The last two years have been filled with eureka moments for various disciplines. We have witnessed the emergence of revolutionary methods that have resulted in colossal advances. It was ChatGPT for language models, stable diffusion for generative models, and neural radiation fields (NeRF) for graphics and computer vision.
NeRF has emerged as a groundbreaking technique, revolutionizing the way we represent and render 3D scenes. NeRF represents a scene as a continuous 3D volume, which encodes geometry and appearance information. Unlike traditional explicit renderings, NeRF captures scene properties via a neural network, enabling novel view synthesis and accurate reconstruction of complex scenes. By modeling the volumetric density and color of each point in the scene, NeRF achieves impressive photorealism and fidelity of detail.
The versatility and potential of NeRF have sparked extensive research efforts to improve its capabilities and address its limitations. Techniques have been proposed to speed up NeRF inference, handle dynamic scenes, and allow scene editing, further expanding the applicability and impact of this novel representation.
Despite all these efforts, NeRFs still have limitations that prevent their adaptability in practical scenarios. NeRF scene editing is one of the most important examples here. This is challenging due to the implicit nature of NeRFs and the lack of explicit separation between the different components of the scene.
Unlike other methods that provide explicit representations such as meshes, NeRFs do not provide a clear distinction between shape, color, and material. Also, combining new objects into NeRF scenes requires consistency across multiple views, further complicating the editing process.
The ability to capture scenes in 3D is only part of the equation. Being able to edit the output is equally important. Digital images and videos are powerful because we can edit them with relative ease, especially with the recent text-to-X AI models that allow effortless editing. So how could we bring that power to the scenes of NeRF? time to meet with Mixed-NeRF.
Mixed-NeRF is an approach for ROI-based editing of NeRF scenes guided by text messages or image patches. It allows you to edit any region of a real-world scene while preserving the rest of the scene without the need for new feature spaces or two-dimensional mask sets.
The goal is to produce eye-consistent, natural-looking results that blend seamlessly with the existing scene. More important, Mixed-NeRF it is not restricted to a specific class or domain and allows for complex text-guided manipulations such as object insertion/replacement, object blending, and texture conversion.
Achieving all these characteristics is not easy. That’s why Mixed-NeRF Leverages a pre-trained language image model, such as CLIP, and a NeRF model initialized in an existing NeRF scene as a generator to synthesize and combine new objects in the scene’s region of interest (ROI).
The CLIP model guides the rendering process based on user-provided text cues or image patches, enabling the generation of various 3D objects that blend naturally with the scene. To enable general local edits while preserving the rest of the scene, the user is presented with a simple GUI to locate a 3D frame within the NeRF scene, using depth information for intuitive feedback. For a perfect match, a new distance smoothing operation is proposed, merging the original and synthesized radiation fields by combining the sampled 3D points along each camera beam.
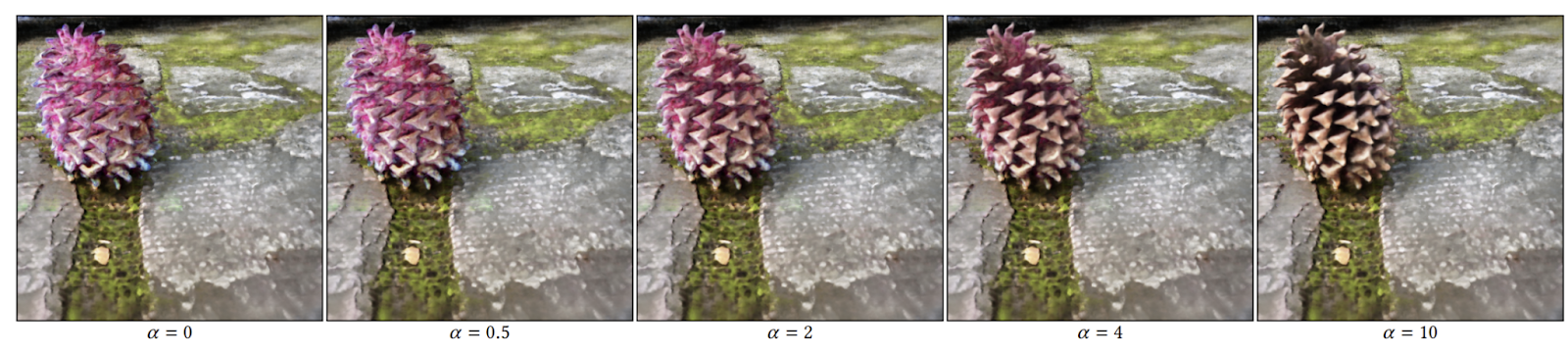
However, there was one more problem. Using this pipeline to edit NeRF scenes produces low-quality, inconsistent, and inconsistent results. To address this, the researchers behind Mixed-NeRF incorporate augmentations and enhancements suggested in previous work, such as depth regularization, pose sampling, and direction-dependent cues, to achieve more realistic and consistent results.
review the Paper and Project. Don’t forget to join our 26k+ ML SubReddit, discord channel, and electronic newsletter, where we share the latest AI research news, exciting AI projects, and more. If you have any questions about the article above or if we missed anything, feel free to email us at [email protected]
🚀 Check out 100 AI tools at AI Tools Club
Ekrem Çetinkaya received his B.Sc. in 2018, and M.Sc. in 2019 from Ozyegin University, Istanbul, Türkiye. She wrote her M.Sc. thesis on denoising images using deep convolutional networks. She received her Ph.D. He graduated in 2023 from the University of Klagenfurt, Austria, with his dissertation titled “Video Coding Improvements for HTTP Adaptive Streaming Using Machine Learning.” His research interests include deep learning, computer vision, video encoding, and multimedia networking.