This research delves into a formidable challenge within the domain of neural autoregressive operators: the limited ability to extend the forecast horizon. Autoregressive models, although promising, face instability problems that significantly impede their effectiveness in spatiotemporal forecasting. This general problem is ubiquitous, encompassing scenarios ranging from relatively fluid fields to complex, large-scale systems typified by data sets such as ERA5.
Current methods face formidable obstacles when attempting to expand the forecast horizon for neural autoregressive operators. Recognizing these limitations, the research team presents a revolutionary solution to improve predictability. The proposed method initiates a fundamental architectural change in spectral neural operators, a strategic move to mitigate instability issues. In stark contrast to existing methodologies, this innovative approach gives these operators an indefinite forecast horizon, marking a significant advance.
Currently, autoregressive neural operators reveal a major hurdle in their ability to forecast beyond a limited horizon. The instability challenges of traditional methods restrict their effectiveness, particularly in complex spatiotemporal forecasting scenarios. To address this, the research team proposes a novel solution that fundamentally reshapes the architecture of spectral neural operators, unlocking the potential for an extended forecast horizon.
The core of the proposed method is the restructuring of the neural operator block. To address challenges such as aliasing and discontinuity, the researchers introduce a novel framework in which nonlinearities are consistently replaced by learnable filters capable of effectively handling the newly generated high frequencies. A key innovation is the introduction of dynamic filters, which replace static convolutional filters and adapt to the specific data being considered. This adaptability is achieved through a multilayer perceptron (MLP) that operates in the frequency domain.
The essence of the proposed method lies in reinventing the neural operator block. Addressing challenges such as aliasing and discontinuity, the researchers introduce a sophisticated framework where nonlinearities are constantly tracked by learnable filters adept at handling newly generated high frequencies. An innovative element is the incorporation of dynamic filters, which replace conventional static convolutional filters and adapt to the complexities of the specific data set. This adaptability is achieved through a multilayer perceptron (MLP) that operates in the frequency domain.
The experimental results underline the effectiveness of the method and reveal significant improvements in stability. This is particularly evident when applying the approach to scenarios such as the shallow water spinning equations and the ERA5 data set. Dynamic filters, generated through frequency adaptive MLP, are essential to guarantee the adaptability of the model to various data sets. By replacing static filters with dynamic counterparts, the method deftly handles the complexities of data-dependent aliasing patterns, an achievement unattainable using fixed strategies.
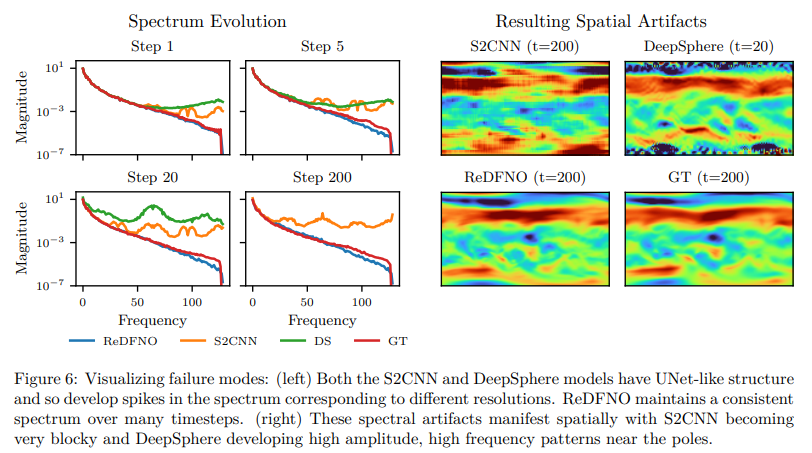
In conclusion, the research represents an innovative step to overcome the persistent challenge of expanding the forecast horizon in autoregressive neural operators. The restructuring of the neural operator block, characterized by incorporating dynamic filters generated through a frequency adaptive MLP, is a very effective strategy to mitigate instability problems and allow an indefinite forecast horizon. As the research community grapples with the complexities of prediction, this work serves as a beacon guiding future efforts toward more robust and reliable spatiotemporal prediction models.
Review the Paper and GitHub. All credit for this research goes to the researchers of this project. Also, don't forget to join. our 33k+ ML SubReddit, 41k+ Facebook community, Discord channel, and Electronic newsletterwhere we share the latest news on ai research, interesting ai projects and more.
If you like our work, you'll love our newsletter.
Madhur Garg is a consulting intern at MarktechPost. He is currently pursuing his Bachelor's degree in Civil and Environmental Engineering from the Indian Institute of technology (IIT), Patna. He shares a great passion for machine learning and enjoys exploring the latest advancements in technologies and their practical applications. With a keen interest in artificial intelligence and its various applications, Madhur is determined to contribute to the field of data science and harness the potential impact of it in various industries.
<!– ai CONTENT END 2 –>