Multimodal reasoning (the ability to process and integrate information from diverse data sources, such as text, images, and videos) remains a demanding area of research in artificial intelligence (ai). Despite advances, many models still struggle to achieve efficient and contextually accurate cross-modal understanding. These challenges often arise from scale limitations, narrowly focused data sets, and restricted access to advanced models. Proprietary systems, in particular, can hinder collaborative progress, leaving a gap in the development of more versatile and inclusive ai systems. The need for accessible, high-performance tools is clear as the field works toward practical, generalizable solutions.
The Qwen team has addressed these challenges by launching QvQ, an open weight model designed specifically for multimodal reasoning. Building on Qwen2-VL-72B, QvQ integrates architectural improvements that improve intermodal reasoning. Its open weight design underscores the team's commitment to making advanced ai more accessible.
Technical innovations and benefits
The QvQ architecture is designed to handle complex multimodal reasoning tasks with efficiency and accuracy. It employs a hierarchical structure that integrates visual and linguistic information while preserving contextual nuances. This design ensures that computational resources are used efficiently without sacrificing accuracy. Additionally, QvQ's alignment mechanism for visual and text input is based on advanced transformer architectures, enabling high-precision multimodal embeddings.
With 72 billion parameters, QvQ is designed for scalability and is capable of handling large and diverse data sets. The open nature of the model allows researchers to customize it for specific applications in domains such as healthcare, education, and creative industries. This flexibility makes QvQ a valuable resource for precisely addressing domain-specific challenges.
Results and insights
Preliminary evaluations show that QvQ delivers strong performance on key benchmarks in multimodal reasoning. The model has achieved notable results on datasets such as Visual7W and VQA, demonstrating its ability to process and respond to complex visual queries accurately. These results highlight how QvQ leverages the strengths of Qwen2-VL-72B while incorporating significant improvements.
One of the key strengths of QvQ is its generalizability. Unlike models that require significant adjustments for each new task, QvQ works effectively in various scenarios with minimal adjustment. Its pre-trained architecture, combined with cross-domain dataset evaluations, underlines its adaptability and potential as a universal tool for multimodal reasoning.
Conclusion
The launch of QvQ is a notable step forward in the development of advanced multimodal ai systems. By addressing critical challenges and offering an open, scalable solution, Qwen Team provides a resource that fosters collaboration and innovation. QvQ's combination of strong technical features and accessibility positions it as a valuable tool for researchers and practitioners. As its applications are further explored, QvQ has the potential to make significant contributions in several fields, enhancing ai capabilities in multimodal reasoning and beyond.
Verify he manifestation, model, and details. All credit for this research goes to the researchers of this project. Also, don't forget to follow us on <a target="_blank" href="https://twitter.com/Marktechpost”>twitter and join our Telegram channel and LinkedIn Grabove. Don't forget to join our SubReddit over 60,000 ml.
Trending: LG ai Research launches EXAONE 3.5 – three frontier-level bilingual open-source ai models that deliver unmatched instruction following and broad context understanding for global leadership in generative ai excellence….
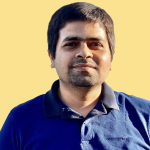
Asif Razzaq is the CEO of Marktechpost Media Inc.. As a visionary entrepreneur and engineer, Asif is committed to harnessing the potential of artificial intelligence for social good. Their most recent endeavor is the launch of an ai media platform, Marktechpost, which stands out for its in-depth coverage of machine learning and deep learning news that is technically sound and easily understandable to a wide audience. The platform has more than 2 million monthly visits, which illustrates its popularity among the public.
<script async src="//platform.twitter.com/widgets.js” charset=”utf-8″>