Introduction
Meta has once again redefined the limits of artificial intelligence with the launch of the Segment Anything Model 2 (SAM-2). This groundbreaking advancement in computer vision takes the impressive capabilities of its predecessor, SAM, to the next level.
SAM-2 revolutionizes real-time image and video segmentation, precisely identifying and segmenting objects. This leap forward in visual understanding opens up new possibilities for ai applications across various industries, setting a new standard for what is achievable in computer vision.
Overview
- Meta’s SAM-2 advances computer vision with real-time image and video segmentation, building on its predecessor’s capabilities.
- SAM-2 enhances Meta ai’s models, extending from static image segmentation to dynamic video tasks with new features and improved performance.
- SAM-2 supports video segmentation, unifies architecture for image and video tasks, introduces memory components, and improves efficiency and occlusion handling.
- SAM-2 offers real-time video segmentation, zero-shot segmentation for new objects, user-guided refinement, occlusion prediction, and multiple mask predictions, excelling in benchmarks.
- SAM-2’s capabilities span video editing, augmented reality, surveillance, sports analytics, environmental monitoring, e-commerce, and autonomous vehicles.
- Despite advancements, SAM-2 faces challenges in temporal consistency, object disambiguation, fine detail preservation, and long-term memory tracking, indicating areas for future research.
In the rapidly evolving landscape of artificial intelligence and computer vision, Meta ai continues to push boundaries with its groundbreaking models. Building upon the revolutionary Segment Anything Model (SAM), which we explored in depth in our previous article “Meta’s Segment Anything Model: A Leap in Computer Vision,” Meta ai has now introduced SAM Meta 2, representing yet another significant leap forward in the image and video segmentation technology.
Our previous exploration delved into SAM’s innovative approach to image segmentation, its flexibility in responding to user prompts, and its potential to democratize advanced computer vision across various industries. SAM’s ability to generalize to new objects and situations without additional training and the release of the extensive Segment Anything Dataset (SA-1B) set a new standard in the field.
Now, with Meta SAM 2, we witness the evolution of this technology, extending its capabilities from static images to the dynamic world of video segmentation. This article builds upon our previous insights, examining how Meta SAM 2 not only enhances the foundational strengths of its predecessor but also introduces novel features that promise to reshape our interaction with visual data in motion.
Differences from the Original SAM
While SAM 2 builds upon the foundation laid by its predecessor, it introduces several significant improvements:
- Video Capability: Unlike SAM, which was limited to images, SAM 2 can segment objects in videos.
- Unified Architecture: SAM 2 uses a single model for both image and video tasks, whereas SAM is image-specific.
- Memory Mechanism: The introduction of memory components allows SAM 2 to track objects across video frames, a feature absent in the original SAM.
- Occlusion Handling: SAM 2’s occlusion head enables it to predict object visibility, a capability not present in SAM.
- Improved Efficiency: SAM 2 is six times faster than SAM in image segmentation tasks.
- Enhanced Performance: SAM 2 outperforms the original SAM on various benchmarks, even in image segmentation.
SAM-2 Features
Let’s understand the Features of this model:
- It can handle both image and video segmentation tasks within a single architecture.
- This model can segment objects in videos at approximately 44 frames per second.
- It can segment objects it has never encountered before, adapt to new visual domains without additional training, or perform zero-shot segmentation on the new images for objects different from its training.
- Users can refine the segmentation on selected pixels by providing prompts.
- The occlusion head facilitates the model in predicting whether an object is visible in a given time frame.
- SAM-2 outperforms existing models on various benchmarks for both image and video segmentation tasks
What’s New in SAM-2?
Here’s what SAM-2 has:
- Video Segmentation: the most important addition is the ability to segment objects in a video, following them across all frames and handling the occlusion.
- Memory Mechanism: this new version adds a memory encoder, a memory bank, and a memory attention module, which stores and uses the information of objects .it also helps in user interaction throughout the video.
- Streaming Architecture: This model processes the video frames one at a time, making it possible to segment long videos in real time.
- Multiple Mask Prediction: SAM 2 can provide several possible masks when the image or video is uncertain.
- Occlusion Prediction: This new feature helps the model to deal with the objects that are temporarily hidden or leave the frame.
- Improved Image Segmentation: SAM 2 is better at segmenting images than the original SAM. While it is superior in video tasks.
