artificial intelligence (ai) is making significant advances in natural language processing (NLP), focusing on improving models that can accurately interpret and generate human language. Researchers are working to develop models that capture complex linguistic structures and generate coherent, contextually relevant responses across extended dialogues. Advances in this area are vital for applications such as automated customer service, content creation, and machine translation, where language accuracy and sustained consistency are critical. As demand for ai capabilities in these applications grows, it is increasingly essential to improve the models' ability to handle nuanced language and maintain context.
A major problem NLP faces is maintaining coherence in long texts. Linguistic models tend to lose sight of long-term dependencies within the text, resulting in inconsistencies and lack of context in responses. This limitation is particularly problematic in applications that require extensive interactive dialogue, as responses may need to be aligned with the preceding context. Solving this problem is crucial to advancing ai applications that rely on natural language understanding and generation for effective and reliable performance.
Current language models, predominantly based on transformative architectures such as GPT and BERT, have made substantial progress, but are often limited by high computational demands and a restricted ability to maintain context in extended text. These transformers process text in a way that requires a significant amount of memory and processing power, making their application impractical in environments with limited computational resources. Additionally, transformative models sometimes need help with the coherence of long texts, which limits their effectiveness on complex linguistic tasks. Therefore, researchers are exploring ways to balance performance with computational efficiency.
Researchers from amazon and Michigan State University introduced a new model to address these challenges by refining the transformer architecture. This model aims to reduce computational load while preserving coherence across long text segments, employing a novel segmentation approach to maintain the accuracy of contextually relevant responses. By introducing error-aware reasoning by segmenting text into smaller units, the model can process long passages without compromising coherence, which is a considerable advance in the field of NLP. This segmentation also allows for scalable modular adjustments, making the model versatile for linguistic tasks including question answering and conversational ai.
This model incorporates an error-aware demonstration mechanism, allowing it to adjust predictions based on inaccuracies detected in intermediate reasoning steps. Instead of processing text in one large unit, this model divides input into smaller segments that maintain contextual links, allowing for coherent processing across long passages. The modular design further allows researchers to tune specific model parameters to meet the needs of different applications without requiring a complete system redesign. This scalability positions the model as a flexible and efficient solution for various NLP applications.
In experiments, this model demonstrated marked improvements across several benchmarks. For example, on the “Random Object Tracking” dataset, the model accuracy increased from 56.53% to 61.20%, while on the “Penguins on a Table” dataset, performance improved from 81%. .34% to 82.19%. These results underline the improved ability of the model to handle complex reasoning tasks. The model also showed significant performance improvements on specific benchmarks; Accuracy improved by more than 2% in some cases, showing that it can consistently outperform standard transformers by accurately managing intermediate reasoning steps.
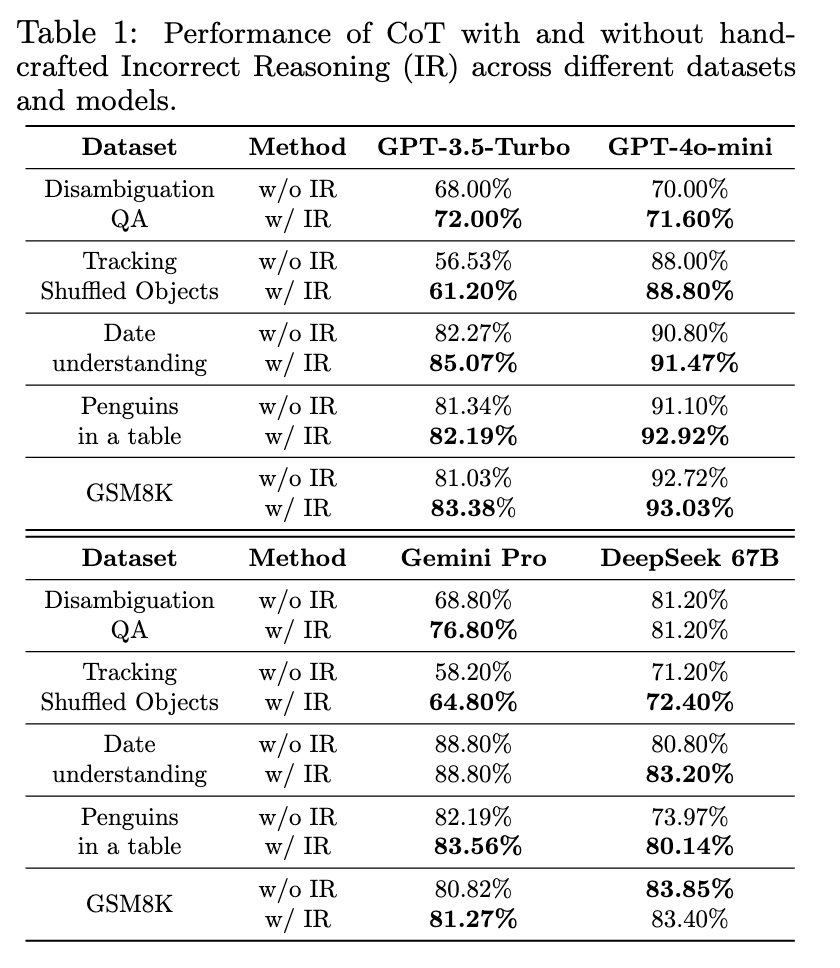
The study further highlights how the model reduces computational costs while maintaining consistency. For example, accuracy improved by approximately 2% in specific scenarios when applying error-aware reasoning to multi-step tasks. The research found that incorporating correct and incorrect reasoning paths improved the model's ability to detect and correct reasoning errors, which is particularly beneficial in complex dialogues or extended reasoning scenarios. These findings suggest that the model's robust architecture could make it an ideal choice for applications that require accurate and sustained language understanding during prolonged interactions.
Overall, this research by amazon and Michigan State University presents a notable advancement in NLP by addressing critical challenges in maintaining consistency and reducing computational stress. The proposed model balances accuracy with efficiency and promises substantial benefits for various linguistic applications. Its modular and adaptable structure positions it as a versatile tool for real-world ai tasks that demand accurate and contextually aware language processing in diverse fields.
look at the Paper. All credit for this research goes to the researchers of this project. Also, don't forget to follow us on twitter.com/Marktechpost”>twitter and join our Telegram channel and LinkedIn Grabove. If you like our work, you will love our information sheet.. Don't forget to join our SubReddit over 55,000ml.
(Next live webinar: October 29, 2024) Best platform to deliver optimized models: Predibase inference engine (promoted)
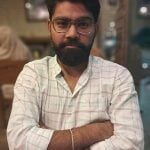
Nikhil is an internal consultant at Marktechpost. He is pursuing an integrated double degree in Materials at the Indian Institute of technology Kharagpur. Nikhil is an ai/ML enthusiast who is always researching applications in fields like biomaterials and biomedical science. With a strong background in materials science, he is exploring new advances and creating opportunities to contribute.
<script async src="//platform.twitter.com/widgets.js” charset=”utf-8″>