artificial intelligence, particularly in training large multimodal models (LMMs), relies heavily on large datasets that include image and text sequences. These datasets enable the development of sophisticated models capable of understanding and generating multimodal content. As the capabilities of ai models advance, the need for large, high-quality datasets becomes even more critical, driving researchers to explore new methods of data collection and curation.
A major challenge in ai research is the need for large-scale, open-source, multimodal interleaved datasets. These datasets are essential for training models that seamlessly integrate text and image data. The limited availability of such datasets hinders the development of robust, high-performance open-source models, leading to a performance gap between open-source and proprietary models. Addressing this gap requires innovative approaches to building datasets that can provide the necessary scale and diversity.
Existing methods for creating multimodal datasets often involve collecting and curating data from HTML documents. Notable datasets such as OBELICS have been instrumental, but they are limited in scale and diversity as they source data primarily from HTML. This restriction impacts the variety and richness of the data, impacting the performance and applicability of the resulting ai models. Researchers have found that datasets sourced solely from HTML documents must capture the full spectrum of multimodal content required for comprehensive model training.
Researchers from the University of Washington, Salesforce Research, Stanford University, the University of Texas at Austin and the University of California at Berkeley presented Mint-1Tthe largest and most diverse open-source multimodal interleaved dataset to date, addressing the need for larger and more diverse datasets. MINT-1T comprises one trillion text tokens and 3.4 billion images from HTML, PDFs, and ArXiv papers. This dataset represents a tenfold increase over previous datasets, significantly improving the data for training multimodal models. Institutions such as the University of Washington and Salesforce Research collaborated on this initiative, demonstrating a concerted effort to close the gap in dataset availability.
The creation of the MINT-1T dataset involved a complex process of data acquisition, filtering, and deduplication. HTML documents were expanded to include data from previous years, and PDFs were processed to extract readable text and images. ArXiv articles were analyzed for figures and text, ensuring a comprehensive collection of multimodal content. Advanced filtering methods were employed to remove low-quality, non-English, or out-of-place content. Deduplication processes were also implemented to remove repetitive data, ensuring the quality and diversity of the dataset.
Experiments showed that machine learning models trained on the MINT-1T dataset matched and often outperformed models trained on previous leading datasets such as OBELICS. The inclusion of more diverse sources in MINT-1,T resulted in better generalization and performance on multiple benchmarks. In particular, the dataset significantly improved performance on tasks involving visual question answering and multimodal reasoning. The researchers found that models trained on MINT-1T performed better across multiple demonstrations, highlighting the effectiveness of the dataset.
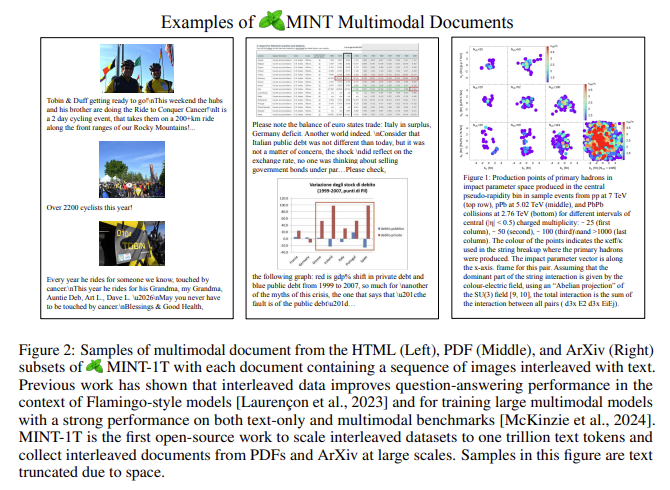
The construction of the MINT-1T dataset included detailed steps to ensure data quality and diversity. For example, the dataset consists of 922 billion HTML tokens, 106 billion PDF tokens, and 9 billion ArXiv tokens. The filtering process involved removing documents with inappropriate content and non-English texts, using tools such as Fasttext for language identification and NSFW detectors for image content. The deduplication process was crucial, as it involved Bloom filters to remove duplicate paragraphs and documents and hashing techniques to remove repeated images.
