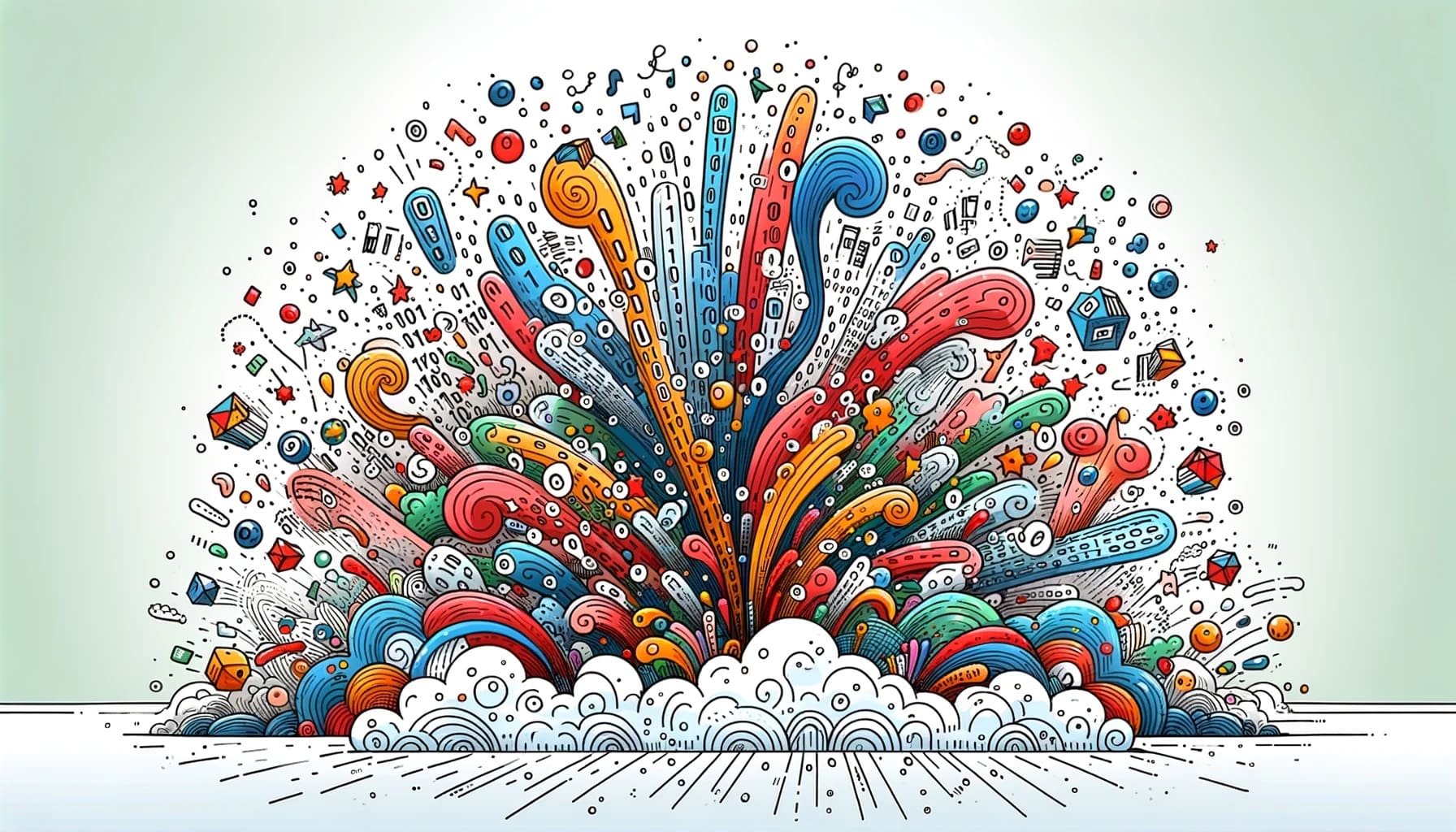
Image generated with DALLE-3
In the ever-evolving technology landscape, the data revolution is emerging as a formidable force reshaping the fabric of industries, economies and social norms. Data science and machine learning are at the center of this transformative drive and serve as crucial catalysts for innovation. They propel us toward an era in which problem solving transcends mere human cognition and evolves into a collaborative dance between human intellect and intelligent machines. This article embarks on a comprehensive journey, delving into emerging trends within data science and machine learning, uncovering the fundamental developments that are leading us towards a data-driven future.
A major trend in data science and machine learning revolves around incorporating artificial intelligence (ai) to drive automation. Industries across the spectrum are harnessing the potential of machine learning algorithms to optimize everyday tasks, fine-tune processes, and increase efficiency. Whether in manufacturing, healthcare, finance or logistics, the wave of ai-driven automation is fundamentally transforming the operational landscape of businesses. This shift reduces costs and raises overall productivity, marking a revolutionary step in the way businesses conduct their daily functions.
Use cases
- Finance:
In finance, automated trading systems have taken center stage, employing the power of machine learning to analyze market trends and execute trades seamlessly in real time. It is a sophisticated technological integration into the dynamic realm of financial markets, ushering in a new era of efficiency and data-driven decision making.
Picture of ai-in-the-finance-sector/” rel=”noopener” target=”_blank”>AISmartz
- Health care:
In the healthcare sector, the incredible capabilities of machine learning algorithms are taking on critical roles. These algorithms aid in diagnosis, provide insights into predictive analytics of patient outcomes, and even contribute to the accuracy of robotic surgeries. It is a remarkable fusion of technology and medicine that is reshaping the landscape of patient care.
