Artificial General Intelligence (AGI) captivates the field of ai and symbolizes systems that exceed human capabilities. OpenAI, an AGI fundamental researcher, recently transitioned Q* to focus on proximal policy optimization (PPO). This change signifies the prominence of PPO as OpenAI’s enduring favorite, echoing Peter Welinder’s anticipation: “Everyone who reads about Q-learning, wait until you hear about PPO.” In this article, we delve into PPO, decode its complexities, and explore its implications for the future of AGI.
PPO Decoding
Proximate Policy Optimization (PPO), a reinforcement learning algorithm developed by OpenAI. It is a technique used in artificial intelligence, where an agent interacts with an environment to learn a task. In simple terms, let’s say the agent is trying to find the best way to play a game. PPO helps the agent learn by being careful with changes in his strategy. Instead of making big adjustments all at once, PPO makes small, cautious improvements over multiple rounds of learning. It is as if the agent is practicing and honing his gaming skills with a thoughtful and gradual approach.
PPO also pays attention to past experiences. Not only does it use all the data it has collected; select the most useful parts to learn from. This way you avoid repeating mistakes and focus on what works. Unlike traditional algorithms, PPO’s small-step updates maintain stability, which is crucial for consistent AGI system training.
Versatility in application
The versatility of PPO stands out as it strikes a delicate balance between exploration and exploitation, a critical aspect in reinforcement learning. OpenAI uses PPO in various domains, from training agents in simulated environments to mastering complex games. Its incremental policy updates ensure adaptability while limiting changes, making it indispensable in fields such as robotics, autonomous systems, and algorithmic trading.
Paving the way to AGI
OpenAI strategically leans on PPO, emphasizing a tactical approach to AGI. By leveraging PPO in games and simulations, OpenAI pushes the boundaries of ai capabilities. The acquisition of Global Illumination underscores OpenAI’s dedication to realistic agent training in simulated environments.
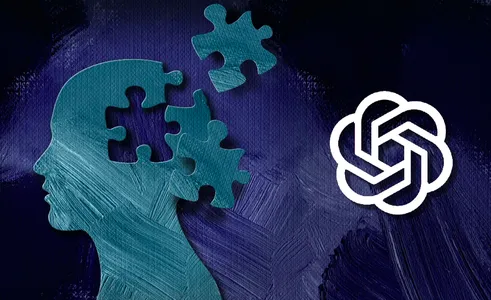
Our opinion
Since 2017, OpenAI uses PPO as the default reinforcement learning algorithm, due to its ease of use and good performance. PPO’s ability to navigate complexities, maintain stability, and adapt positions it as the cornerstone of OpenAI’s AGI. The various applications of PPO underscore its effectiveness and cement its critical role in the changing ai landscape.