Text-to-audio generation has transformed the way audio content is created, automating processes that traditionally required significant expertise and time. This technology enables the conversion of textual cues into diverse and expressive audio, streamlining workflows in audio production and the creative industries. Combining text input with realistic audio outputs has opened up possibilities in applications such as multimedia storytelling, music, and sound design.
One of the important challenges in text-to-audio systems is ensuring that the generated audio closely aligns with the textual prompts. Current models often fail to capture intricate details, leading to complete inconsistencies. Some outputs omit essential elements or introduce unwanted audio artifacts. The lack of standardized methods to optimize these systems further exacerbates the problem. Unlike language models, text-to-audio systems do not benefit from strong alignment strategies such as reinforcement learning with human feedback, leaving much room for improvement.
Previous approaches to text-to-audio generation relied heavily on broadcast-based models, such as AudioLDM and Stable Audio Open. While these models offer decent quality, they have limitations. Their reliance on extensive denoising steps makes them computationally expensive and time-consuming. Additionally, many models are trained on proprietary data sets, which limits their accessibility and reproducibility. These limitations hinder its scalability and ability to handle diverse and complex requests effectively.
To address these challenges, researchers from Singapore University of technology and Design (SUTD) and NVIDIA introduced TANGOFLUX, an advanced text-to-audio generation model. This model is designed to provide efficiency and high-quality results, achieving significant improvements over previous methods. TANGOFLUX uses the CLAP Classified Preference Optimization (CRPO) framework to iteratively refine audio generation and ensure alignment with textual descriptions. Its compact architecture and innovative training strategies allow it to perform exceptionally well and require fewer parameters.
TANGOFLUX integrates advanced methodologies to achieve cutting-edge results. It employs a hybrid architecture that combines diffusion transformer (DiT) and multimode diffusion transformer (MMDiT) blocks, allowing it to handle variable-length audio generation. Unlike traditional diffusion-based models, which rely on multiple denoising steps, TANGOFLUX uses a flow adaptation framework to create a direct, rectified path from noise to output. This rectified stream approach reduces the computational steps required for high-quality audio generation. During training, the system incorporates textual and duration conditioning to ensure accuracy in capturing the nuances of input cues and the desired duration of audio output. The CLAP model evaluates alignment between audio and textual cues by generating preference pairs and optimizing them iteratively, a process inspired by alignment techniques used in language models.
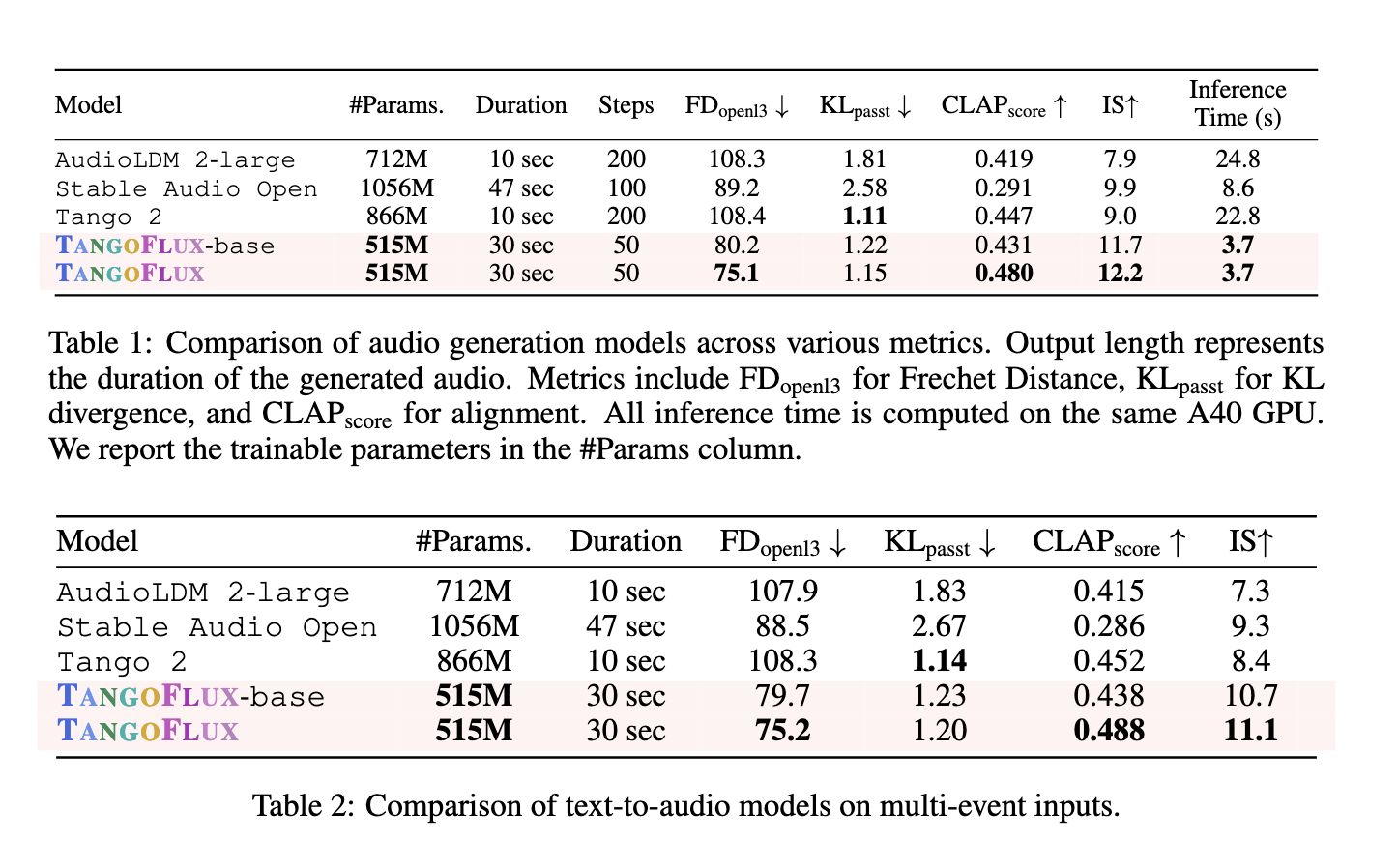
In terms of performance, TANGOFLUX outshines its predecessors in multiple metrics. It generates 30 seconds of audio in just 3.7 seconds using a single A40 GPU, demonstrating exceptional efficiency. The model achieves a CLAP score of 0.48 and an FD score of 75.1, both indicative of high-quality, text-aligned audio outputs. Compared to Stable Audio Open, which achieves a CLAP score of 0.29, TANGOFLUX significantly improves alignment accuracy. In multi-event scenarios, where cues include multiple distinct events, TANGOFLUX excels, showcasing its ability to capture intricate details and temporal relationships effectively. The system's robustness is further highlighted by its ability to maintain performance even at low sampling steps, a feature that enhances its practicality in real-time applications.