Demo and Web UI of SAM-2
Meta has also released a web-based demo to show SAM 2 capabilities where users can
- Upload the short videos or images
- Segment objects in real-time using points, boxes, or masks
- Refine Segmentation across video frames
- Apply video effects based on the model predictions
- Can add the background effect also to a segmented video
Here’s what the Demo page looks like, which gives plenty of options to choose from, pin the object to be traced, and apply different effects.
The Demo is a great tool for researchers and developers to explore SAM 2 potential and practical applications.
Original Video
We are tracing the ball here.
Segmented video
Research on the Model
Research and Development of Meta SAM 2
Model Architecture of Meta SAM 2
Meta SAM 2 expands on the original SAM model, generalizing its ability to handle images and videos. The architecture is designed to support various types of prompts (points, boxes, and masks) on individual video frames, enabling interactive segmentation across entire video sequences.
Key Components:
- Image Encoder: Utilizes a pre-trained Hiera model for efficient, real-time processing of video frames.
- Memory Attention: Conditions current frame features on past frame information and new prompts using transformer blocks with self-attention and cross-attention mechanisms.
- Prompt Encoder and Mask Decoder: Similar to SAM, but adapted for video context. The decoder can predict multiple masks for ambiguous prompts and includes a new head to detect object presence in frames.
- Memory Encoder: Generates compact representations of past predictions and frame embeddings.
- Memory Bank: This storage area stores information from recent frames and prompted frames, including spatial features and object pointers for semantic information.
Innovations:
- Streaming Approach: Processes video frames sequentially, allowing for real-time segmentation of arbitrary-length videos.
- Temporal Conditioning: Uses memory attention to incorporate information from past frames and prompts.
- Flexibility in Prompting: Allows for prompts on any video frame, enhancing interactive capabilities.
- Object Presence Detection: Addresses scenarios where the target object may not be present in all frames.
Training:
The model is trained on both image and video data, simulating interactive prompting scenarios. It uses sequences of 8 frames, with up to 2 frames randomly selected for prompting. This approach helps the model learn to handle various prompting situations and propagate segmentation across video frames effectively.
This architecture enables Meta SAM 2 to provide a more versatile and interactive experience for video segmentation tasks. It builds upon the strengths of the original SAM model while addressing the unique challenges of video data.
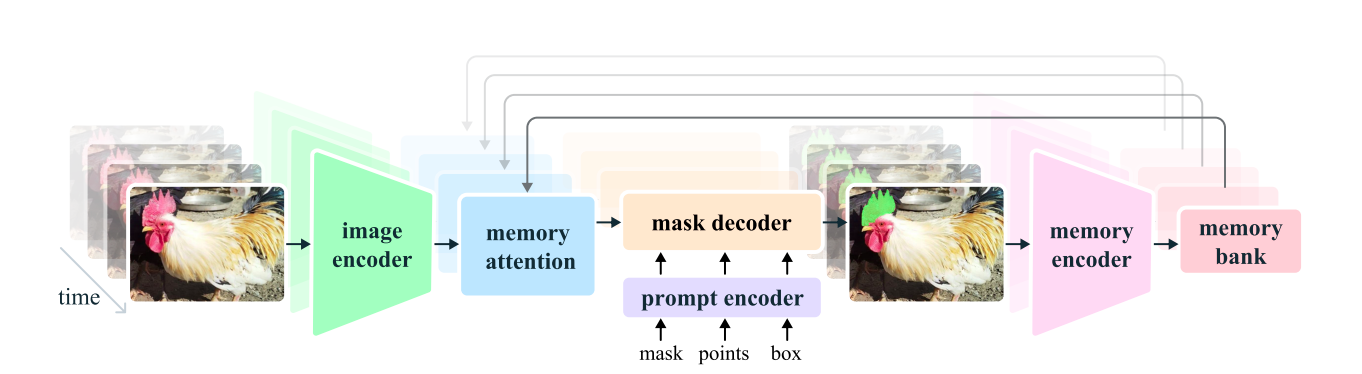
Promptable Visual Segmentation: Expanding SAM’s Capabilities to Video
Promptable Visual Segmentation (PVS) represents a significant evolution of the Segment Anything (SA) task, extending its capabilities from static images to the dynamic realm of video. This advancement allows for interactive segmentation across entire video sequences, maintaining the flexibility and responsiveness that made SAM revolutionary.
In the PVS framework, users can interact with any video frame using various prompt types, including clicks, boxes, or masks. The model then segments and tracks the specified object throughout the entire video. This interaction maintains the instantaneous response on the prompted frame, similar to SAM’s performance on static images, while also generating segmentations for the entire video in near real-time.
