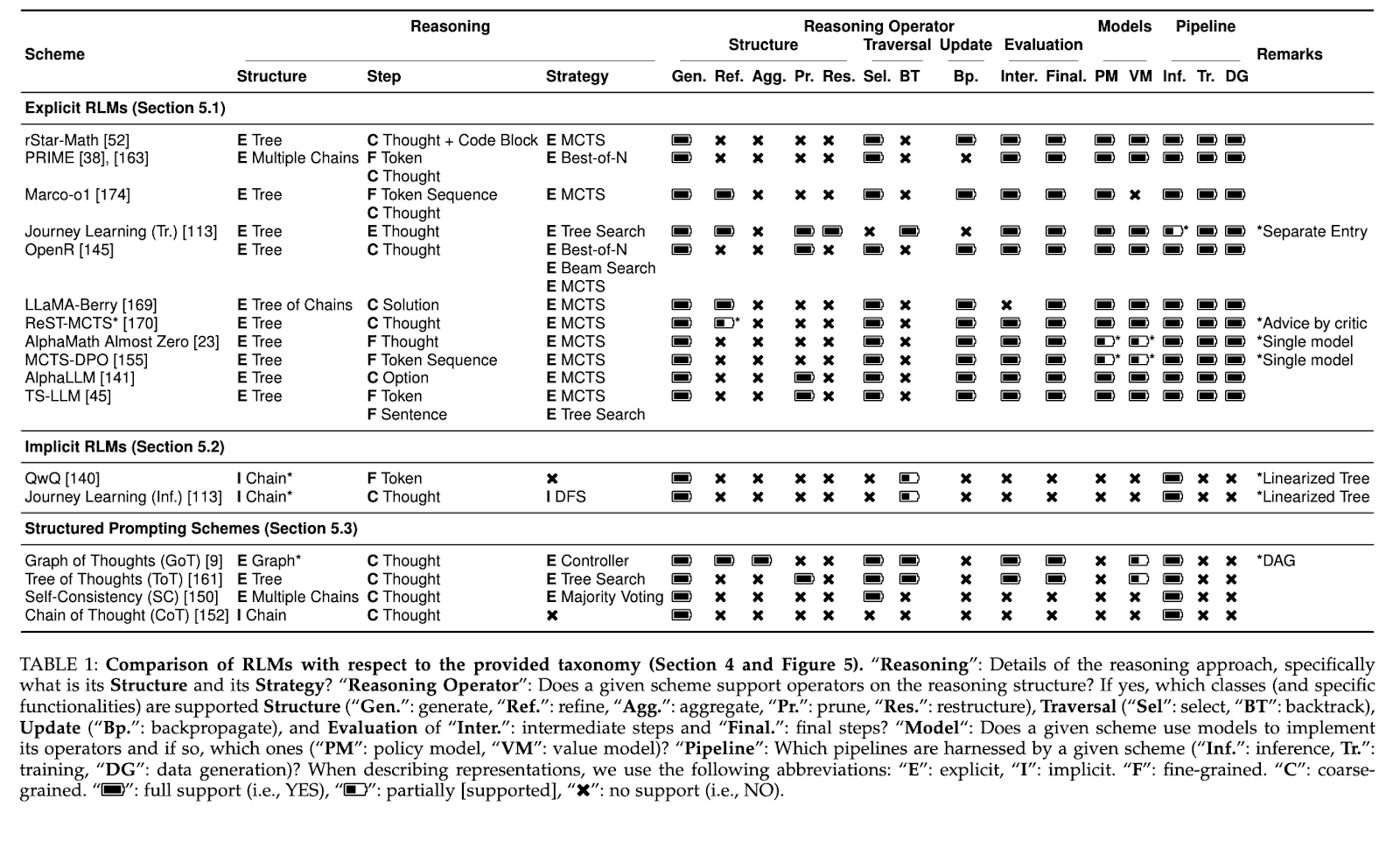
The Plan organizes the construction of RLM in a clear set of components: reasoning schemes, operators and pipes. The reasoning schemes define the structures and strategies to solve complex problems that range from sequential chains to hierarchical graphics of several levels. Operators control how these patterns change so that operations can include without problems adjustments, pruning and restructuring of reasoning routes. The pipes allow an easy flow between training, inference and data generation and are adaptable between applications. This block component structure admits individual access, while models can be adjusted to a detailed task, such as token reasoning or broader structured challenges.
The team demonstrated the effectiveness of the model and framework X1 through empirical studies and implementations in the real world. This modular design provided multi -phase training strategies that could optimize policies and values models, further improving the precision and scalability of reasoning. He took advantage of family training distributions to maintain high precision in all applications. The results worth mentioning included great improvements in efficiency in reasoning tasks attributed to the simplified integration of reasoning structures. For example, it demonstrated the potential of effective techniques for augmented recovery generation through experiments, reducing the computational cost of complex decision -making scenarios. These advances reveal that the plan allows democratizing advanced reasoning technologies even for low -income organizations.
