Multimodal Large Language Models (MLLM) bridge vision and language, enabling effective interpretation of visual content. However, achieving an accurate and scalable regional understanding of static images and dynamic videos remains a challenge. Temporal inconsistencies, scale inefficiencies, and limited video understanding hinder progress, particularly in maintaining consistent representations of objects and regions across video frames. Temporal drift, caused by changes in motion, scale, or perspective, along with reliance on computationally heavy methods such as bounding boxes or features aligned to regions of interest (RoI), increases complexity and limits real-time video analysis. and on a large scale.
Recent strategies, such as textual region coordinates, visual markers, and RoI-based features, have attempted to address these issues. However, they often fail to ensure temporal consistency between frames or efficiently process large data sets. Bounding boxes lack robustness for tracking multiple frames, and static frame analysis ignores intricate temporal relationships. While innovations such as incorporating coordinates into textual prompts and using image-based markers have advanced the field, a unified solution for the image and video domains remains out of reach.
Researchers at NVIDIA and Yonsei University developed Omni-RGPTa novel multimodal big language model designed for regionally seamless understanding in images and videos to address these challenges. This model introduces symbolic markan innovative method that incorporates region-specific tokens into visual and text cues, establishing a unified connection between the two modalities. The Token Mark system replaces traditional RoI-based approaches by defining a unique token for each target region, which remains consistent across all frames of a video. This strategy avoids temporal drift and reduces computational costs, enabling robust reasoning for static and dynamic inputs. The inclusion of a temporal region guidance head further improves the model's performance on video data when classifying visual tokens to avoid reliance on complex tracking mechanisms.
Omni-RGPT leverages a newly created large-scale dataset called RegVID-300k, which contains 98,000 unique videos, 214,000 annotated regions, and 294,000 region-level instruction samples. This dataset was built by combining data from ten public video datasets, offering diverse and detailed instructions for region-specific tasks. The dataset supports common sense visual reasoning, region-based captioning, and reference expression understanding. Unlike other datasets, RegVID-300k includes detailed captions with temporal context and mitigates visual hallucinations using advanced validation techniques.
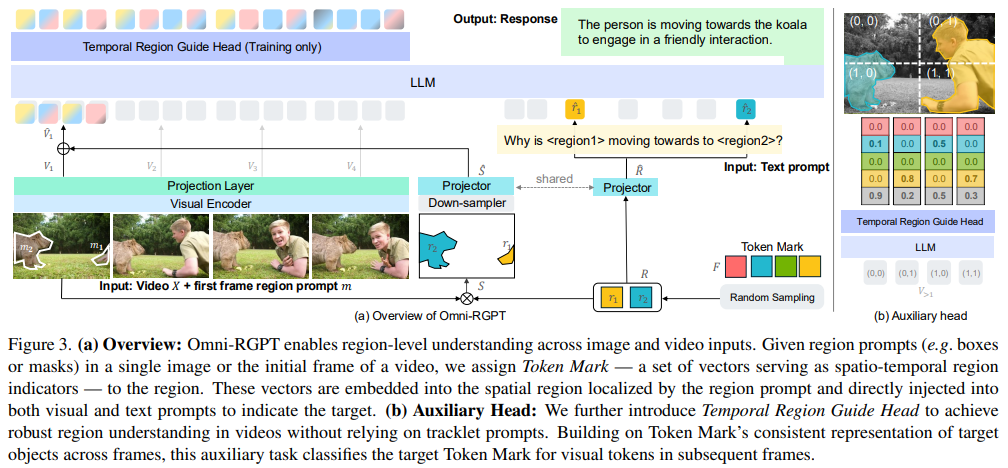
Omni-RGPT achieved state-of-the-art results on several benchmarks, including 84.5% accuracy on the Causal-VidQA dataset, which evaluates temporal and spatial reasoning in video sequences. The model outperformed existing methods such as MotionEpic by more than 5% on some subtasks, demonstrating superior performance in prediction and counterfactual reasoning. Similarly, the model excelled in video captioning tasks, achieving high METEOR scores on challenging data sets such as Vid-STG and BenSMOT. The model achieved remarkable accuracy for image-based tasks on the Visual Commonsense Reasoning (VCR) dataset, outperforming methods specifically optimized for image domains.
