Large language models (LLMs) have attracted significant attention for their ability to understand and generate human-like text. These models have the unique ability to encode factual knowledge effectively, thanks to the large amount of data they are trained on. This capability is crucial in various applications, ranging from natural language processing (NLP) tasks to more advanced forms of artificial intelligence. However, understanding how these models acquire and retain objective information during pre-training is a complex challenge. This research investigates the intricate process through which LLMs internalize knowledge and explores how these models can be optimized to maintain and generalize the knowledge they acquire.
One of the main problems that researchers face in LLM training is the loss of factual knowledge over time. When large data sets are used in pre-training, LLMs struggle to retain details of specific facts, especially when new information is introduced in later stages of training. Additionally, LLMs often have difficulty remembering uncommon or long-tail knowledge, which significantly impacts their ability to generalize across various topics. This retention loss affects the accuracy of models when applied to complex or infrequently encountered scenarios, presenting a considerable barrier to improving the performance of LLMs.
Various methods have been introduced to address these challenges, focusing on improving the acquisition and retention of factual knowledge in LLMs. These methods include scaling model sizes and pre-training data sets, using advanced optimization techniques, and modifying batch sizes to better handle data during training. Deduplication of datasets has also been proposed to reduce redundancy in training data, leading to more efficient learning. Despite these efforts, the fundamental problems of rapid forgetting and the model's difficulty in generalizing less frequent events remain, and current solutions have achieved only incremental improvements.
Researchers from KAIST, UCL and KT have introduced a novel approach to studying the acquisition and retention of factual knowledge in LLMs. They designed an experiment that systematically injected new factual knowledge into the model during pre-training. By analyzing the model's ability to memorize and generalize this knowledge under various conditions, the researchers aimed to uncover the dynamics that govern how LLMs learn and forget. Their approach involved monitoring model performance across different checkpoints and observing the effect of factors such as batch size, data duplication, and paraphrasing on knowledge retention. This experiment provided valuable insights into optimizing training strategies to improve long-term memory in LLMs.
The researchers' methodology was exhaustive and involved detailed evaluation at multiple stages of pre-training. They conducted the experiments using fictitious knowledge that the model had not encountered before to ensure the accuracy of the analysis. Various conditions were tested, including injecting the same factual knowledge repeatedly, paraphrasing it, or presenting it only once. To measure the effectiveness of knowledge retention, the team evaluated the model's performance by examining changes in the probability of remembering specific facts over time. They found that larger batches helped the model maintain factual knowledge more effectively, while duplicate data led to faster forgetting. By using a variety of testing conditions, the research team was able to determine the most effective strategies for training LLMs to retain and generalize knowledge.
The performance of the proposed methodology revealed several key findings. First, the research showed that larger models, such as those with 7 billion parameters, exhibited better retention of factual knowledge than smaller models with only 1 billion parameters. Interestingly, the amount of training data used did not significantly affect retention, contradicting the belief that more data leads to better model performance. Instead, the researchers found that models trained on a deduplicated data set were more robust and had slower forgetting rates. For example, models exposed to paraphrased knowledge showed a higher degree of generalization, meaning they could apply the knowledge more flexibly in different contexts.
Another key finding was the relationship between batch size and knowledge retention. Models trained with larger batches, such as 2048, demonstrated greater resistance to forgetting than those trained with smaller batches of 128. The study also discovered a power-law relationship between training steps and forgetting, showing that Factual knowledge degrades more rapidly in models. trained with duplicate data. On the other hand, models exposed to a higher volume of unique facts retained this knowledge longer, underscoring the importance of data set quality over mere quantity. For example, the decay constant for the duplicated data in the last pre-training stage was 0.21, compared to 0.16 for the paraphrased data, indicating slower forgetting when the data set was deduplicated.