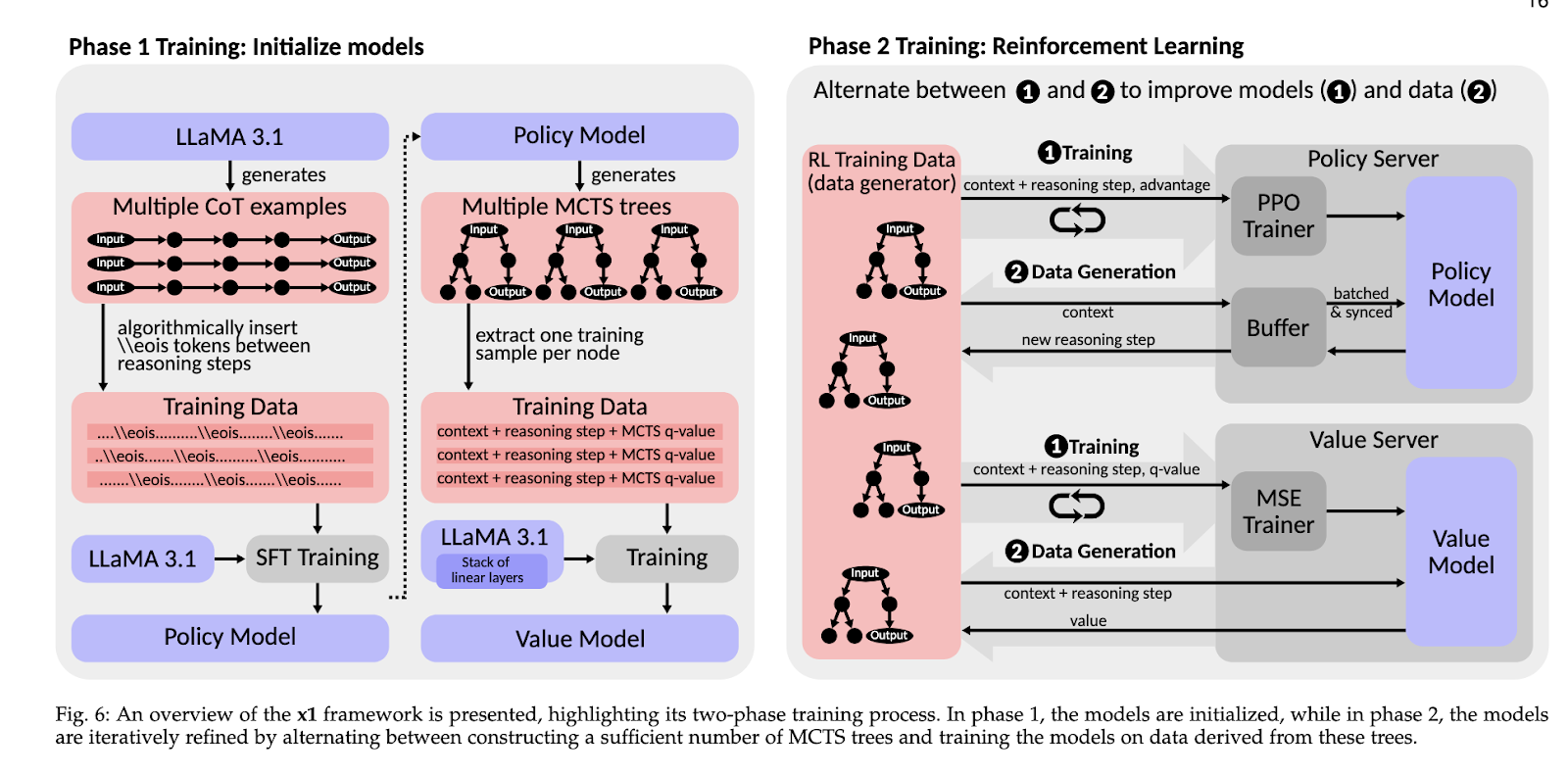
This work marks a turning point in the RLM design. This research addresses important issues in access and scalability to allow researchers and organizations to develop new reasoning paradigms. The modular design encourages experimentation and adaptation, helping to close the gap between ownership systems and open innovation. The introduction of Frame X1 underlines this effort even more by providing a practical tool to develop and implement scalable RLM. This work offers a road map for the advancement of intelligent systems, guaranteeing that the benefits of advanced reasoning models can be widely shared between industries and disciplines.
Verify he Paper. All credit for this investigation goes to the researchers of this project. Besides, do not forget to follow us in <a target="_blank" href="https://x.com/intent/follow?screen_name=marktechpost” target=”_blank” rel=”noreferrer noopener”>twitter and join our Telegrams channel and LINKEDIN GRabove. Do not forget to join our Subbreeddit of more than 70,000 ml.
<a target="_blank" href="https://nebius.com/blog/posts/studio-embeddings-vision-and-language-models?utm_medium=newsletter&utm_source=marktechpost&utm_campaign=embedding-post-ai-studio” target=”_blank” rel=”noreferrer noopener”> (Recommended Reading) Nebius ai Studio expands with vision models, new language, inlays and Lora models (Promoted)
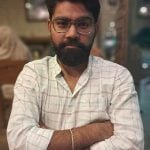
Nikhil is an internal consultant at Marktechpost. He is studying a double degree integrated into materials at the Indian Institute of Kharagpur technology. Nikhil is an IA/ML enthusiast who is always investigating applications in fields such as biomaterials and biomedical science. With a solid training in material science, it is exploring new advances and creating opportunities to contribute.