A promising new development in artificial intelligence called MobileVLM has emerged, designed to maximize the potential of mobile devices. This cutting-edge Multimodal Vision Language Model (MMVLM) represents a significant advancement in bringing ai to mainstream technology as it is designed to work effectively in mobile situations.
Researchers from Meituan Inc., Zhejiang University, and Dalian University of technology spearheaded the creation of MobileVLM to address difficulties in integrating LLM with vision models for tasks such as visual question answering and image captioning, particularly in situations with limited resources. The traditional method of using large data sets created a barrier that hindered the development of text-to-video generation models. By using regulated and open source datasets, MobileVLM solves this problem and allows you to build high-performance models without being limited by large amounts of data.
MobileVLM architecture is a fusion of innovative design and practical application. It includes a visual encoder, a language model designed for peripheral devices, and an efficient projector. This projector is crucial for aligning graphic and text features and is designed to minimize computational costs while maintaining spatial information. The model significantly reduces the number of visual tokens, improving the speed of inference without compromising the quality of the output.
The MobileVLM training process consists of three key stages. Initially, the basic models of the language model are pretrained on a text-only data set. This is followed by supervised fine-tuning using multi-turn dialogues between humans and ChatGPT. The final stage involves training large vision models with multimodal datasets. This comprehensive training strategy ensures that MobileVLM is efficient and robust in its performance.
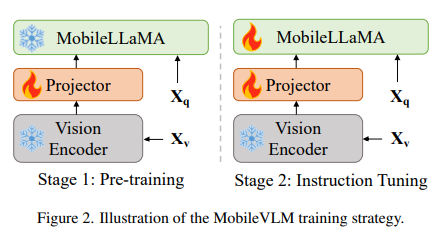
MobileVLM's performance in terms of language understanding and common sense reasoning is noteworthy. It competes favorably with existing models, demonstrating its effectiveness in reasoning and language processing tasks. MobileVLM's performance on several vision language model benchmarks underscores its potential. Despite its reduced parameters and reliance on limited training data, it achieves comparable results to larger, more resource-intensive models.
In conclusion, MobileVLM stands out for several reasons:
- It efficiently bridges the gap between large language and vision models, enabling advanced multimodal interactions on mobile devices.
- The innovative architecture, comprising an efficient projector and a custom language model, optimizes performance and speed.
- MobileVLM's training process, which involves pre-training, fine-tuning, and using multimodal datasets, contributes to its robustness and adaptability.
- It demonstrates competitive performance on several benchmarks, indicating its potential in real-world applications.
Review the Paper and GitHub. All credit for this research goes to the researchers of this project. Also, don't forget to join. our SubReddit of more than 35,000 ml, 41k+ Facebook community, Discord channel, LinkedIn Grabove, Twitterand Electronic newsletterwhere we share the latest news on ai research, interesting ai projects and more.
If you like our work, you'll love our newsletter.
Hello, my name is Adnan Hassan. I'm a consulting intern at Marktechpost and soon to be a management trainee at American Express. I am currently pursuing a double degree from the Indian Institute of technology, Kharagpur. I am passionate about technology and I want to create new products that make a difference.
<!– ai CONTENT END 2 –>