Going by the advances made by ai in drug discovery, it can be said that there is a lot of untapped potential. Therapeutic nanobodies, in particular, have seen relatively limited progress as they require complex interdisciplinary expertise. The COVID-19 pandemic prompted the development of therapeutic nanobodies that exhibit high binding affinity and stability for SARS-CoV-2 in a short period. However, developing and testing a new drug requires many resources and a lot of time. Researchers from Stanford University's Department of Computer Science and Biomedical Data Sciences and Chan Zuckerberg Biohub in San Francisco have used a remarkable framework, Virtual Lab, that has helped streamline the drug development process from design to production. evidence.
Conventional methods involve experimental screening of large libraries of nanobody candidates against the target antigen to identify high-affinity binders. However, it requires a lot of time, resources and manpower. Computational methods have also been developed to identify nanobody candidates, but have been found to lack precision, which could be very detrimental if used as a therapeutic. Given the rapid mutation rates of the SARS-CoV-2 virus, it is imperative that a substantial number of lives are lost while drugs are in development. These limitations have put pressure on the health system.
The proposed method employs a virtual laboratory environment where ai agents with different areas of expertise collaborate and address the problem, mimicking real-world scientific teamwork. A computational process is developed after holding meetings between the ai agents. Key components of this pipeline include:
- ESM (Evolutionary Scale Modeling): Analyzes protein sequences and observes the effects of various mutations on protein function and stability. This tool is essential to find potential mutations that improve the binding of the nanobody to the spike proteins of our virus.
- AlphaFold-Multimer: To predict the protein-protein interaction between the virus and the nanobody, AplhaFold-Multimer uses deep learning and generates high-confidence structural predictions.
- Rosetta: Uses the iterative refinement process to optimize the three-dimensional structures of the designed nanobodies.
Experimental validation showed that more than 90% of the designed nanobodies were expressed and soluble, and two candidates showed superior binding properties specifically against the new SARS-CoV-2 variants JN.1 and KP.3, while retaining strong interactions with the ancestral peak. protein. This is an essential result to demonstrate the effectiveness of the Virtual Laboratory computational framework in rapidly generating viable therapeutic candidates.
In conclusion, this article describes ai-based nanobodies produced with their incorporation into existing experimental methodologies. This synergistic framework of multiple artificial agents greatly elevates the design and validation stages of many established methods, which tend to be time- and resource-consuming. Optimal identification of nanobodies targeting SARS-CoV-2 variants provides essential evidence that ai can be instrumental in accelerating therapeutic discoveries. This novel approach improves efficiency in nanobody design and facilitates rapid response to emerging viral threats. This gives you a perspective that describes the tremendous effect of artificial intelligence on biomedical research and its applications in the development of therapies.
Verify the paper. All credit for this research goes to the researchers of this project. Also, don't forget to follow us on <a target="_blank" href="https://twitter.com/Marktechpost”>twitter and join our Telegram channel and LinkedIn Grabove. If you like our work, you will love our information sheet.. Don't forget to join our 59k+ ML SubReddit.
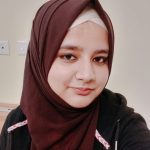
Afeerah Naseem is a Consulting Intern at Marktechpost. He is pursuing his bachelor's degree in technology from the Indian Institute of technology (IIT), Kharagpur. He is passionate about data science and fascinated by the role of artificial intelligence in solving real-world problems. He loves discovering new technologies and exploring how they can make everyday tasks easier and more efficient.
<script async src="//platform.twitter.com/widgets.js” charset=”utf-8″>