Music generation has long been a fascinating domain, combining creativity with technology to produce compositions that resonate with human emotions. The process involves generating music that aligns with specific themes or emotions conveyed through textual descriptions. While the development of music from text has seen notable progress, a major challenge remains: editing the generated music to refine or alter specific elements without starting from scratch. This task involves complex adjustments to the attributes of the music, such as changing the sound of an instrument or the overall mood of the piece, without affecting its core structure.
The models are mainly divided into autoregressive (AR) and diffusion-based categories. AR models produce longer, higher-quality audio at the cost of longer inference times, and diffusion models excel at parallel decoding despite challenges in generating extended sequences. The innovative MagNet model combines the advantages of AR and broadcast, optimizing quality and efficiency. While models like InstructME and M2UGen demonstrate cross- and intra-stem editing capabilities, Loop Copilot facilitates compositional editing without altering the architecture or interface of the original models.
Researchers from QMU London, Sony ai and MBZUAI have introduced a novel approach called MusicMagus. This approach offers a sophisticated yet easy-to-use solution for editing music generated from text descriptions. By leveraging advanced diffusion models, MusicMagus enables precise modifications of specific musical attributes while maintaining the integrity of the original composition.
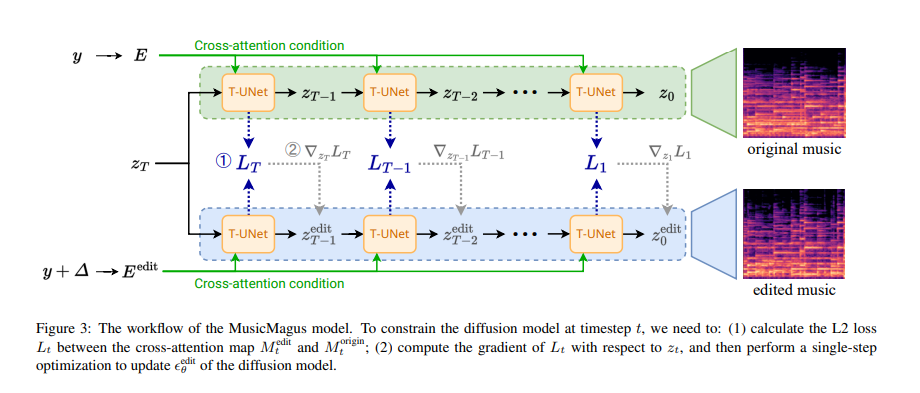
MusicMagus showcases its unrivaled ability to edit and refine music through sophisticated methodologies and innovative use of data sets. The backbone of the system is based on the prowess of the AudioLDM 2 model, which uses a variational autoencoder (VAE) framework to compress musical audio spectrograms into latent space. This space is then manipulated to generate or edit music based on textual descriptions, bridging the gap between textual input and musical production. MusicMagus' editing mechanism leverages the latent capabilities of pre-trained diffusion-based models, a novel approach that significantly improves its editing accuracy and flexibility.
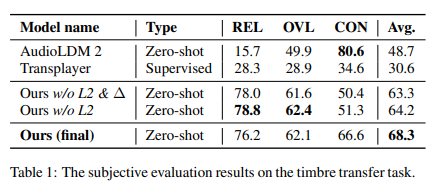
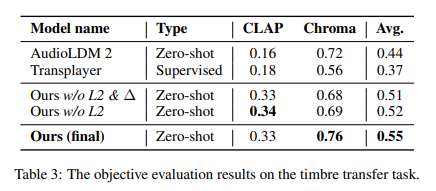
The researchers conducted extensive experiments to validate the effectiveness of MusicMagus, involving critical tasks such as timbre and style transfer, comparing its performance to established baselines such as AudioLDM 2, Transplayer and MusicGen. These comparative analyzes are based on the use of metrics such as CLAP Similarity and Chromagram Similarity for objective evaluations and Overall Quality (OVL), Relevance (REL) and Structural Consistency (CON) for subjective evaluations. The results reveal that MusicMagus outperforms the baselines with a notable increase in CLAP similarity score of up to 0.33 and chromagram similarity of 0.77, indicating significant progress in maintaining semantic integrity and coherence. structural of music. The data sets used in these experiments, including POP909 and MAESTRO for the timbre transfer task, have played a crucial role in demonstrating the superior capabilities of MusicMagus to alter musical semantics while preserving the essence of the composition. original.
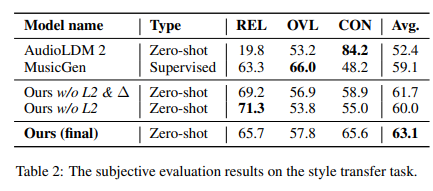
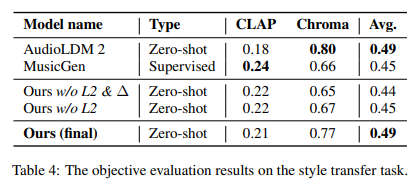
In conclusion, MusicMagus presents a pioneering text-to-music editing framework capable of manipulating specific musical aspects while preserving the integrity of the composition. Although it faces challenges with generating multi-instrument music, trade-offs between editability versus fidelity, and maintaining structure during substantial changes, it marks a significant advance in music editing technology. Despite its limitations in handling long sequences and being limited to a 16 kHz sampling rate, MusicMagus significantly advances next-generation stylistic and timbre transfer, showcasing its innovative approach to music editing.
Review the Paper. All credit for this research goes to the researchers of this project. Also, don't forget to follow us on Twitter. Join our 37k+ ML SubReddit, 41k+ Facebook community, Discord Channeland LinkedIn Grabove.
If you like our work, you will love our Newsletter..
Don't forget to join our Telegram channel
Nikhil is an internal consultant at Marktechpost. He is pursuing an integrated double degree in Materials at the Indian Institute of technology Kharagpur. Nikhil is an ai/ML enthusiast who is always researching applications in fields like biomaterials and biomedical science. With a strong background in materials science, he is exploring new advances and creating opportunities to contribute.
<!– ai CONTENT END 2 –>