Key features of PVS include:
- Multi-frame Interaction: PVS allows prompts on any frame, unlike traditional video object segmentation tasks that typically rely on first-frame annotations.
- Diverse Prompt Types: Users can employ clicks, masks, or bounding boxes as prompts, enhancing flexibility.
- Real-time Performance: The model provides instant feedback on the prompted frame and swift segmentation across the entire video.
- Focus on Defined Objects: Similar to SAM, PVS targets objects with clear visual boundaries, excluding ambiguous regions.
PVS bridges several related tasks in both image and video domains:
- It encompasses the Segment Anything task for static images as a special case.
- It extends beyond traditional semi-supervised and interactive video object segmentation tasks, typically limited to specific prompts or first-frame annotations.
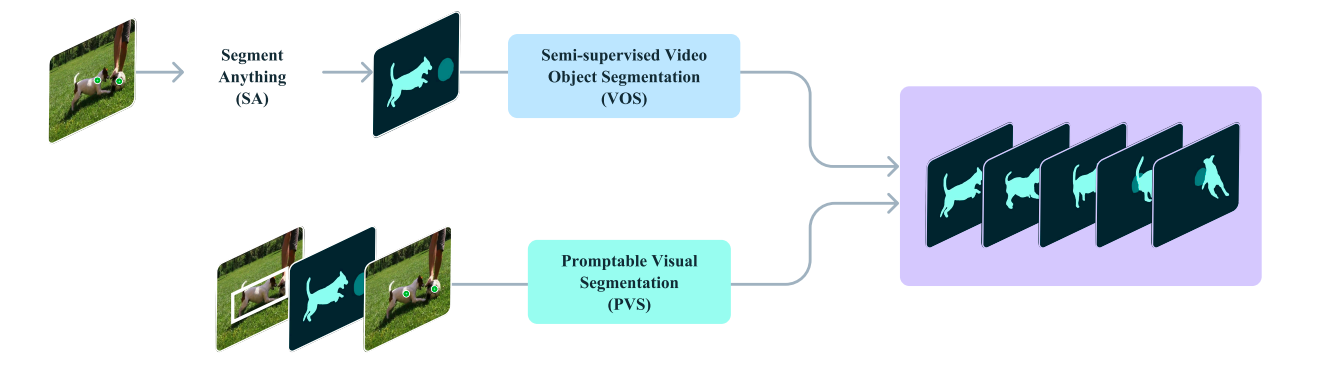
The evolution of Meta SAM 2 involved a three-phase research process, each phase bringing significant improvements in annotation efficiency and model capabilities:
1st Phase: Foundational Annotation with SAM
- Approach: Used image-based interactive SAM for frame-by-frame annotation
- Process: Annotators manually segmented objects at 6 FPS using SAM and editing tools
- Results:
- 16,000 masklets collected across 1,400 videos
- Average annotation time: 37.8 seconds per frame
- Produced high-quality spatial annotations but was time-intensive
2nd Phase: Introducing SAM 2 Mask
- Improvement: Integrated SAM 2 Mask for temporal mask propagation
- Process:
- Initial frame annotated with SAM
- SAM 2 Mask propagated annotations to subsequent frames
- Annotators refined predictions as needed
- Outcomes:
- 63,500 masklets collected
- Annotation time reduced to 7.4 seconds per frame (5.1x speed-up)
- The model was retrained twice during this phase
3rd Phase: Full Implementation of SAM 2
- Features: Unified model for interactive image segmentation and mask propagation
- Advancements:
- Accepts various prompt types (points, masks)
- Utilizes temporal memory for improved predictions
- Results:
- 197,000 masklets collected
- Annotation time was further reduced to 4.5 seconds per frame (8.4x speed-up from Phase 1)
- The model was retrained five times with newly collected data
Here’s a comparison between phases :

Key Improvements:
- Efficiency: Annotation time decreased from 37.8 to 4.5 seconds per frame across phases.
- Versatility: Evolved from frame-by-frame annotation to seamless video segmentation.
- Interactivity: Progressed to a system requiring only occasional refinement clicks.
- Model Enhancement: Continuous retraining with new data improved performance.
This phased approach showcases the iterative development of Meta SAM 2, highlighting significant advancements in both the model’s capabilities and the efficiency of the annotation process. The research demonstrates a clear progression towards a more robust, versatile, and user-friendly video segmentation tool.
The research paper demonstrates several significant advancements achieved by Meta SAM 2:
- Meta SAM 2 outperforms existing approaches across 17 zero-shot video datasets, requiring approximately 66% fewer human-in-the-loop interactions for interactive video segmentation.
