In recent years there have been drastic changes in the field of imaging, mainly due to the development of latent-based generative models, such as Latent diffusion models (LDM) and Mask Image Models (MIM). Reconstructive autoencoders, such as VQGAN and FEETcan reduce images to smaller, simpler shapes called low-dimensional latent space. This allows these models to create very realistic images. Considering the great influence of autoregressives (Arkansas) generative models, such as large language models in natural language processing (NLP), it is interesting to explore whether similar approaches can work with images. Although autoregressive models use the same latent space as models like LDM and MIM, they still fail somewhere in generating images. This contrasts sharply with natural language processing (NLP), where the GPT autoregressive model has achieved significant dominance.
current methods such as LDM and MIM use reconstructive autoencoders, such as VQGAN and FEETto transform images into a latent space. However, these approaches also face stability and performance challenges. It is seen that, in the VQGAN model, as the image reconstruction quality improves (indicated by a lower FID score), the overall quality of the generation may actually decrease. To address these problems, researchers have proposed a new method called Discriminative Generative Image Transformer (DiGIT). Unlike traditional autoencoder approaches, DiGIT separates training of encoders and decoders, starting with training only the encoder through a discriminative self-supervised model.
A team of researchers from the School of Data Science and the School of Computer Science and technology of the University of Science and technology of China, as well as the State Key Laboratory of Cognitive Intelligence and Zhejiang University propose Discriminative Generative Image Transformer (DiGIT). This method separates the training of encoders and decoders, starting with the encoder and training through a discriminative self-supervised model. This strategy improves the stability of the latent space, making it more robust for autoregressive modeling. They use a VQGAN-inspired method to convert the encoder's latent feature space into discrete tokens using K-means clustering. Research suggests that image autoregressive models can perform similarly to GPT models in natural language processing. The main contributions of this work include a unified perspective on the relationship between latent space and generative models, emphasizing the importance of stable latent spaces; a novel method that separates the training of encoders and decoders to stabilize the latent space; and an efficient discrete image tokenizer that improves the performance of image autoregressive models.
During testing, the researchers compared each image patch to the closest token in the codebook. After training a Causal Transformer to predict the next token using these tokens, the researchers obtained good results on ImageNet. The DiGIT model outperforms previous techniques in image understanding and generation, demonstrating that using a smaller token grid can lead to higher accuracy. Experiments conducted by researchers highlighted the effectiveness of the proposed discriminative tokenizer, which significantly increases the performance of the model as the number of parameters increases. The study also found that increasing the number of K-Means clusters improves accuracy, reinforcing the advantages of a larger vocabulary in autoregressive modeling.
In conclusion, this article presents a unified view of how latent space and generative models are related, highlighting the importance of a stable latent space in image generation and introducing a simple but effective image tokenizer and an autoregressive generative model called Digit. The results also challenge the common belief that being good at reconstruction also means having an effective latent space for autoregressive generation. Through this work, researchers aim to revive interest in generative pre-training of autoregressive image models, encourage a re-evaluation of the fundamental components that define the latent space for generative models, and make this a step towards new technologies and methods.
look at the Paper and GitHub. All credit for this research goes to the researchers of this project. Also, don't forget to follow us on twitter.com/Marktechpost”>twitter and join our Telegram channel and LinkedIn Grabove. If you like our work, you will love our information sheet.. Don't forget to join our SubReddit over 55,000ml.
(Next live webinar: October 29, 2024) Best platform to deliver optimized models: Predibase inference engine (promoted)
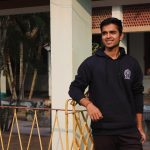
Divyesh is a Consulting Intern at Marktechpost. He is pursuing a BTech in Agricultural and Food Engineering from the Indian Institute of technology Kharagpur. He is a data science and machine learning enthusiast who wants to integrate these leading technologies in agriculture and solve challenges.
<script async src="//platform.twitter.com/widgets.js” charset=”utf-8″>