Music information retrieval (MIR) has become increasingly vital as the digitalization of music has skyrocketed. MIR involves the development of algorithms that can analyze and process musical data to recognize patterns, classify genres, and even generate new musical compositions. This multidisciplinary field combines elements of music theory, machine learning, and audio processing, with the goal of creating tools that can understand music in a way that is meaningful to humans and machines. Advances in MIR are paving the way for more sophisticated music recommendation systems, automated music transcription, and innovative applications in the music industry.
A major challenge facing the MIR community is the need for standardized benchmarks and evaluation protocols. This lack of consistency makes it difficult for researchers to compare the performance of different models on various tasks. The diversity of music itself further exacerbates the problem (it spans multiple genres, cultures, and forms), making it nearly impossible to create a universal evaluation system that applies to all types of music. Without a unified framework, progress in the field is slow as innovations cannot be reliably measured or compared, leading to a fragmented landscape where advances in one area may not translate well to others.
Currently, MIR tasks are evaluated using a variety of datasets and metrics, each tailored to specific tasks such as music transcription, chord estimation, and melody extraction. However, these tools and benchmarks are often limited in scope and do not allow for comprehensive evaluations of performance across different tasks. For example, chord estimation and melody extraction may use completely different datasets and evaluation metrics, making it difficult to measure the overall effectiveness of a model. Furthermore, the tools used are often designed for Western tonal music, leaving a gap in the evaluation of non-Western or folk music traditions. This fragmented approach has led to inconsistent results and a lack of clear direction in MIR research, hindering the development of more universal solutions.
To address these questions, researchers have introduced MARBLE, a new benchmark that aims to standardize the evaluation of audio representations of music across multiple hierarchical levels. Developed by researchers at Queen Mary University of London and Carnegie Mellon University, MARBLE seeks to provide a comprehensive framework for evaluating music understanding models. This benchmark covers a wide range of tasks, from high-level genre classification and emotion recognition to more detailed tasks such as pitch tracking, beat tracking, and melody extraction. By categorizing these tasks into different levels of complexity, MARBLE enables a more structured and consistent evaluation process, allowing researchers to more effectively compare models and identify areas that require further improvement.
MARBLE’s methodology ensures that models are evaluated comprehensively and fairly across different tasks. The benchmark includes tasks involving high-level descriptions, such as genre classification and music tagging, as well as more complex tasks such as pitch and rhythm tracking, melody extraction, and lyric transcription. Additionally, MARBLE incorporates performance-level tasks, such as ornament and technique detection, and acoustic-level tasks, including singer identification and instrument classification. This hierarchical approach addresses the diversity of musical tasks and promotes consistency in evaluation, allowing for more accurate comparison of models. The benchmark also includes a unified protocol that standardizes input and output formats for these tasks, further improving the reliability of evaluations. Additionally, MARBLE’s comprehensive approach considers factors such as robustness, security, and alignment with human preferences, ensuring that models are technically competent and applicable in real-world scenarios.
The evaluation using the MARBLE method highlighted the varied performance of the models across different tasks. The results indicated strong performance in genre classification and music tagging tasks, where the models showed consistent accuracy. However, the models faced challenges in more complex functions such as pitch tracking and melody extraction, revealing areas where further refinement is needed. The results underlined the effectiveness of the models in certain aspects of music understanding, while identifying gaps, particularly in handling diverse and non-Western musical contexts.