- It surpasses the original SAM on its 23-dataset zero-shot benchmark suite while operating six times faster for image segmentation tasks.
- Meta SAM 2 excels on established video object segmentation benchmarks like DAVIS, MOSE, LVOS, and YouTube-VOS, setting new state-of-the-art standards.
- The model achieves an inference speed of approximately 44 frames per second, providing a real-time user experience. When used for video segmentation annotation, Meta SAM 2 is 8.4 times faster than manual per-frame annotation with the original SAM.
- To ensure equitable performance across diverse user groups, the researchers conducted fairness evaluations of Meta SAM 2:
The model shows minimal performance discrepancy in video segmentation across perceived gender groups.
These results underscore Meta SAM 2’s speed, accuracy, and versatility advancements across various segmentation tasks while demonstrating its consistent performance across different demographic groups. This combination of technical prowess and fairness considerations positions Meta SAM 2 as a significant step forward in visual segmentation.
The Segment Anything 2 model is built upon a robust and diverse dataset called SA-V (Segment Anything – Video). This dataset represents a significant advancement in computer vision, particularly for training general-purpose object segmentation models from open-world videos.
SA-V comprises an extensive collection of 51,000 diverse videos and 643,000 spatio-temporal segmentation masks called masklets. This large-scale dataset is designed to cater to a wide range of computer vision research applications operating under the permissive CC BY 4.0 license.
Key characteristics of the SA-V dataset include:
- Scale and Diversity: With 51,000 videos and an average of 12.61 masklets per video, SA-V offers a rich and varied data source. The videos cover various subjects, from locations and objects to complex scenes, ensuring comprehensive coverage of real-world scenarios.
- High-Quality Annotations: The dataset features a combination of human-generated and ai-assisted annotations. Out of the 643,000 masklets, 191,000 were created through SAM 2-assisted manual annotation, while 452,000 were automatically generated by SAM 2 and verified by human annotators.
- Class-Agnostic Approach: SA-V adopts a class-agnostic annotation strategy, focusing on mask annotations without specific class labels. This approach enhances the model’s versatility in segmenting various objects and scenes.
- High-Resolution Content: The average video resolution in the dataset is 1401×1037 pixels, providing detailed visual information for effective model training.
- Rigorous Validation: All 643,000 masklet annotations underwent review and validation by human annotators, ensuring high data quality and reliability.
- Flexible Format: The dataset provides masks in different formats to suit various needs – COCO run-length encoding (RLE) for the training set and PNG format for validation and test sets.
