Due to recent advances in the underlying modeling methods, generative image models have attracted interest like never before. The most effective models today are based on diffusion models, autoregressive transformers, and generative antagonistic networks. Particularly desired features of diffusion models (DMs) include their robust and scalable training target and tendency to need fewer parameters than their transformer-based counterparts. The scarcity of large-scale, generic, and publicly accessible video data sets and the high computational cost involved with training on video data are the key reasons why video modeling has lagged behind. At the same time, image mastery has made great strides.
Although there is a large body of research on video synthesis, most efforts, including the video DMs above, only produce short, low-resolution movies. They create high-resolution extended films by applying video models to real problems. They focus on two pertinent real-world video generation issues: (i) text-driven video synthesis for producing creative content and (ii) high-resolution real-world driving data video synthesis, which has great potential. as a simulation engine in autonomous vehicles. driving. To do this, they rely on latent diffusion models (LDMs), which can decrease significant computational load when learning from high-resolution images.
They generate temporally coherent videos using previously trained image diffusion models. The model first generates a batch of samples that are independent of each other. The samples are temporally aligned and create coherent movies after fine-tuning the temporal video.
Researchers from LMU Munich, NVIDIA, Vector Institute, the University of Toronto, and the University of Waterloo recommend Video LDM and expand LDM to high-resolution video creation, a process that requires a lot of computing power. Unlike previous research on video DMs, their video BOMs are initially pretrained on images exclusively (or use existing pretrained image BOMs), allowing us to leverage large image data sets. After adding a time dimension to the latent spatial DM, they turn the LDM image generator into a video generator by fixing the pretrained spatial layers and training only the temporal layers on image sequences or encoded movies (Fig. 1). To establish temporal consistency in pixel space. They tune the LDM decoder in a similar way (Fig. 2).
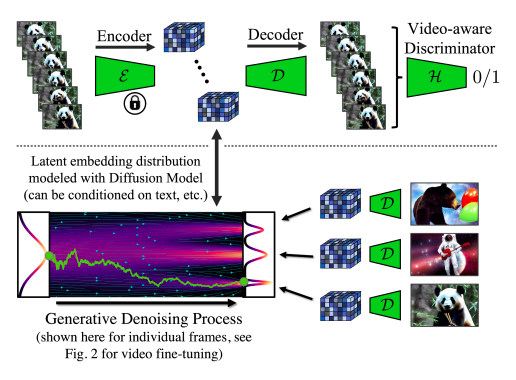
They also temporally align pixel space and latent DM samplers, which are frequently used for image super-resolution, by converting them to time-consistent video super-resolution models to further improve spatial resolution. His approach, which is based on LDM, can produce large, globally consistent movies using little memory and processing power. The video sampler only has to work locally for synthesis at extremely high resolutions, creating little training and computing demands. To achieve state-of-the-art video quality, they test their technology using 5121024 movies of real driving scenarios and synthesize videos of several minutes in length.
In addition, they enhance a powerful text-to-image BOM known as stable casting so that it can be used to create text-to-video up to 1280 x 2048 resolution. They can use a reasonably small training set of subtitled movies. since they need to train the time alignment layers in such a scenario. Introducing the first instance of custom text-to-video creation by transferring learned temporal layers to variously configured text-to-image BOMs. They anticipate that their work will pave the way for more effective digital content generation and autonomous driving simulation.
The following are their contributions:
(i) They provide a practical method for developing BOM-based video production models with high resolution and long-term consistency. Their significant discovery is using DMs of pretrained images to generate video by adding temporal layers that can train images to align consistently over time (Figs 1 and 2).
(ii) They further refine super-resolution MDs, which are widely used in the timing literature.
(iii) They can produce multi-minute movies and achieve state-of-the-art high-resolution video synthesis performance on recordings of real driving scenarios.
They (i) upgrade the publicly accessible broadcast stable text-to-image BOM to a robust and expressive text-to-video BOM (ii), (iii) show that learned temporal layers can be integrated with other control model checkpoints. image (such as DreamBooth), and (iv) do the same for the learned temporary layers.
review the Paper and Project. Don’t forget to join our 19k+ ML SubReddit, discord channel, and electronic newsletter, where we share the latest AI research news, exciting AI projects, and more. If you have any questions about the article above or if we missed anything, feel free to email us at [email protected]
🚀 Check out 100 AI tools at AI Tools Club
Aneesh Tickoo is a consulting intern at MarktechPost. She is currently pursuing her bachelor’s degree in Information Science and Artificial Intelligence at the Indian Institute of Technology (IIT), Bhilai. She spends most of her time working on projects aimed at harnessing the power of machine learning. Her research interest is image processing and she is passionate about creating solutions around her. She loves connecting with people and collaborating on interesting projects.