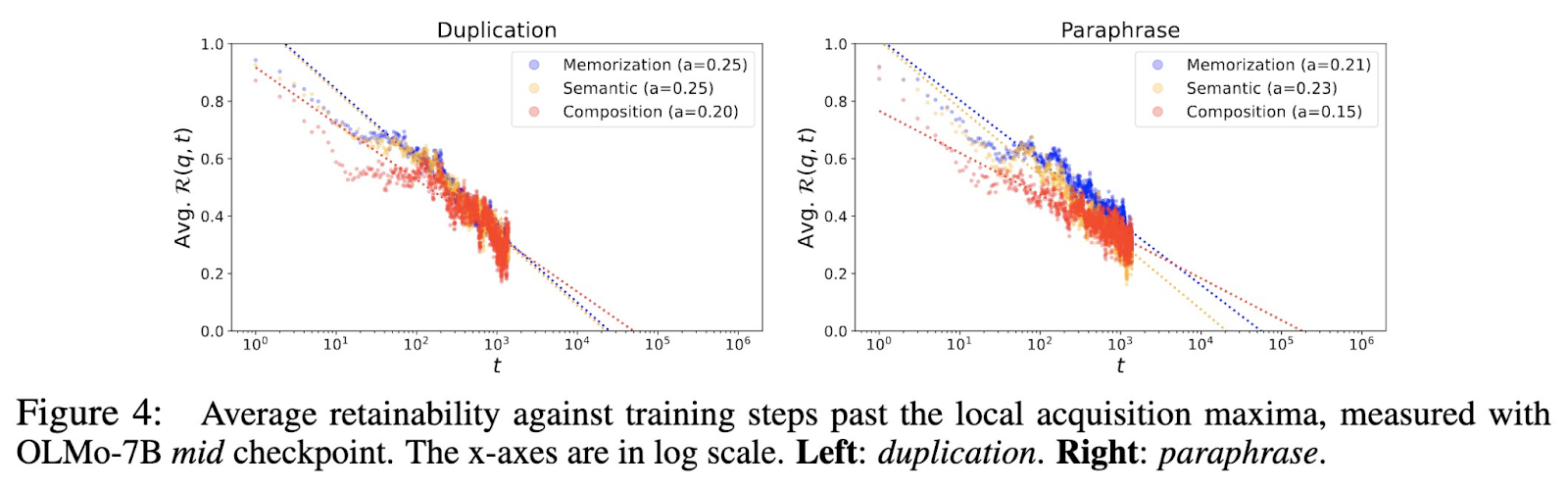
The research offers a promising approach to address the problems of forgetting and poor generalization in LLMs. The findings suggest that optimizing batch size and deduplication during the pre-training phase can significantly improve retention of factual knowledge in LLMs. These improvements can make models more reliable across a broader range of tasks, especially when dealing with less common or long-tail knowledge. Ultimately, this study provides a clearer understanding of the mechanisms behind knowledge acquisition in LLMs, opening new avenues for future research to refine training methods and further enhance the capabilities of these powerful models.
This research has provided valuable insights into how large language models acquire and retain knowledge. By identifying factors such as model size, batch size, and data set quality, the study offers practical solutions to improve LLM performance. These findings highlight the importance of efficient training techniques and underline the potential of optimizing LLMs to make them even more effective in handling complex and diverse linguistic tasks.
look at the Paper. All credit for this research goes to the researchers of this project. Also, don't forget to follow us on twitter.com/Marktechpost”>twitter and join our Telegram channel and LinkedIn Grabove. Don't forget to join our SubReddit over 50,000ml
Subscribe to the fastest growing ML newsletter with over 26,000 subscribers.

Nikhil is an internal consultant at Marktechpost. He is pursuing an integrated double degree in Materials at the Indian Institute of technology Kharagpur. Nikhil is an ai/ML enthusiast who is always researching applications in fields like biomaterials and biomedical science. With a strong background in materials science, he is exploring new advances and creating opportunities to contribute.
<script async src="//platform.twitter.com/widgets.js” charset=”utf-8″>