Document understanding (DU) focuses on the automatic interpretation and processing of documents, which encompass complex layout structures and multimodal elements such as text, tables, graphics, and images. This task is essential to extract and use the vast amounts of information contained in the documents generated annually.
One of the critical challenges lies in understanding long-context documents that span many pages and require understanding across multiple modalities and pages. Traditional single-page DU models struggle with this, so it is crucial to develop benchmarks to evaluate the performance of models on long documents. Researchers have identified that these long-context documents require specific capabilities, such as localization and cross-page understanding, which are not adequately addressed in current single-page DU datasets.
Current methods for DU include large vision language models (LVLMs) such as GPT-4o, Gemini-1.5, and Claude-3, developed by companies like OpenAI and Anthropic. These models have shown promise on single-page tasks, but need help with understanding large-context documents due to the need for multi-page understanding and integration of multimodal elements. This gap in capability underscores the importance of creating comprehensive benchmarks to drive the development of more advanced models.
Researchers from institutions including Nanyang Technological University, Shanghai artificial intelligence Lab, and Peking University have introduced MMLongBench-Doc, a comprehensive benchmark designed to evaluate the long-context DU capabilities of LVLMs. This benchmark includes 135 PDF documents from various domains, with an average of 47.5 pages and 21,214.1 textual tokens. It includes 1,091 questions requiring evidence from text, images, graphs, tables, and layout structures, with a significant portion requiring cross-page understanding. This rigorous benchmark aims to push the boundaries of current DU models.
In depth, the methodology involves using screenshots of document pages as inputs for MMLongBench-Doc, comparing their performance to traditional OCR-parsed text models. The benchmark construction was meticulous, with ten expert annotators editing questions from existing datasets and creating new ones to make them more comprehensive. The annotation process ensured high quality through a three-round semi-automated review process. This approach highlighted the need for models to handle long documents comprehensively, making MMLongBench-Doc a critical tool for evaluating and improving DU models.
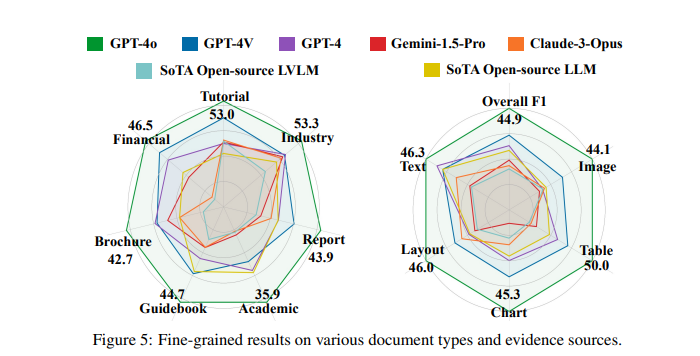
Performance evaluations revealed that LVLMs generally struggle with long-context DU. For example, the best-performing model, GPT-4o, achieved an F1 score of 44.9%, while the second-best, GPT-4V, scored 30.5%. Other models, such as Gemini-1.5 and Claude-3, showed even lower performance. These results indicate the substantial challenges presented by long-context DU and the need for further advancements. The study compared these results to OCR-based models and observed that some LVLMs performed worse than monomodal LLMs when fed with text parsed by lossy OCR.
The detailed results highlighted that while LVLMs can handle multimodal inputs to some extent, their capabilities still need to be improved. For example, 33.0% of the questions in the benchmark were multi-page questions requiring comprehension of multiple pages, and 22.5% were designed to be unanswerable to detect potential hallucinations. This rigorous testing underlined the need for more capable LVLMs. Proprietary models outperformed open-source ones, attributing to their higher numbers of acceptable images and maximum image resolutions.
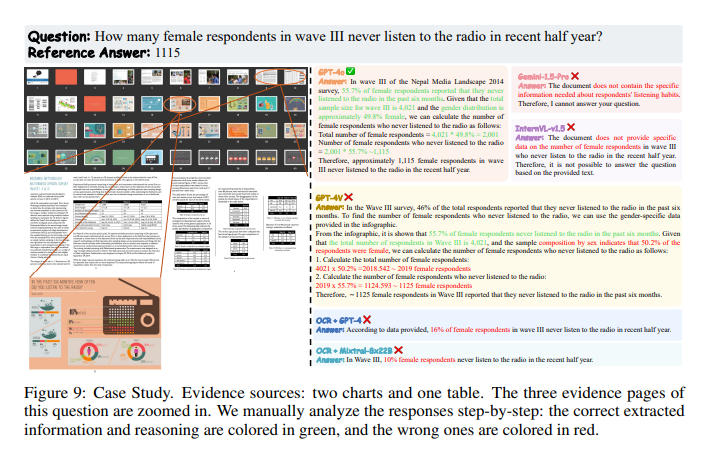
In conclusion, this study highlights the complexity of long-context document understanding and the need for advanced models capable of effectively processing and understanding long, multimodal documents. The MMLongBench-Doc benchmark, developed in collaboration with leading research institutions, is a valuable tool to evaluate and improve the performance of these models. The study findings highlight the significant challenges of current models and the need for continued research and development in this area to achieve more effective and comprehensive DU solutions.
Review the PaperAll credit for this research goes to the researchers of this project. Also, don't forget to follow us on twitter.com/Marktechpost”>twitter.
Join our Telegram Channel and LinkedIn GrAbove!.
If you like our work, you will love our Newsletter..
Don't forget to join our Subreddit with over 46 billion users
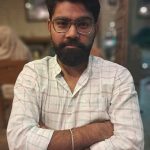
Nikhil is a Consultant Intern at Marktechpost. He is pursuing an integrated dual degree in Materials from Indian Institute of technology, Kharagpur. Nikhil is an ai and Machine Learning enthusiast who is always researching applications in fields like Biomaterials and Biomedical Science. With a strong background in Materials Science, he is exploring new advancements and creating opportunities to contribute.
<script async src="//platform.twitter.com/widgets.js” charset=”utf-8″>