Multilingual applications and multilingual tasks are critical to natural language processing (NLP) today, making robust integration models essential. These models underpin systems such as augmented recovery generation and other ai-powered solutions. However, existing models often struggle with noisy training data, limited domain diversity, and inefficiencies in handling multilingual data sets. These limitations affect performance and scalability. Researchers at Harbin Institute of technology (Shenzhen) have addressed these challenges with KaLM-Embedding, a model that emphasizes data quality and innovative training methodologies.
KaLM-Embedding is a multilingual embedding model built on Qwen 2-0.5B and released under the MIT license.. Designed with compactness and efficiency in mind, it is particularly suitable for real-world applications where computational resources are limited.
The data-centric design of the model is a key strength. It incorporates 550,000 synthetic data samples generated using human-based techniques to ensure diversity and relevance. Additionally, it employs classification consistency filtering to remove noisy and false negative samples, improving the quality and robustness of the training data.
Technical characteristics and advantages
KaLM-Embedding incorporates advanced methodologies to deliver powerful multilingual text embeddings. A notable feature is Matryoshka Representation Learning, which supports flexible embedding dimensions. This adaptability allows embeddings to be optimized for different applications, ranging from 64 to 896 dimensions.
The training strategy consists of two stages: weakly supervised pretraining and supervised fine tuning. More than 70 diverse data sets were used during tuning, spanning a variety of languages and domains. Semi-homogeneous task batching further refined the training process by balancing the challenges posed by negatives in the batch with the risk of false negatives.
KaLM-Embedding also benefits from its foundation on Qwen 2-0.5B, a pre-trained autoregressive language model. This architecture allows effective adaptation to integration tasks, offering an advantage over traditional BERT-type models.
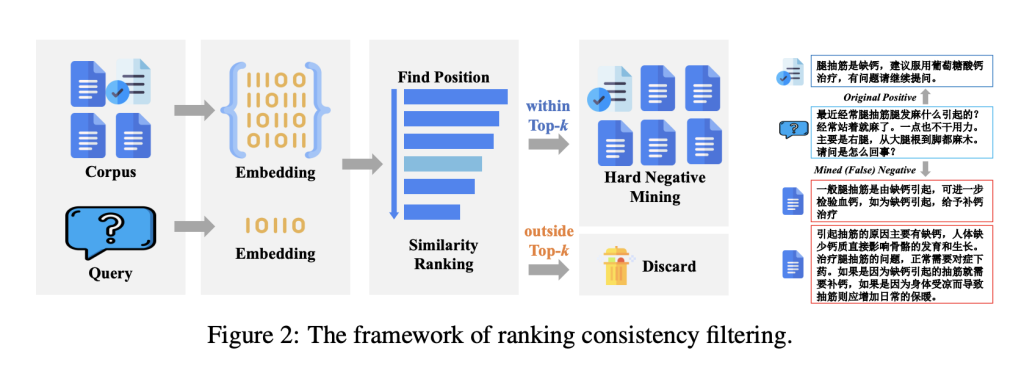
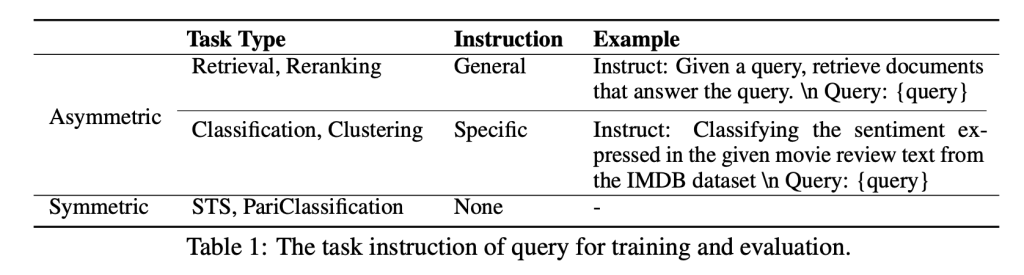
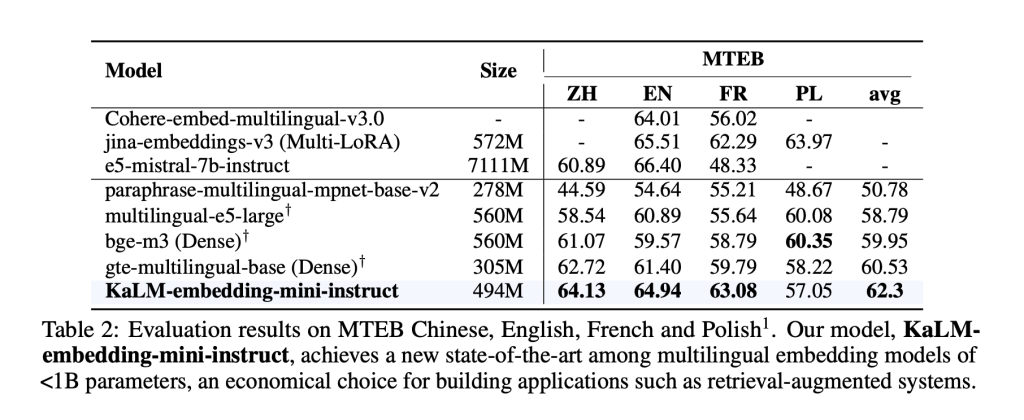
Performance and benchmark results
The performance of KaLM-Embedding was evaluated on the Massive Text Embedding Benchmark (MTEB). It achieved an average score of 64.53, setting a high standard for models with fewer than one billion parameters. The scores of 64.13 in Chinese-MTEB and 64.94 in English-MTEB highlight its multilingual capabilities. Despite limited fit data for some languages, the model demonstrated strong generalization capabilities.
