The integration of multimodal data, such as text, images, audio, and video, is a burgeoning field in ai, driving advances far beyond traditional unimodal models. Traditional ai has thrived in unimodal contexts, but the complexity of real-world data often intertwines these modes, presenting a substantial challenge. This complexity demands a model capable of seamlessly processing and integrating multiple types of data for a more holistic understanding.
To address this, the recent development of “Unified-IO 2” by researchers at the Allen Institute for ai, the University of Illinois Urbana-Champaign, and the University of Washington marks a monumental leap in ai capabilities. Unlike its predecessors, which were limited in handling dual modalities, Unified-IO 2 is an autoregressive multimodal model capable of interpreting and generating a wide range of data types, including text, images, audio, and video. It is the first of its kind, trained from scratch on a wide range of multimodal data. Its architecture is based on a single encoder-decoder transformer model, designed exclusively to convert varied inputs into a unified semantic space. This innovative approach allows the model to process different types of data together, overcoming the limitations of previous models.
The methodology behind Unified-IO 2 is as complex as it is innovative. It employs a shared representation space to encode multiple inputs and outputs, a feat accomplished by using byte-pair encoding for text and special tokens to encode sparse structures such as bounding boxes and keypoints. Images are encoded with a pre-trained Vision Transformer and a linear layer transforms these features into embeddings suitable for the transformer input. Audio data follows a similar path, being processed into spectrograms and encoded using an audio spectrogram transformer. The model also includes dynamic packing and a multimodal combination of denoising objectives, which improves its efficiency and effectiveness in handling multimodal signals.
Unified-IO 2's performance is as impressive as its design. Evaluated on over 35 data sets, it sets a new benchmark in GRIT evaluation, excelling at tasks such as keypoint estimation and surface normal estimation. It matches or outperforms many recently proposed vision-language models on vision and language tasks. Particularly notable is its imaging capabilities, where it outperforms its closest competitors in terms of fidelity to indications. The model also effectively generates audio from images or text, showing versatility despite its wide range of capabilities.
The conclusion drawn from the development and application of Unified-IO 2 is profound. It represents a significant advance in ai's ability to process and integrate multimodal data and opens up new possibilities for ai applications. Its success in understanding and generating multimodal results highlights the potential of ai to interpret complex real-world scenarios more effectively. This development marks a pivotal moment in ai and paves the way for more comprehensive and nuanced models in the future.
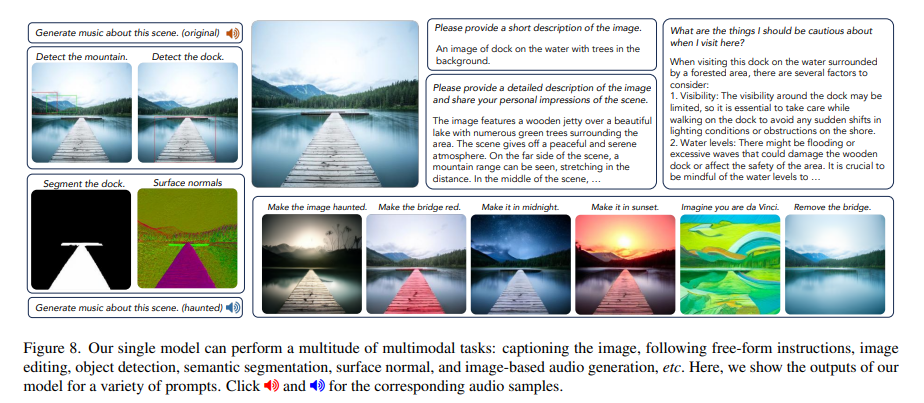
At its core, Unified-IO 2 serves as a beacon of ai's inherent potential, symbolizing a shift toward more integrative, versatile, and capable systems. Its success in navigating the complexities of multimodal data integration sets a precedent for future ai models, pointing toward a future where ai can more accurately reflect and interact with the multifaceted nature of the human experience.
Review the Paper, Projectand GitHub. All credit for this research goes to the researchers of this project. Also, don't forget to join. our SubReddit of more than 35,000 ml, 41k+ Facebook community, Discord channel, LinkedIn Graboveand Electronic newsletterwhere we share the latest news on ai research, interesting ai projects and more.
If you like our work, you'll love our newsletter.
Sana Hassan, a consulting intern at Marktechpost and a dual degree student at IIT Madras, is passionate about applying technology and artificial intelligence to address real-world challenges. With a strong interest in solving practical problems, she brings a new perspective to the intersection of ai and real-life solutions.
<!– ai CONTENT END 2 –>