Human evaluations corroborate these results, with TANGOFLUX scoring highest in subjective metrics such as overall quality and immediate relevance. Annotators consistently rated their results as clearer and more aligned than other models such as AudioLDM and Tango 2. The researchers also emphasized the importance of the CRPO framework, which allowed the creation of a preference dataset that outperformed alternatives such as BATON and Audio-Alpaca. The model avoided performance degradation typically associated with offline data sets by generating new synthetic data during each training iteration.
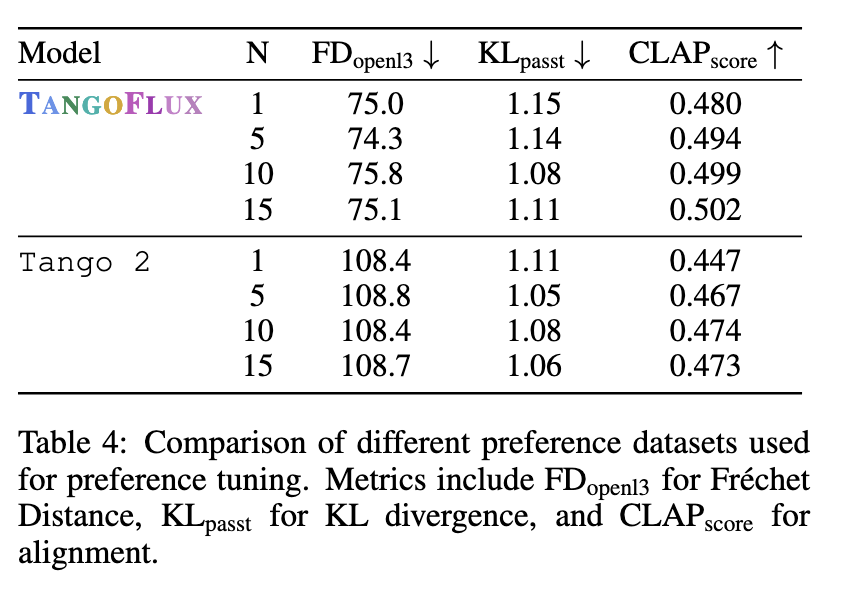
The research successfully addresses the critical limitations of text-to-audio systems by introducing TANGOFLUX, which combines efficiency with superior performance. Its innovative use of rectified flow and preference optimization sets a benchmark for future advances in this field. This development improves the quality and alignment of the generated audio and demonstrates scalability, making it a practical solution for widespread adoption. The work of SUTD and NVIDIA represents a major advancement in text-to-audio technology, pushing the boundaries of what can be achieved in this rapidly evolving domain.
Verify he Paper, Code repositoryand Pretrained model. All credit for this research goes to the researchers of this project. Also, don't forget to follow us on <a target="_blank" href="https://twitter.com/Marktechpost”>twitter and join our Telegram channel and LinkedIn Grabove. Don't forget to join our SubReddit over 60,000 ml.
UPCOMING FREE ai WEBINAR (JANUARY 15, 2025): <a target="_blank" href="https://info.gretel.ai/boost-llm-accuracy-with-sd-and-evaluation-intelligence?utm_source=marktechpost&utm_medium=newsletter&utm_campaign=202501_gretel_galileo_webinar”>Increase LLM Accuracy with Synthetic Data and Assessment Intelligence–<a target="_blank" href="https://info.gretel.ai/boost-llm-accuracy-with-sd-and-evaluation-intelligence?utm_source=marktechpost&utm_medium=newsletter&utm_campaign=202501_gretel_galileo_webinar”>Join this webinar to learn practical information to improve LLM model performance and accuracy while protecting data privacy..
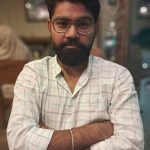
Nikhil is an internal consultant at Marktechpost. He is pursuing an integrated double degree in Materials at the Indian Institute of technology Kharagpur. Nikhil is an ai/ML enthusiast who is always researching applications in fields like biomaterials and biomedical science. With a strong background in materials science, he is exploring new advances and creating opportunities to contribute.
<script async src="//platform.twitter.com/widgets.js” charset=”utf-8″>