Ablation studies provided additional information. Features such as Matryoshka representation learning and classification consistency filtering were shown to improve performance. However, the studies also highlighted areas for improvement, such as refining low-dimensional embeddings to further increase efficiency.
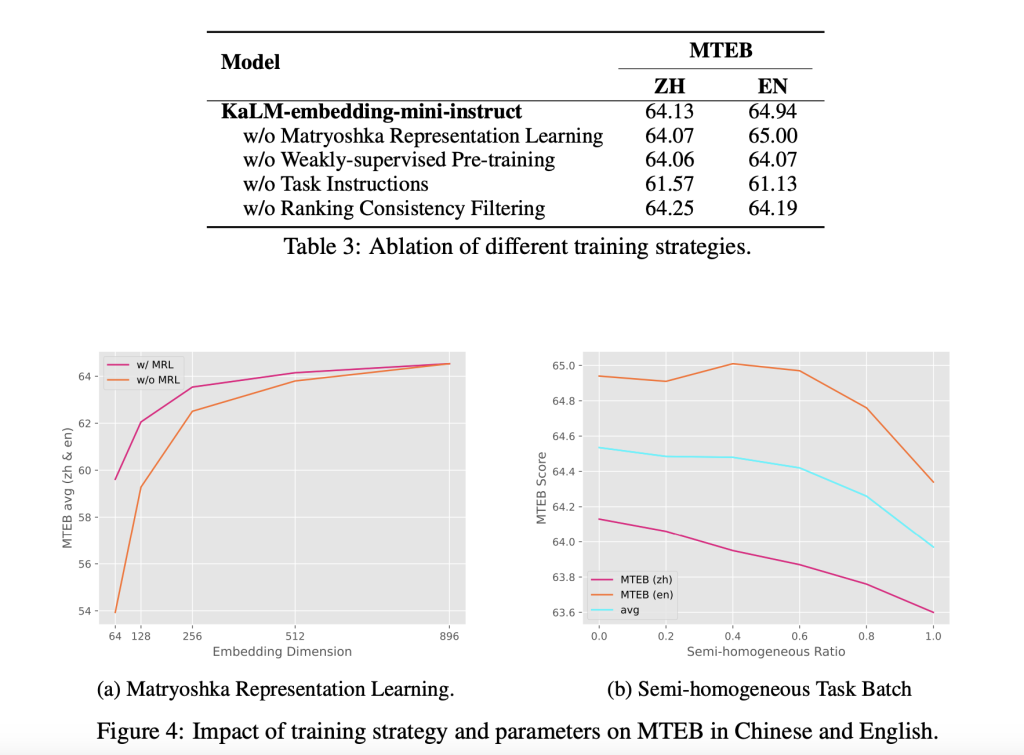
Conclusion: a step forward in multilingual incorporations
KaLM-Embedding represents a significant advance in multilingual embedding models. By addressing challenges such as noisy data and inflexible architectures, it strikes a balance between efficiency and performance. The open source version under the MIT license invites researchers and practitioners to explore and develop this work.
With its strong multilingual performance and innovative methodologies, KaLM-Embedding is well positioned for diverse applications, from augmented retrieval systems to multilingual tasks. As the need for multilingual NLP solutions continues to grow, KaLM-Embedding serves as a testament to the impact of high-quality data and thoughtful model design.
Verify he Paper, Modelsand Code. All credit for this research goes to the researchers of this project. Also, don't forget to follow us on <a target="_blank" href="https://x.com/intent/follow?screen_name=marktechpost” target=”_blank” rel=”noreferrer noopener”>twitter and join our Telegram channel and LinkedIn Grabove. Don't forget to join our SubReddit over 60,000 ml.
UPCOMING FREE ai WEBINAR (JANUARY 15, 2025): <a target="_blank" href="https://info.gretel.ai/boost-llm-accuracy-with-sd-and-evaluation-intelligence?utm_source=marktechpost&utm_medium=newsletter&utm_campaign=202501_gretel_galileo_webinar”>Increase LLM Accuracy with Synthetic Data and Assessment Intelligence–<a target="_blank" href="https://info.gretel.ai/boost-llm-accuracy-with-sd-and-evaluation-intelligence?utm_source=marktechpost&utm_medium=newsletter&utm_campaign=202501_gretel_galileo_webinar”>Join this webinar to learn practical information to improve LLM model performance and accuracy while protecting data privacy..
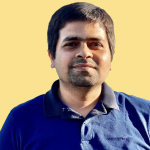
Asif Razzaq is the CEO of Marktechpost Media Inc.. As a visionary entrepreneur and engineer, Asif is committed to harnessing the potential of artificial intelligence for social good. Their most recent endeavor is the launch of an ai media platform, Marktechpost, which stands out for its in-depth coverage of machine learning and deep learning news that is technically sound and easily understandable to a wide audience. The platform has more than 2 million monthly visits, which illustrates its popularity among the public.