In 3D reconstruction and generation, it is essential to look for techniques that balance visual richness with computational efficiency. Effective methods such as Gaussian Splatting often have significant limitations, particularly in handling high-frequency and sharp-edged signals due to their inherent low-pass characteristics. This limitation affects the quality of rendered scenes and imposes a substantial memory footprint, making it less ideal for real-time applications.
In the changing landscape of 3D reconstruction, a combination of classical and neural network methodologies transforms 2D images into detailed 3D structures. Neural Radiation Fields (NeRF) introduce a paradigm shift in creating photorealistic views from sparse inputs optimized for efficiency. Rendering improvements come from Gaussian Splatting, differentiable rasterization, and visual fidelity fine-tuning. Neural point-based representation along with NeRF enriches geometric and textural accuracy. Innovations such as zero-shot generators, DreamFusion, and Gauss-based methods accelerate the creation of 3D content, showcasing advancements in rendering technologies.
Researchers from the University of Oxford, KAUST, Columbia University and Snap Inc. have introduced Generalized Exponential Splatting (GES), which, by leveraging the generalized exponential function (GEF), offers more efficient rendering of 3D scenes, significantly reducing the number. of particles needed to model a scene accurately. This innovation improves the rendering of sharp edges and high-frequency signals and improves memory efficiency and rendering speed, marking a significant step forward in 3D scene modeling.
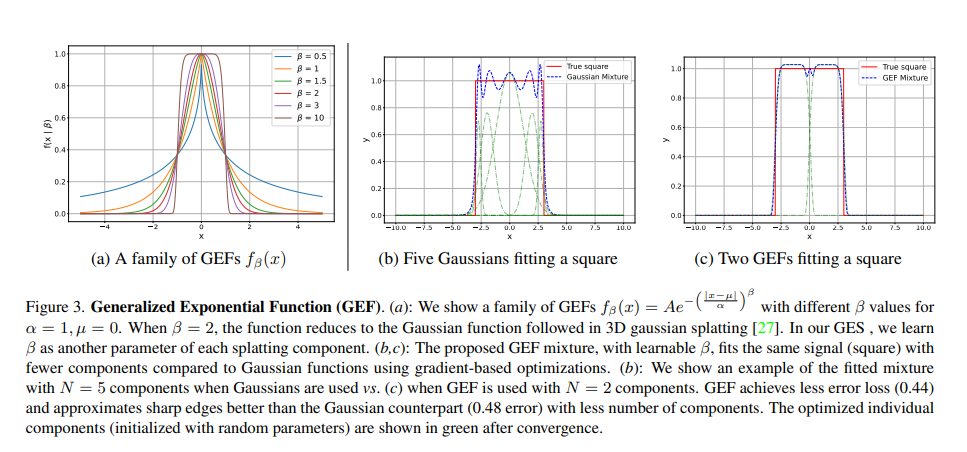
GES leverages GEF to redefine 3D scene modeling, significantly improving rendering efficiency and quality over Gaussian Splatting. By incorporating a shape parameter (β), GES precisely delineates scene edges, delivering superior memory utilization and performance on new view synthesis benchmarks. It employs a differentiable GES formulation, with sophisticated components such as spherical harmonics for color and a camera space covariance matrix (Σ′), refined using Structure from Motion (SfM) techniques. Advanced rendering is achieved using a fast differentiable rasterizer, which integrates along-ray radiation with β-based modifications and is optimized with a frequency-modulated image loss (Lω). This methodological advance presents a plug-and-play alternative to Gaussian Splatting, which guarantees efficient and high-quality rendering in various 3D scenes.
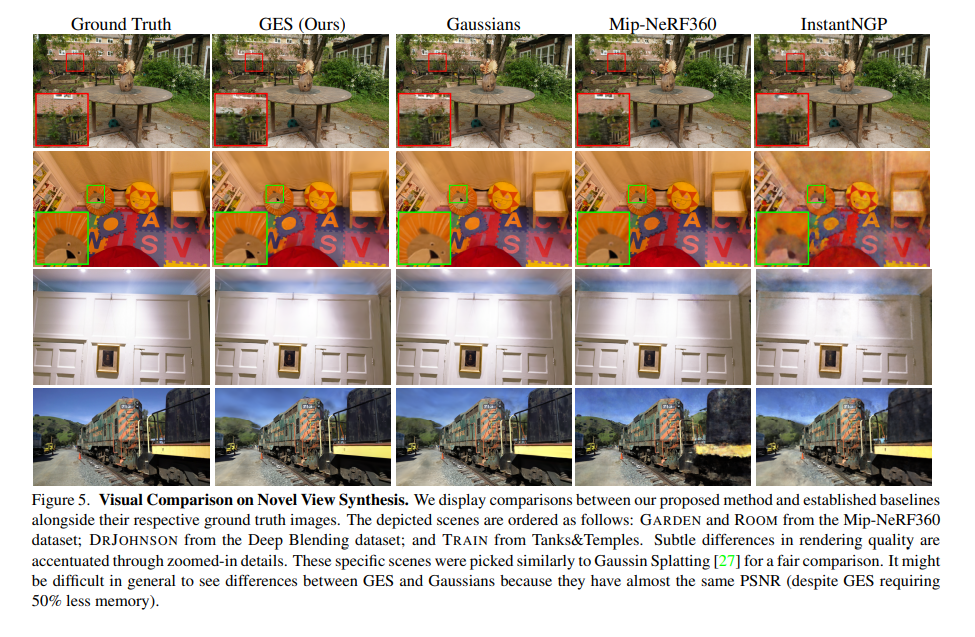
GES demonstrates exceptional efficiency and fidelity in novel view synthesis, using only 377 MB of memory and processing in 2 minutes, outperforming Gaussian methods in speed, up to a 39% increase, and memory usage, approximately less than half. of memory storage compared to Gaussian Splatting. . It stands out in the modeling of details and fine edges, improving the visual result. Fundamental to its performance is the precise approximation of the shape parameters and the implementation of a frequency modulated loss, which optimizes high contrast areas. The optimal parameter λω is set to 0.5, balancing file size reduction with performance. The integration of GES into Gaussian pipelines significantly improves the efficiency of 3D generation, showing its potential for real-time applications.
In conclusion, the research presents GES, a technique for 3D scene modeling that improves Gaussian Splatting in memory efficiency and signal representation, with demonstrated effectiveness in novel view synthesis and 3D generation tasks, but with limitations. in performance for more complex scenes. GES represents a significant leap in the field of 3D scene modeling and paves the way for more immersive and responsive virtual experiences, promising to profoundly impact various applications within the realm of 3D technology.
Review the Paper. All credit for this research goes to the researchers of this project. Also, don't forget to follow us on Twitter and Google news. Join our 38k+ ML SubReddit, 41k+ Facebook community, Discord channeland LinkedIn Grabove.
If you like our work, you will love our Newsletter..
Don't forget to join our Telegram channel
You may also like our FREE ai Courses….
Nikhil is an internal consultant at Marktechpost. He is pursuing an integrated double degree in Materials at the Indian Institute of technology Kharagpur. Nikhil is an ai/ML enthusiast who is always researching applications in fields like biomaterials and biomedical science. With a strong background in materials science, he is exploring new advances and creating opportunities to contribute.
<!– ai CONTENT END 2 –>