The creation of SA-V involved a meticulous data collection, annotation, and validation process. Videos were sourced through a contracted third-party company and carefully selected based on content relevance. The annotation process leveraged both the capabilities of the SAM 2 model and the expertise of human annotators, resulting in a dataset that balances efficiency with accuracy.
Here are example videos from the SA-V dataset with masklets overlaid (both manual and automatic). Each masklet is represented by a unique color, and each row displays frames from a single video, with a 1-second interval between frames:
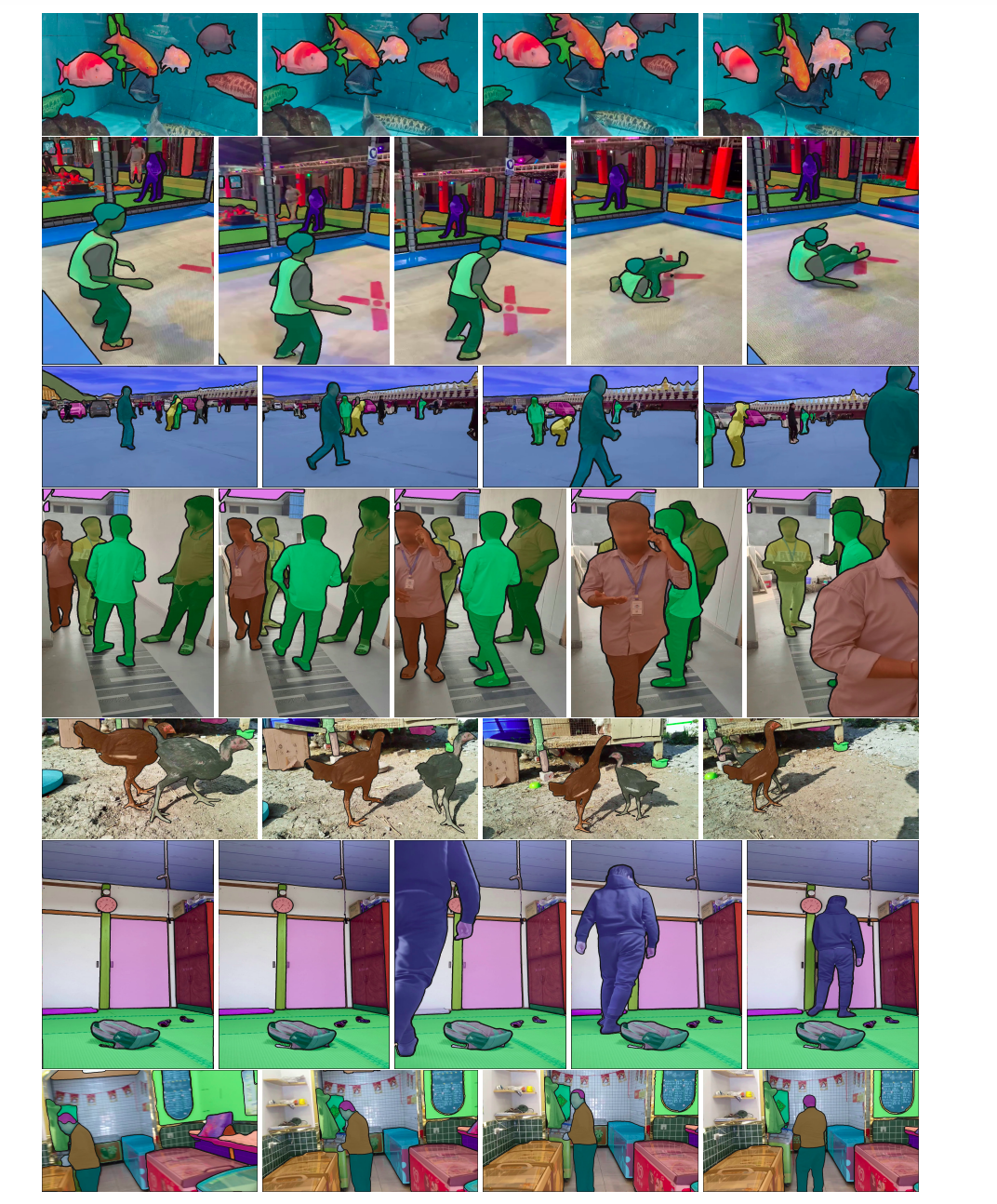
You can download the SA-V dataset directly from Meta ai. The dataset is available at the following link:
ai.meta.com/datasets/segment-anything-video-downloads” target=”_blank” rel=”noreferrer noopener nofollow”>Dataset Link
To access the dataset, you must provide certain information during the download process. This typically includes details about your intended use of the dataset and agreement to the terms of use. When downloading and using the dataset, it’s important to carefully read and comply with the licensing terms (CC BY 4.0) and usage guidelines provided by Meta ai.
While Meta SAM 2 represents a significant advancement in video segmentation technology, it’s important to acknowledge its current limitations and areas for future improvement:
1. Temporal Consistency
The model may struggle to maintain consistent object tracking in scenarios involving rapid scene changes or extended video sequences. For instance, Meta SAM 2 might lose track of a specific player during a fast-paced sports event with frequent camera angle shifts.
2. Object Disambiguation
The model can occasionally misidentify the target in complex environments with multiple similar objects. For example, a busy urban street scene might confuse different cars of the same model and color.
3. Fine Detail Preservation
Meta SAM 2 may not always capture intricate details accurately for objects in swift motion. This could be noticeable when trying to segment the individual feathers of a bird in flight.
4. Multi-Object Efficiency
While capable of segmenting multiple objects simultaneously, the model’s performance decreases as the number of tracked objects increases. This limitation becomes apparent in scenarios like crowd analysis or multi-character animation.
5. Long-term Memory
The model’s ability to remember and track objects over extended periods in longer videos is limited. This could pose challenges in applications like surveillance or long-form video editing.
6. Generalization to Unseen Objects
Meta SAM 2 may struggle with highly unusual or novel objects that significantly differ from its training data despite its broad training.
7. Interactive Refinement Dependency
In challenging cases, the model often relies on additional user prompts for accurate segmentation, which may not be ideal for fully automated applications.
8. Computational Resources
While faster than its predecessor, Meta SAM 2 still requires substantial computational power for real-time performance, potentially limiting its use in resource-constrained environments.
