Video has become ubiquitous, from streaming our favorite movies and TV shows to participating in video conferences and calls. With the increasing use of smartphones and other capture devices, video quality has become more important. However, due to various factors such as low light, digital noise, or simply poor acquisition quality, the quality of the videos captured by these devices is often far from perfect. In these situations, video enhancement techniques come into play, with the aim of improving resolution and visual characteristics.
Over the years, various video enhancement techniques have been developed until the advent of complex machine learning algorithms to remove noise and improve image quality. One of the most promising video enhancement technologies is neural networks. They have recently emerged as a powerful video enhancement tool, enabling unprecedented levels of clarity and detail in videos.
Among the most exciting applications of neural networks in video enhancement are super resolution, which involves increasing the resolution of a video to provide a clearer, more detailed image, and denoising, which aims to convert blurry areas. in distinguished features. With the help of neural networks, these tasks have become a reality.
However, the complexity of these video enhancement tasks poses several challenges in real-time applications. For example, several recent techniques, such as diffusion models, involve multiple, resource-intensive steps to generate an image from pure noise. For diffusion models, only the denoising steps require a powerful GPU.
With this challenge in mind, a new neural network framework called ReBotNet has been developed. An overview of the proposed system is available in the following figure.
The network takes the frame it needs to improve and the previously predicted frame as input. The uniqueness of the method lies in its design, which employs MLP-based and convolutional blocks to avoid the high computational complexity associated with traditional attention mechanisms while maintaining good performance.
Authors tokenize input frames in two ways to allow the network to learn spatial and temporal features. Each set of tokens is passed through separate mixer layers to determine the dependencies between them. The enhanced frame is predicted using a simple decoder based on these tokens. The method also uses temporal redundancy in real-world video to improve efficiency and temporal consistency. To achieve this, a frame recursive training setup is used where the previous prediction is used as an additional input to the network, allowing the propagation of information to future frames.
This approach is more efficient than techniques that use a multi-frame stack as input. As for the quality achieved, some results are presented below and compared with state-of-the-art techniques.
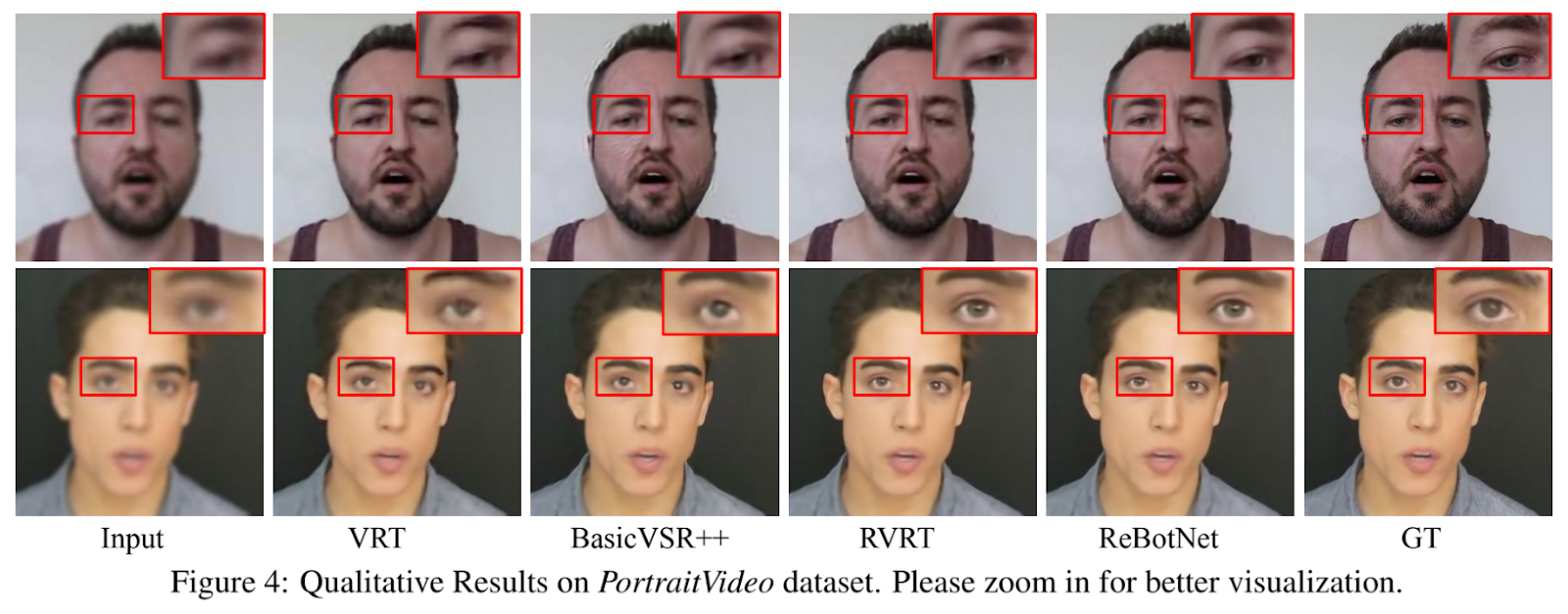
The authors claim that the proposed method is 2.5 times faster than previous state-of-the-art methods while equaling or slightly improving visual quality in terms of PSNR.
This was the brief for ReBotNet, a new AI framework for real-time video enhancement.
If you are interested or would like more information about this work, you can find a link to the document and the project page.
review the Paper and Project. All credit for this research goes to the researchers of this project. Also, don’t forget to join our 17k+ ML SubReddit, discord channeland electronic newsletterwhere we share the latest AI research news, exciting AI projects, and more.
Daniele Lorenzi received his M.Sc. in ICT for Internet and Multimedia Engineering in 2021 from the University of Padua, Italy. He is a Ph.D. candidate at the Institute of Information Technology (ITEC) at the Alpen-Adria-Universität (AAU) Klagenfurt. He currently works at the Christian Doppler ATHENA Laboratory and his research interests include adaptive video streaming, immersive media, machine learning and QoS / QoE evaluation.
🔥 Must Read: What is AI Hallucination? What goes wrong with AI chatbots? How to detect an amazing artificial intelligence?