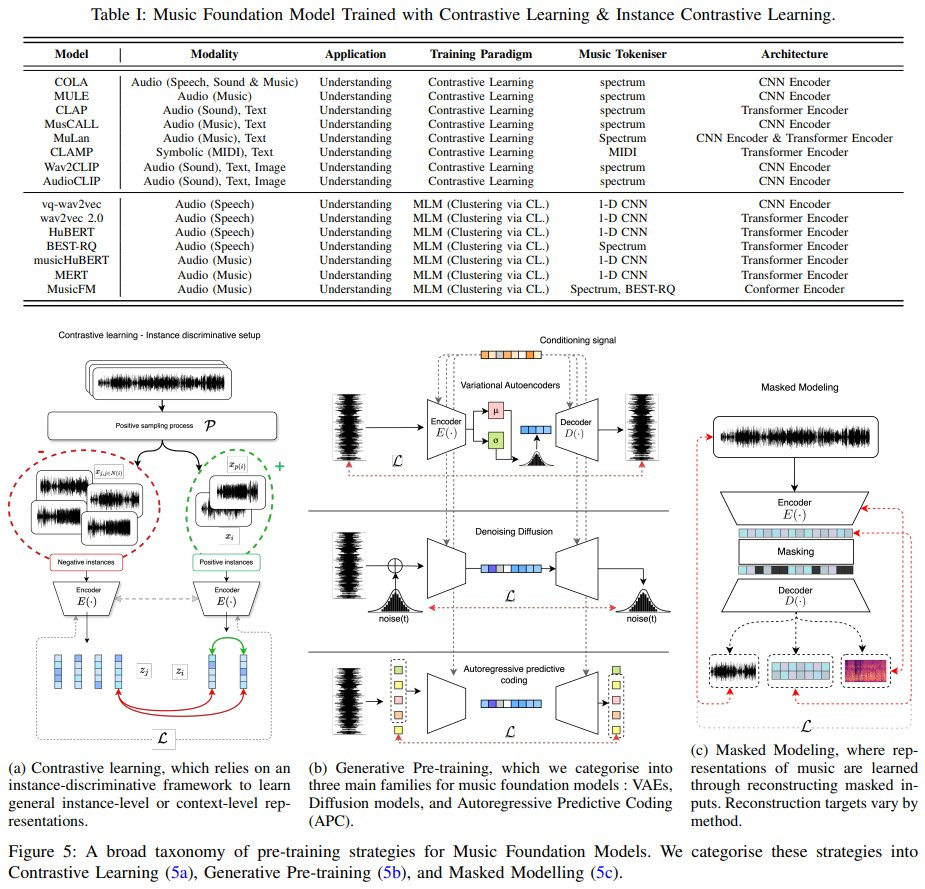
In conclusion, the introduction of the MARBLE benchmark represents a significant advancement in the field of music information retrieval. By providing a standardized and comprehensive evaluation framework, MARBLE addresses a critical gap in the field, allowing for more consistent and reliable comparisons of music understanding models. This benchmark not only highlights the areas in which current models excel, but also identifies the challenges that must be overcome to advance the state of music information retrieval. The work conducted by researchers at Queen Mary University of London and Carnegie Mellon University paves the way for more robust and universally applicable music analysis tools, ultimately contributing to the evolution of the music industry in the digital age.
Take a look at the Paper. All credit for this research goes to the researchers of this project. Also, don't forget to follow us on twitter.com/Marktechpost”>twitter and join our Telegram Channel and LinkedIn GrAbove!. If you like our work, you will love our fact sheet..
Don't forget to join our SubReddit of over 50,000 ml
Below is a highly recommended webinar from our sponsor: ai/webinar-nvidia-nims-and-haystack?utm_campaign=2409-campaign-nvidia-nims-and-haystack-&utm_source=marktechpost&utm_medium=banner-ad-desktop” target=”_blank” rel=”noreferrer noopener”>'Developing High-Performance ai Applications with NVIDIA NIM and Haystack'
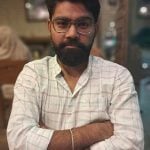
Nikhil is a Consultant Intern at Marktechpost. He is pursuing an integrated dual degree in Materials from Indian Institute of technology, Kharagpur. Nikhil is an ai and Machine Learning enthusiast who is always researching applications in fields like Biomaterials and Biomedical Science. With a strong background in Materials Science, he is exploring new advancements and creating opportunities to contribute.
<script async src="//platform.twitter.com/widgets.js” charset=”utf-8″>