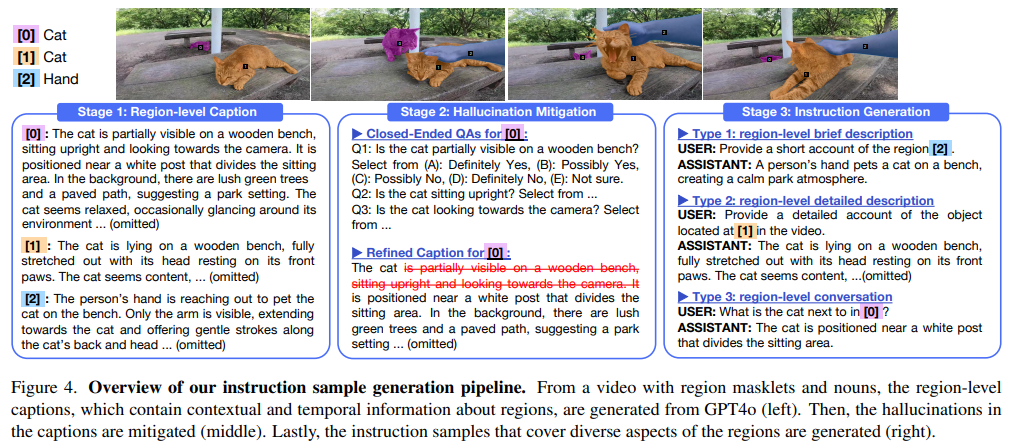
Several key takeaways from the Omni-RGPT research include:
- This approach enables regionally consistent and scalable understanding by incorporating predefined tokens into visual and text inputs. This prevents temporal drift and supports fluid reasoning between frames.
- The dataset provides diverse, rich, and detailed annotations, allowing the model to excel in complex video tasks. It includes 294,000 instructions at the regional level and addresses gaps in existing data sets.
- Omni-RGPT demonstrated superior performance on benchmarks such as Causal-VidQA and VCR, achieving accuracy improvements of up to 5% compared to leading models.
- The model design reduces computational overhead by avoiding dependence on bounding box coordinates or entire video tracklets, making it suitable for real-world applications.
- The framework seamlessly integrates image and video tasks under a single architecture, achieving exceptional performance without compromising efficiency.
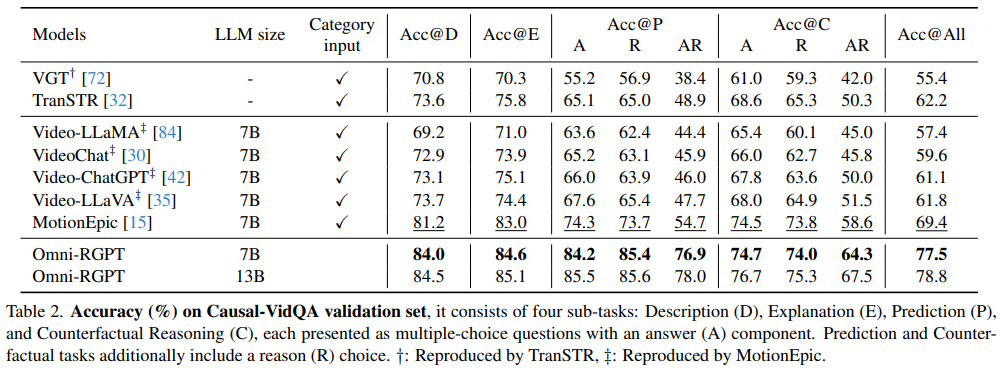
In conclusion, Omni-RGPT addresses critical challenges in region-specific multimodal learning by introducing Token Mark and a novel dataset to support fine-grained understanding in images and videos. The model's scalable design and state-of-the-art performance on various tasks set a new benchmark in this field. Omni-RGPT provides a solid foundation for future research and practical applications in ai by eliminating temporal drift, reducing computational complexity, and leveraging large-scale data.
Verify he Paper and Project page. All credit for this research goes to the researchers of this project. Also, don't forget to follow us on <a target="_blank" href="https://x.com/intent/follow?screen_name=marktechpost” target=”_blank” rel=”noreferrer noopener”>twitter and join our Telegram channel and LinkedIn Grabove. Don't forget to join our SubReddit over 65,000 ml.
Recommend open source platform: Parlant is a framework that transforms the way ai agents make decisions in customer-facing scenarios. (Promoted)
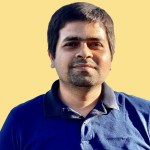
Asif Razzaq is the CEO of Marktechpost Media Inc.. As a visionary entrepreneur and engineer, Asif is committed to harnessing the potential of artificial intelligence for social good. Their most recent endeavor is the launch of an ai media platform, Marktechpost, which stands out for its in-depth coverage of machine learning and deep learning news that is technically sound and easily understandable to a wide audience. The platform has more than 2 million monthly visits, which illustrates its popularity among the public.