Portrait synthesis has become a rapidly growing field of computer graphics in recent years. If you’re wondering what portrait synthesis means, it’s an artificial intelligence (AI) task that involves an image generator. This generator is trained to produce photorealistic facial images that can be manipulated in various ways, such as haircut, clothing, poses, and pupil color. With advances in deep learning and computer vision, it is now possible to generate photorealistic 3D faces that can be used in various applications such as virtual reality, video games, and movies. Despite these advances, existing methods still face challenges in balancing the quality and editability of the generated portraits. Some methods produce low-resolution but editable faces, while others produce high-quality but non-editable faces.
Existing methods using StyleGAN aim to provide editing capabilities by learning specific addresses of attributes in the latent space or by incorporating various antecedents to create a more controlled and separate latent space. These techniques are successful in generating 2D images, but have difficulty maintaining consistency across different views when applied to editing 3D faces.
Other methods focus on neural representations to build 3D-aware generative antagonistic networks (GANs). Initially, NeRF-based generators were developed to consistently generate portraits across different views by utilizing volumetric rendering. However, this approach is memory inefficient and has limitations on the resolution and authenticity of the synthesized images. The 3D-compatible generative model presented in this article has been developed to overcome these problems.
The framework is called IDE-3D and comprises a multi-headed StyleGAN2 function generator, a neural volume renderer, and a 2D CNN-based upsampler. Below is an overview of the architecture.
Shape and texture codes are independently fed into the surface and deep layers of the StyleGAN function generator to separate different facial attributes. The resulting features are used to build 3D volumes of shape and texture, which are encoded in facial semantics and rendered in an efficient triplane representation. These volumes can then be turned into view-consistent, photorealistic portraits with freeview capability via the volume renderer and CNN 2D-based upsampler.
The authors propose a hybrid GAN inversion approach for face editing applications, which involves mapping the input image and semantic mask to latent space and editing the encoded face. The method uses a combination of optimization-based GAN inversion and texture and semantic encoders to obtain latent codes, which are used for high-fidelity reconstruction. However, the encoders’ latent output code cannot accurately reconstruct the input images and semantic masks. To address this limitation, the authors introduce a “canonical editor” that normalizes the input image to a standard view and maps it to latent space for real-time editing without sacrificing fidelity.
According to the authors, the proposed approach results in a locally unraveled, semantically aware 3D face generator, which supports interactive 3D face synthesis and editing with state-of-the-art performance (in photorealism and efficiency). The following figure offers a comparison between the proposed framework and more advanced approaches.
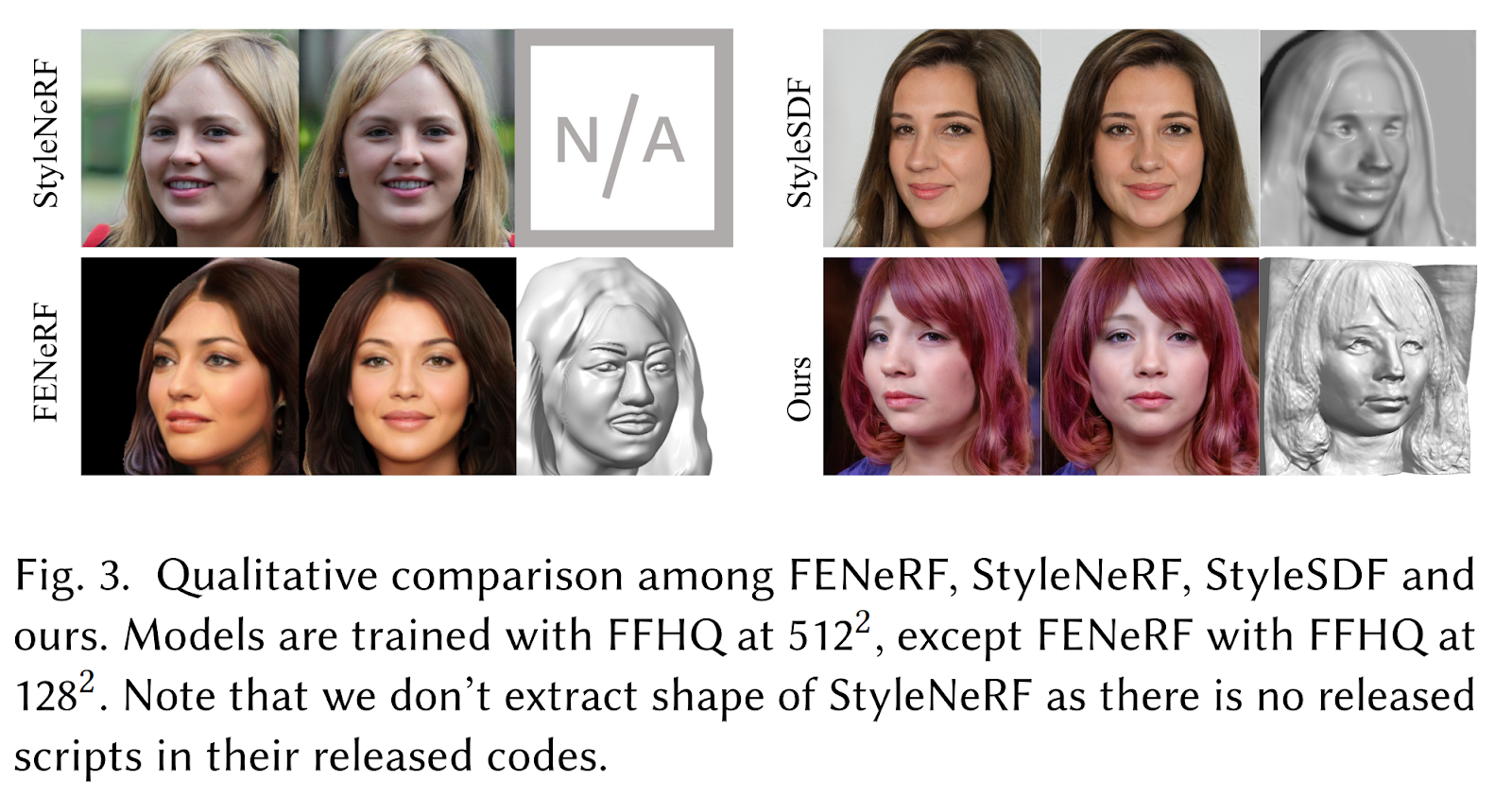
This was the brief for IDE-3D, a novel and efficient framework for the synthesis of high-resolution, photorealistic 3D portraits.
If you are interested or would like more information on this framework, you can find a link to the document and the project page.
review the Paper, Codeand project page. All credit for this research goes to the researchers of this project. Also, don’t forget to join our 13k+ ML SubReddit, discord channel, and electronic newsletterwhere we share the latest AI research news, exciting AI projects, and more.
Daniele Lorenzi received his M.Sc. in ICT for Internet and Multimedia Engineering in 2021 from the University of Padua, Italy. He is a Ph.D. candidate at the Institute of Information Technology (ITEC) at the Alpen-Adria-Universität (AAU) Klagenfurt. He currently works at the Christian Doppler ATHENA Laboratory and his research interests include adaptive video streaming, immersive media, machine learning and QoS / QoE evaluation.