The challenges of generative modeling in generating motion controllable videos present significant obstacles to research. Current approaches in video generation struggle with precise control of motion in various scenarios. The field uses three main motion control techniques: local object motion control using bounding boxes or masks, global parameterization of camera motion, and motion transfer from reference videos. Despite these approaches, researchers have identified critical limitations including complex model modifications, difficulties in acquiring precise motion parameters, and the fundamental trade-off between motion control accuracy and spatiotemporal visual quality. Existing methods often require technical interventions that restrict their generalization and practical applicability in different video generation contexts.
Existing research on motion controllable video generation has explored multiple methodological approaches to address motion control challenges. Image and video diffusion models have used techniques such as noise warping and temporal attention tuning to improve video generation capabilities. Noise warping methods such as HIWYN attempt to create temporally correlated latent noise, although they suffer from spatial Gaussianity preservation issues and computational complexity. Advanced video diffusion models such as AnimateDiff and CogVideoX have made significant progress by fine-tuning temporal attention layers and combining spatial and temporal encoding strategies. Additionally, motion control approaches have focused on local object motion control, global parameterization of camera motion, and motion transfer from reference videos.
Researchers from Netflix Eyeline Studios, Netflix, Stony Brook University, University of Maryland, and Stanford University have proposed a novel approach to improve motion control in video broadcast models. Their method introduces a structured latent noise sampling technique that transforms video generation by preprocessing training videos to produce structured noise. Unlike existing approaches, this technique requires no modifications to model architectures or training pipelines, making it uniquely adaptable to different diffusion models. This innovative approach provides a solution for motion control, including local object motion, global camera motion, and motion transfer with improved temporal coherence and pixel-per-frame quality.
The proposed method consists of two main components: a noise deformation algorithm and fine-tuning of the video diffusion. The noise warping algorithm operates independently of the diffusion model training process, generating noise patterns used to train the diffusion model without introducing additional parameters to the video diffusion model. Inspired by existing noise warping techniques, researchers use warped noise as a motion conditioning mechanism for video generation models. The method fine-tunes state-of-the-art video diffusion models such as CogVideoX-5B, using a huge general-purpose video dataset of 4 million videos with resolutions of 720×480 or higher. Furthermore, the approach is data- and model-independent, allowing adaptation of motion control across various video broadcast models.
The experimental results demonstrate the effectiveness and efficiency of the proposed method through multiple evaluation metrics. Statistical analysis using Moran's I index reveals that the method achieved an exceptionally low spatial cross-correlation value of 0.00014, with a high p-value of 0.84, indicating excellent preservation of spatial Gaussianity. The Kolmogorov-Smirnov (KS) test further validates the performance of the method, obtaining a KS statistic of 0.060 and a p-value of 0.44, suggesting that the warped noise closely follows a standard normal distribution. Performance efficiency tests performed on a 40 GB NVIDIA A100 GPU show that the proposed method outperforms existing baselines and runs 26 times faster than the most recently published approach.
In conclusion, the proposed method represents a significant advance in motion controllable video generation, addressing critical challenges in generative modeling. Researchers have developed a perfect approach to incorporate motion control into video broadcast noise sampling. This innovative technique transforms the video generation landscape by providing a unified paradigm for easy-to-use motion control across diverse applications. The method bridges the gap between random noise and structured outputs, allowing precise manipulation of video motion without compromising visual quality or computational efficiency. Additionally, this method excels in motion control, temporal consistency, and visual fidelity, positioning itself as a robust and versatile solution for next-generation video broadcast models.
Verify he Paper and Project page. All credit for this research goes to the researchers of this project. Also, don't forget to follow us on <a target="_blank" href="https://x.com/intent/follow?screen_name=marktechpost” target=”_blank” rel=”noreferrer noopener”>twitter and join our Telegram channel and LinkedIn Grabove. Don't forget to join our SubReddit over 70,000 ml.
<a target="_blank" href="https://nebius.com/blog/posts/studio-embeddings-vision-and-language-models?utm_medium=newsletter&utm_source=marktechpost&utm_campaign=embedding-post-ai-studio” target=”_blank” rel=”noreferrer noopener”> (Recommended Reading) Nebius ai Studio Expands with Vision Models, New Language Models, Embeddings, and LoRA (Promoted)
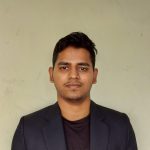
Sajjad Ansari is a final year student of IIT Kharagpur. As a technology enthusiast, he delves into the practical applications of ai with a focus on understanding the impact of ai technologies and their real-world implications. Its goal is to articulate complex ai concepts in a clear and accessible way.