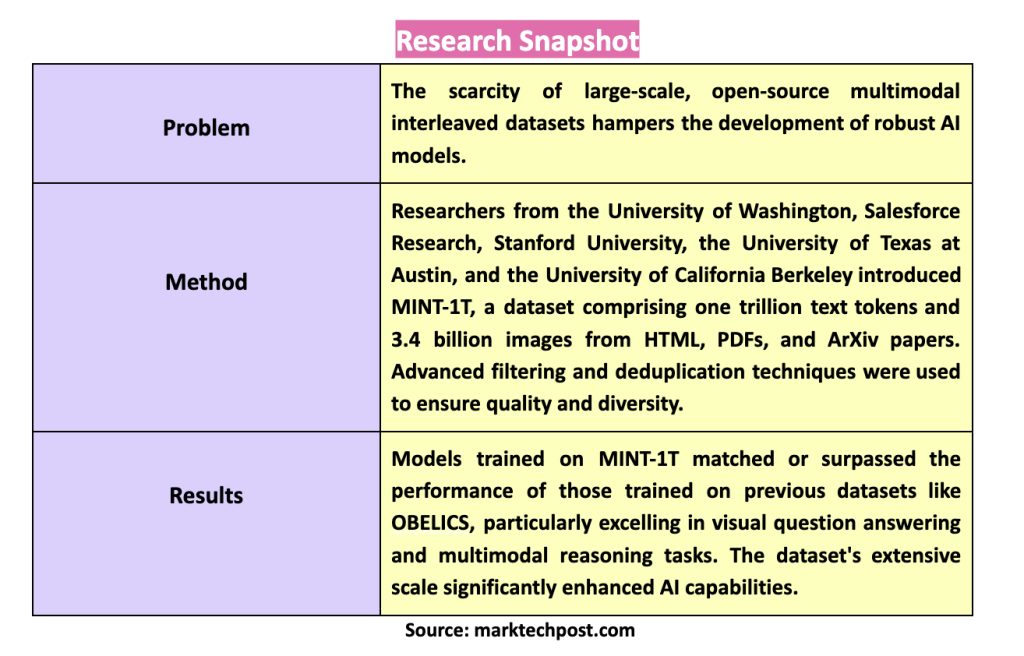
In conclusion, the MINT-1T dataset addresses the sparsity and diversity of datasets. By introducing a larger and more diverse dataset, researchers have enabled the development of more robust and high-performing open-source multimodal models. This work highlights the importance of data diversity and scale in ai research and paves the way for future improvements and applications in multimodal ai. The broad scale of the dataset, including one trillion text tokens and 3.4 billion images, provides a solid foundation for advancing ai capabilities.
Review the Paper, Detailsand GitHubAll credit for this research goes to the researchers of this project. Also, don't forget to follow us on twitter.com/Marktechpost”>twitter and join our Telegram Channel and LinkedIn GrAbove!. If you like our work, you will love our Newsletter..
Don't forget to join our Over 47,000 ML subscribers on Reddit
Find upcoming ai webinars here
<figure class="wp-block-embed is-type-rich is-provider-twitter wp-block-embed-twitter“/>
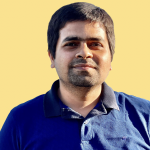
Asif Razzaq is the CEO of Marktechpost Media Inc. As a visionary engineer and entrepreneur, Asif is committed to harnessing the potential of ai for social good. His most recent initiative is the launch of an ai media platform, Marktechpost, which stands out for its in-depth coverage of machine learning and deep learning news that is technically sound and easily understandable to a wide audience. The platform has over 2 million monthly views, illustrating its popularity among the public.
<script async src="//platform.twitter.com/widgets.js” charset=”utf-8″>