LLMs have advanced significantly, showcasing their capabilities in various domains. Intelligence, a multifaceted concept, involves multiple cognitive abilities, and LLMs have brought ai closer to achieving general intelligence. Recent developments, such as OpenAI's o1 model, integrate reasoning techniques such as chain of thought (CoT) to improve problem solving. While o1 performs well on general tasks, its effectiveness in specialized areas such as medicine remains uncertain. Current benchmarks for medical LLMs often focus on narrow aspects, such as knowledge, reasoning, or safety, complicating a comprehensive evaluation of these models in complex medical tasks.
Researchers from UC Santa Cruz, the University of Edinburgh, and the National Institutes of Health evaluated OpenAI's o1 model, the first LLM to use CoT techniques with reinforcement learning. This study explored o1 performance on medical tasks, assessing comprehension, reasoning, and multilingualism across 37 medical data sets, including two new quality assurance benchmarks. The o1 model outperformed the GPT-4 in accuracy by 6.2%, but still exhibited problems such as hallucinations and inconsistent multilingual ability. The study emphasizes the need for consistent assessment metrics and improved instructional templates.
LLMs have shown notable progress in language comprehension tasks through predicting the next token and adjusting instruction. However, they often have difficulty with complex logical reasoning tasks. To overcome this, researchers introduced CoT guidance models to emulate human reasoning processes. OpenAI's o1 model, trained with extensive CoT data and reinforcement learning, aims to improve reasoning capabilities. LLMs such as GPT-4 have demonstrated strong performance in the medical domain, but domain-specific tuning is necessary for reliable clinical applications. The study investigates the potential of o1 for clinical use and shows improvements in comprehension, reasoning and multilingual abilities.
The evaluation process focuses on three key aspects of the model's capabilities: understanding, reasoning and multilingualism, in line with clinical needs. These aspects are tested on 37 data sets, covering tasks such as concept recognition, summarization, question answering, and clinical decision making. The models are guided by three stimulus strategies: direct stimulus, chain of thought and learning in few opportunities. Metrics such as accuracy, F1 score, BLEU, ROUGE, AlignScore, and Mauve evaluate model performance by comparing the generated responses with real data. These metrics measure accuracy, response similarity, objective consistency, and alignment with human-written text, ensuring comprehensive evaluation.
The experiments compare o1 with models such as GPT-3.5, GPT-4, MEDITRON-70B and Llama3-8B on medical data sets. o1 excels in clinical tasks such as concept recognition, medical summaries, and calculations, outperforming GPT-4 and GPT-3.5. It achieves notable accuracy improvements on benchmarks such as NEJMQA and LancetQA, outperforming GPT-4 by 8.9% and 27.1%, respectively. o1 also offers higher F1 and accuracy scores on tasks like BC4Chem, highlighting its superior medical knowledge and reasoning ability and positioning it as a promising tool for real-world clinical applications.
The o1 model demonstrates significant progress in general NLP and in the medical field, but it has certain drawbacks. Its longer decoding time (more than twice that of GPT-4 and nine times that of GPT-3.5) can cause delays in complex tasks. Furthermore, o1's performance is inconsistent across different tasks and underperforms on simpler tasks such as concept recognition. Traditional metrics like BLEU and ROUGE may not adequately evaluate your production, especially in specialized medical fields. Future assessments require improved metrics and stimulation techniques to better capture their capabilities and mitigate limitations such as hallucinations and factual accuracy.
look at the Paper and Project. All credit for this research goes to the researchers of this project. Also, don't forget to follow us on twitter.com/Marktechpost”>twitter and join our Telegram channel and LinkedIn Grabove. If you like our work, you will love our information sheet..
Don't forget to join our SubReddit over 50,000ml
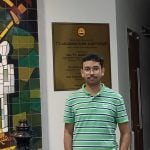
Sana Hassan, a consulting intern at Marktechpost and a dual degree student at IIT Madras, is passionate about applying technology and artificial intelligence to address real-world challenges. With a strong interest in solving practical problems, he brings a new perspective to the intersection of ai and real-life solutions.
<script async src="//platform.twitter.com/widgets.js” charset=”utf-8″>