Proteins, the essential molecular machinery of life, play a central role in numerous biological processes. Decoding its intricate sequence, structure, and function (SSF) is a fundamental quest in biochemistry, molecular biology, and drug development. Understanding the interaction between these three aspects is crucial to discovering the principles of life at the molecular level. Computational tools have been developed to address this challenge, with alignment-based methods such as BLAST, MUSCLE, TM-align, MMseqs2 and Foldseek making significant progress. However, these tools often prioritize efficiency by focusing on local alignments, which can limit their ability to capture global insights. Furthermore, they typically operate within a single modality (sequence or structure) without integrating multiple modalities. This limitation is compounded by the fact that almost 30% of the proteins in UniProt remain unannotated because their sequences are too divergent from their known functional counterparts.
Recent advances in neural network-based tools have enabled more precise functional annotation of proteins, identifying corresponding tags for given sequences. However, these methods rely on predefined annotations and cannot interpret or generate detailed natural language descriptions of protein functions. The emergence of LLMs such as ChatGPT and LLaMA have demonstrated exceptional capabilities in natural language processing. Similarly, the rise of protein language models (PLM) has opened new avenues in computational biology. Building on these developments, the researchers propose to create a fundamental protein model that leverages advanced language modeling to comprehensively represent the SSF protein, addressing the limitations of current approaches.
ProTrek, developed by researchers at Westlake University, is a next-generation tri-modal PLM that integrates SSF. Using contrastive learning, it aligns these modalities to enable fast and accurate searches across nine SSF combinations. ProTrek outperforms existing tools like Foldseek and MMseqs2 in speed (100x) and accuracy, while outperforming ESM-2 in downstream prediction tasks. Trained with 40 million protein-text pairs, it provides global representation and learning to identify proteins with similar functions despite structural or sequence differences. With its zero-shot adjustment and recovery capabilities, ProTrek sets new benchmarks in protein research and analysis.
Descriptive data from UniProt subsections were classified at sequence level (e.g., function descriptions) and residue level (e.g., binding sites) to construct protein-function pairs. GPT-4 was used to organize data at the residue level and paraphrase descriptions at the sequence level, yielding 14 million Swiss-Prot training pairs. An initial ProTrek model was pretrained on this data set and then used to filter UniRef50, producing a final data set of 39 million pairs. The training involved InfoNCE and MLM losses, leveraging ESM-2 and PubMedBERT encoders with optimization strategies such as AdamW and DeepSpeed. ProTrek outperformed baselines in benchmarks using 4,000 Swiss-Prot proteins and 104,000 UniProt negatives, evaluated using metrics such as MAP and precision.
ProTrek represents a groundbreaking advance in protein exploration by integrating natural language sequence, structure, and function (SSF) into a sophisticated trimodal language model. Leveraging contrastive learning bridges the gap between protein data and human interpretation, enabling highly efficient searches across nine SSF pairwise modality combinations. ProTrek delivers transformative improvements, particularly in function recovery from protein sequences, achieving 30 to 60 times the performance of previous methods. It also outperforms traditional alignment tools such as Foldseek and MMseqs2, demonstrating more than 100-fold speed improvements and greater accuracy in identifying functionally similar proteins with diverse structures. Additionally, ProTrek consistently outperforms the state-of-the-art ESM-2 model, excelling in 9 of 11 downstream tasks and setting new standards in protein intelligence.
These capabilities establish ProTrek as a fundamental protein research and database analysis tool. Its remarkable performance is due to its extensive training data set, which is significantly larger than that of comparable models. ProTrek's natural language understanding capabilities go beyond conventional keyword matching approaches, enabling contextual searches and advanced applications such as text-guided protein design and protein-specific ChatGPT systems. ProTrek enables researchers to efficiently analyze vast protein databases and address complex protein-text interactions by providing superior speed, accuracy, and versatility, paving the way for significant advances in protein science and engineering.
Verify he Paper. All credit for this research goes to the researchers of this project. Also, don't forget to follow us on <a target="_blank" href="https://twitter.com/Marktechpost”>twitter and join our Telegram channel and LinkedIn Grabove. Don't forget to join our SubReddit over 60,000 ml.
UPCOMING FREE ai WEBINAR (JANUARY 15, 2025): <a target="_blank" href="https://info.gretel.ai/boost-llm-accuracy-with-sd-and-evaluation-intelligence?utm_source=marktechpost&utm_medium=newsletter&utm_campaign=202501_gretel_galileo_webinar”>Increase LLM Accuracy with Synthetic Data and Assessment Intelligence–<a target="_blank" href="https://info.gretel.ai/boost-llm-accuracy-with-sd-and-evaluation-intelligence?utm_source=marktechpost&utm_medium=newsletter&utm_campaign=202501_gretel_galileo_webinar”>Join this webinar to learn actionable insights to improve LLM model performance and accuracy while protecting data privacy..
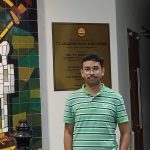
Sana Hassan, a consulting intern at Marktechpost and a dual degree student at IIT Madras, is passionate about applying technology and artificial intelligence to address real-world challenges. With a strong interest in solving practical problems, he brings a new perspective to the intersection of ai and real-life solutions.
<script async src="//platform.twitter.com/widgets.js” charset=”utf-8″>