Video editing, the process of manipulating and rearranging video clips to meet desired goals, has been revolutionized by the integration of artificial intelligence (AI) into computing. AI-powered video editing tools enable faster and more efficient post-production processes. With the advancement of deep learning algorithms, AI can now automatically perform tasks like color correction, object tracking, and even content creation. By analyzing patterns in video data, AI can suggest edits and transitions that would improve the overall appearance of the final product. Additionally, AI-based tools can help organize and categorize large video libraries, making it easier for publishers to find the material they need. The use of AI in video editing has the potential to significantly reduce the time and effort required to produce high-quality video content, while also enabling new creative possibilities.
The use of GAN in text-guided image synthesis and manipulation has experienced significant advances in recent years. Text-to-image generation models, such as DALL-E, and recent methods using pretrained CLIP embedding have proven successful. Diffusion models, such as Stable Diffusion, have also been successful in text-guided image generation and editing, leading to various creative applications. However, for video editing, more than spatial fidelity is required, and that is temporal consistency.
The work presented in this article extends the semantic image editing capabilities of the next-generation Stable Diffusion text-to-image model to consistent video editing.
The pipeline for the proposed architecture is shown below.
Given an input video and a text prompt, the proposed shape-aware video editing method produces consistent video with appearance and shape changes while preserving motion in the input video. For temporal consistency, the approach uses a pre-trained NLA (non-linear atlas) to decompose the input video into unified background (BG) and foreground (FG) atlases with associated per-frame UV mapping. After the video has been decomposed, a single keyframe of the video is manipulated using a text-to-image diffusion model (stable diffusion). The model took advantage of this edited keyframe to estimate the dense semantic correspondence between the input and edited keyframes, allowing shape deformation to be performed. This step is very tricky, as it produces the shape warp vector applied to the target image to maintain temporal consistency. This shape warp serves as the basis for the per-frame warp, since UV mapping and atlas are used to associate edits with each frame. Additionally, a pre-trained broadcast model is exploited to ensure that the output video is perfect with no hidden pixels.
According to the authors, the proposed approach results in a reliable video editing tool that consistently delivers the desired look and feel. The following figure offers a comparison between the proposed framework and more advanced approaches.
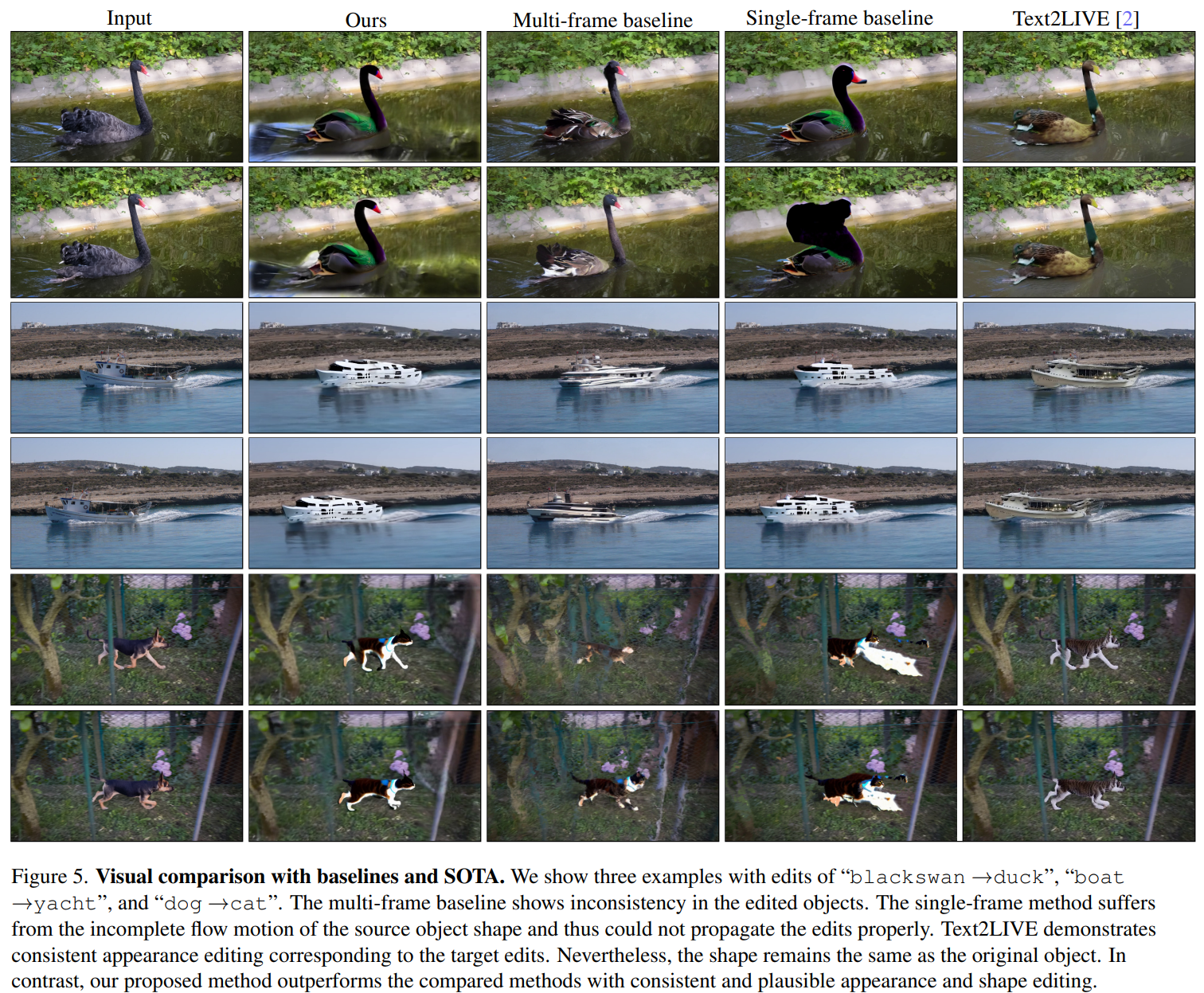
This was the brief for a new AI tool for accurate and consistent text-based video editing.
If you are interested or would like more information on this framework, you can find a link to the document and the project page.
review the Paper and Project. All credit for this research goes to the researchers of this project. Also, don’t forget to join our 14k+ ML SubReddit, discord channel, and electronic newsletterwhere we share the latest AI research news, exciting AI projects, and more.
Daniele Lorenzi received his M.Sc. in ICT for Internet and Multimedia Engineering in 2021 from the University of Padua, Italy. He is a Ph.D. candidate at the Institute of Information Technology (ITEC) at the Alpen-Adria-Universität (AAU) Klagenfurt. He currently works at the Christian Doppler ATHENA Laboratory and his research interests include adaptive video streaming, immersive media, machine learning and QoS / QoE evaluation.