Natural language processing (NLP) has taken center stage in the broad field of machine learning. Thanks to advances in deep learning models like GPT-3, machines are evolving rapidly and displaying a remarkable ability to decipher and generate language that mimics human expression. This transformative trend is reshaping the way we interact with technology, from the intuitive responses of chatbots and virtual assistants to the complexities of language translation and content creation. The new ability of machines to capture and respond to natural language not only redefines our communication landscape, but also opens new avenues to improve accessibility in various areas.
Use cases
- Content generation:
Models like GPT-3 have transformed the landscape of the content creation and writing industries by producing texts that resemble human language. Its influence is palpable and marks the beginning of a new era in which artificial intelligence collaborates with writers to create engaging and coherent content.
Picture of ai-content-creation/” rel=”noopener” target=”_blank”>AnalysisVidhya
- Chatbots and virtual assistants:
Natural language processing (NLP) plays a critical role in the functionality of chatbots like Siri and virtual assistants like Alexa. It's the magic behind its ability to understand and respond to our everyday linguistic queries, making interactions more human and intuitive.
- Language translation:
In language translation, Google Translate relies on the finesse of natural language processing (NLP) to deliver precise and accurate translations in multiple languages. This sophisticated use of technology makes fluid communication across linguistic boundaries possible.
In the ever-evolving decision-making landscape, the critical role of data cannot be underestimated. What is increasingly in the spotlight is the imperative for ethical considerations in ai and data science. There is a notable increase in the recognition of ethical principles as integral elements in the development and deployment phases of machine learning models. Issues such as bias, equity, transparency and accountability have come to the fore in debates, shaping the narrative around responsible data science practices. Organizations are actively embracing this ethical shift, adopting frameworks and guidelines that seek to strike a delicate balance between innovation and ethical considerations, steering the course toward a more data-aware era.
Use cases
- Face recognition:
The ethical landscape surrounding facial recognition technology is complex, primarily due to potential biases inherent in the system. This has created a pressing need for thoughtful and responsible deployment, as the consequences of biased facial recognition can have profound implications for privacy, security, and social justice.
- Credit score:
Navigating the terrain of credit scoring with machine learning requires meticulous consideration, as the models involved must be precisely designed to mitigate any potential discriminatory practices. This thoughtful approach is crucial to ensuring fairness and equity in lending practices, recognizing the significant impact of these models on people's financial opportunities.
The widespread adoption of Internet of Things (IoT) devices has triggered a notable increase in data generation right at the edge of networks. One trend that is gaining significant traction is the fusion of edge computing with decentralized machine learning aimed at processing data close to its source. This strategic move promises to curb latency and optimize bandwidth usage. Its relevance is especially pronounced in sectors such as autonomous vehicles, smart cities and industrial IoT, where split-second decision making is paramount. Integrating machine learning models into edge devices is critical to fostering systems that are intelligent and highly responsive to real-time demands.
Use cases
- Autonomous vehicles:
In the autonomous vehicle space, edge computing has proven to be transformative. Enabling rapid processing of data directly from sensors allows these vehicles to make quick decisions, improving their ability to navigate the road with agility and ensuring a level of responsiveness critical to their safe and efficient operation.
- Smart cities:
The incorporation of decentralized machine learning in smart city applications marks an important advance. This innovation facilitates real-time data analysis from various sensors, contributing to overall city efficiency by providing timely information for better decision-making and resource allocation. It exemplifies the seamless integration of technology to create smarter and more responsive urban environments.
Picture of ai-can-help-smart-city-initiatives-f83484891343″ rel=”noopener” target=”_blank”>Towards Data Science
The data science and machine learning landscape is expanding beyond traditional boundaries and evolving into an interdisciplinary domain. There is a notable trend where professionals from diverse backgrounds are collaborating seamlessly to address complex problems. The demand for hybrid skill sets, combining data science competency, domain-specific knowledge and effective communication, is steadily increasing. In this interconnected data ecosystem, professionals skilled in bridging the gap between technical complexities and understanding non-technical stakeholders are emerging as increasingly invaluable assets.
Use cases
- Health analysis:
In the intricate realm of healthcare, dynamic collaboration is developing as data scientists and healthcare professionals join forces. Together, they sift through vast amounts of patient data, applying their combined expertise to gain valuable insights that improve treatment outcomes and usher in a new era of effective, personalized healthcare solutions.
- Finance and data analysis:
Collaboration arises at the intersection of finance and data science when professionals with dual expertise join forces. Together, they channel their insights to create predictive models that delve into the intricate web of market trends, exemplifying a harmonious combination of financial acumen and data-driven insights.
Driven by data science and machine learning, the ongoing data revolution is fundamentally reshaping our daily lives and professional landscapes. Whether it is the advent of ai-driven automation, the growing emphasis on ethical considerations, or the collaborative synergy of interdisciplinary approaches, the trends discussed provide a nuanced view of the dynamic and ever-evolving nature of these fields. Successfully navigating this revolution requires a strong commitment to staying abreast of advances, adopting responsible practices, and cultivating a culture of perpetual learning. Looking ahead, the convergence of data science and machine learning promises to unlock new possibilities, continually driving innovation across various industries.
Aryan Garg It is a B.tech. Electrical Engineering student, currently in the last year of the degree. His interest lies in the field of Web Development and Machine Learning. He has pursued this interest and is eager to work further in these directions.