Future research directions could enhance temporal consistency, improve fine detail preservation in dynamic scenes, and develop more efficient multi-object tracking mechanisms. Additionally, exploring ways to reduce the need for manual intervention and expanding the model’s ability to generalize to a wider range of objects and scenarios would be valuable. As the field progresses, addressing these limitations will be crucial in realizing the full potential of ai-driven video segmentation technology.
The development of Meta SAM 2 opens up exciting possibilities for the future of ai and computer vision:
- Enhanced ai-Human Collaboration: As models like Meta SAM 2 become more sophisticated, we can expect to see more seamless collaboration between ai systems and human users in visual analysis tasks.
- Advancements in Autonomous Systems: The improved real-time segmentation capabilities could significantly enhance the perception systems of autonomous vehicles and robots, allowing for more accurate and efficient navigation and interaction with their environments.
- Evolution of Content Creation: The technology behind Meta SAM 2 could lead to more advanced tools for video editing and content creation, potentially transforming industries like film, television, and social media.
- Progress in Medical Imaging: Future iterations of this technology could revolutionize medical image analysis, enabling more accurate and faster diagnosis across various medical fields.
- Ethical ai Development: The fairness evaluations conducted on Meta SAM 2 set a precedent for considering demographic equity in ai model development, potentially influencing future ai research and development practices.
Meta SAM 2’s capabilities open up a wide range of potential applications across various industries:
- Video Editing and Post-Production: The model’s ability to efficiently segment objects in video could streamline editing processes, making complex tasks like object removal or replacement more accessible.
- Augmented Reality: Meta SAM 2’s real-time segmentation capabilities could enhance AR applications, allowing for more accurate and responsive object interactions in augmented environments.
- Surveillance and Security: The model’s ability to track and segment objects across video frames could improve security systems, enabling more sophisticated monitoring and threat detection.
- Sports Analytics: In sports broadcasting and analysis, Meta SAM 2 could track player movements, analyze game strategies, and create more engaging visual content for viewers.
- Environmental Monitoring: The model could be employed to track and analyze changes in landscapes, vegetation, or wildlife populations over time for ecological studies or urban planning.
- E-commerce and Virtual Try-Ons: The technology could enhance virtual try-on experiences in online shopping, allowing for more accurate and realistic product visualizations.
- Autonomous Vehicles: Meta SAM 2’s segmentation capabilities could improve object detection and scene understanding in self-driving car systems, potentially enhancing safety and navigation.
These applications showcase the versatility of Meta SAM 2 and highlight its potential to drive innovation across multiple sectors, from entertainment and commerce to scientific research and public safety.
Conclusion
Meta SAM 2 represents a significant leap forward in visual segmentation, building upon the foundation laid by its predecessor. This advanced model demonstrates remarkable versatility, handling both image and video segmentation tasks with increased efficiency and accuracy. Its ability to process video frames in real time while maintaining high-quality segmentation marks a new milestone in computer vision technology.
The model’s improved performance across various benchmarks, coupled with its reduced need for human intervention, showcases the potential of ai to revolutionize how we interact with and analyze visual data. While Meta SAM 2 is not without its limitations, such as challenges with rapid scene changes and fine detail preservation in dynamic scenarios, it sets a new standard for promptable visual segmentation. It paves the way for future advancements in the field.
Frequently Asked Questions
Ans. Meta SAM 2 is an advanced ai model for image and video segmentation. Unlike the original SAM, which was limited to images, SAM 2 can segment objects in both images and videos. It’s six times faster than SAM for image segmentation, can process videos at about 44 frames per second, and includes new features like a memory mechanism and occlusion prediction.
Ans. SAM 2’s key features include:
– Unified architecture for both image and video segmentation
– Real-time video segmentation capabilities
– Zero-shot segmentation for new objects
– User-guided refinement of segmentation
– Occlusion prediction
– Multiple mask prediction for uncertain cases
– Improved performance on various benchmarks
Ans. SAM 2 uses a streaming architecture to process video frames sequentially in real time. It incorporates a memory mechanism (including a memory encoder, memory bank, and memory attention module) to track objects across frames and handle occlusions. This allows it to segment and follow objects throughout a video, even when temporarily hidden or leaving the frame.
Ans. SAM 2 was trained on the SA-V (Segment Anything – Video) dataset. This dataset includes 51,000 diverse videos with 643,000 spatio-temporal segmentation masks (called masklets). The dataset combines human-generated and ai-assisted annotations, all validated by human annotators, and is available under a CC BY 4.0 license.