Assessment of LLMs in medical tasks has traditionally been based on multiple-choice question benchmarks. However, these benchmarks are limited in scope, often yield saturated results with high repeat performance of LLMs, and do not accurately reflect real-world clinical scenarios. Clinical reasoning, the cognitive process that doctors use to analyze and synthesize medical data for diagnosis and treatment, is a more meaningful benchmark for evaluating model performance. Recent LLMs have demonstrated the potential to outperform clinicians in complex and routine diagnostic tasks, outperforming previous ai-based diagnostic tools that used regression models, Bayesian approaches, and rule-based systems.
Advances in LLMs, including basic models, have significantly outperformed medical professionals in diagnostic benchmarks, and strategies such as CoT have driven even further improvement of their reasoning capabilities. OpenAI's o1-preview model, introduced in September 2024, integrates a native CoT mechanism, enabling more deliberate reasoning during complex problem-solving tasks. This model has surpassed GPT-4 in addressing complex challenges such as computer science and medicine. Despite these advances, multiple-choice benchmarks fail to capture the complexity of clinical decision-making, often allowing models to leverage semantic patterns rather than genuine reasoning. Real-world clinical practice requires multi-step dynamic reasoning, where models must continually process and integrate diverse data sources, refine differential diagnoses, and make critical decisions under conditions of uncertainty.
Researchers from leading institutions, including Beth Israel Deaconess Medical Center, Stanford University, and Harvard Medical School, conducted a study to evaluate OpenAI's o1 preview model, designed to improve reasoning through chain processes of thought. The model was tested on five tasks: differential diagnosis generation, reasoning explanation, classification diagnosis, probabilistic reasoning, and management reasoning. Expert clinicians evaluated the model results using validated metrics and compared them to previous LLM and human benchmarks. The results showed significant improvements in diagnostic and management reasoning, but no progress in probabilistic reasoning or classification. The study highlights the need for robust benchmarks and real-world trials to evaluate LLM capabilities in clinical settings.
The study evaluated OpenAI's o1 preview model using various medical diagnosis cases, including NEJM Clinicopathological Conference (CPC) cases, NEJM Healers cases, Gray Matters management cases, flagship diagnostic cases, and probabilistic reasoning. Results focused on the quality of differential diagnosis, testing plans, documentation of clinical reasoning, and identification of critical diagnoses. Clinicians evaluated scores using validated metrics such as Bond Scores, R-IDEA, and standardized rubrics. Model performance was compared to historical GPT-4 controls, human benchmarks, and augmented resources. Statistical analyses, including McNemar's test and mixed effects models, were performed in R. Results highlighted the strengths of o1-preview in reasoning, but identified areas such as probabilistic reasoning that need improvement.
The study evaluated the diagnostic capabilities of o1-preview using cases from the New England Journal of Medicine (NEJM) and compared it to GPT-4 and physicians. o1-preview correctly included the diagnosis in 78.3% of NEJM cases, outperforming GPT-4 (88.6% vs. 72.9%). Achieved high test selection accuracy (87.5%) and achieved a perfect clinical reasoning score (R-IDEA) in 78/80 NEJM Healer cases, outperforming GPT-4 and physicians. In management vignettes, o1-preview outperformed GPT-4 and doctors by more than 40%. It achieved an average score of 97% on landmark diagnostic cases, comparable to the GPT-4 but higher than that of physicians. Probabilistic reasoning was performed similarly to GPT -4, with greater precision in coronary stress testing.
In conclusion, the o1-preview model demonstrated superior performance in medical reasoning in five experiments, outperforming GPT-4 and human baselines on tasks such as differential diagnosis, diagnostic reasoning, and management decisions. However, it did not show any significant improvement over GPT-4 in probabilistic reasoning or identification of critical diagnoses. These highlight the potential of LLMs in supporting clinical decisions, although real-world trials are needed to validate their integration into patient care. Current benchmarks, such as the NEJM CPCs, are nearing saturation, creating a need for more realistic and challenging assessments. Limitations include verbosity, lack of human-computer interaction studies, and a focus on internal medicine, underscoring the need for broader evaluations.
Verify he Paper. All credit for this research goes to the researchers of this project. Also, don't forget to follow us on <a target="_blank" href="https://twitter.com/Marktechpost”>twitter and join our Telegram channel and LinkedIn Grabove. Don't forget to join our SubReddit over 60,000 ml.
Trending: LG ai Research launches EXAONE 3.5 – three frontier-level bilingual open-source ai models that deliver unmatched instruction following and broad context understanding for global leadership in generative ai excellence….
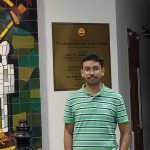
Sana Hassan, a consulting intern at Marktechpost and a dual degree student at IIT Madras, is passionate about applying technology and artificial intelligence to address real-world challenges. With a strong interest in solving practical problems, he brings a new perspective to the intersection of ai and real-life solutions.
<script async src="//platform.twitter.com/widgets.js” charset=”utf-8″>