Machine learning has made notable advances, particularly in generative models such as diffusion models. These models are designed to handle high-dimensional data, including images and audio. Its applications cover various areas, such as art creation and medical imaging, demonstrating its versatility. The main goal has been to improve these models to better align them with human preferences, ensuring that their results are useful and safe for broader applications.
Despite significant advances, current generative models often need help to align perfectly with human preferences. This misalignment can lead to unhelpful or potentially harmful results. The critical issue is to tune these models to consistently produce desirable and safe results without compromising their generative capabilities.
Existing research includes reinforcement learning techniques and preference optimization strategies, such as Diffusion-DPO and SFT. Methods such as proximal policy optimization (PPO) and models such as stable diffusion XL (SDXL) have been used. Additionally, frameworks such as Kahneman-Tversky Optimization (KTO) have been adapted for text-to-image diffusion models. While these approaches improve alignment with human preferences, they often fail to handle various stylistic discrepancies or efficiently manage memory and computational resources.
Researchers from Korea Advanced Institute of Science and technology (KAIST), Korea University, and Hugging Face have introduced a novel method called Maximizing Alignment Preference Optimization (MaPO). This method aims to tune diffusion models more effectively by integrating preference data directly into the training process. The research team conducted extensive experiments to validate their approach, ensuring that it outperforms existing methods in terms of alignment and efficiency.
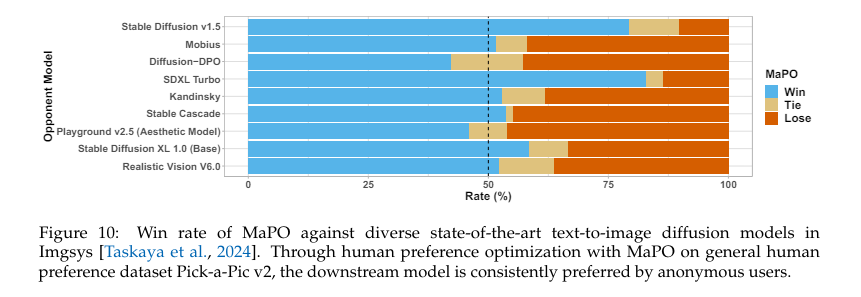
MaPO improves diffusion models by incorporating a preference data set during training. This data set includes several human preferences that the model must align with, such as safety and stylistic choices. The method involves a unique loss function that prioritizes preferred outcomes and penalizes less desirable ones. This tuning process ensures that the model generates results that closely align with human expectations, making it a versatile tool across different domains. The methodology used by MaPO is not based on any reference model, which differentiates it from traditional methods. By maximizing the probability margin between preferred and non-preferred image sets, MaPO learns general stylistic features and preferences without overfitting the training data. This makes the method efficient and memory friendly, suitable for various applications.
The performance of MaPO has been evaluated against several benchmarks. It demonstrated superior alignment with human preferences, achieving higher scores for safety and stylistic adherence. MaPO obtained a score of 6.17 in the Aesthetics benchmark and reduced training time by 14.5%, highlighting its efficiency. Additionally, the method outperformed the Stable Diffusion XL (SDXL) baseline and other existing methods, demonstrating its effectiveness in consistently generating preferred results.
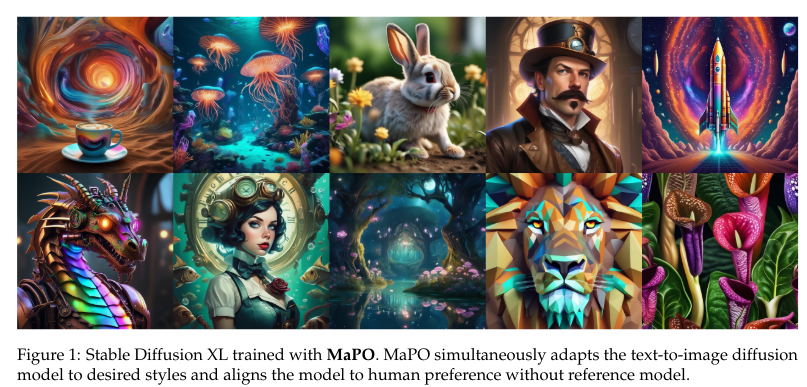
The MaPO method represents a significant advance in aligning generative models with human preferences. Researchers have developed a more efficient and effective solution by integrating preference data directly into the training process. This method improves the security and usefulness of model results and sets a new standard for future developments in this field.
Overall, the research highlights the importance of optimizing direct preferences in generative models. MaPO's ability to handle reference discrepancies and adapt to various stylistic preferences makes it a valuable tool for a variety of applications. The study opens new avenues for further exploration in preference optimization, paving the way for more personalized and secure generative models in the future.
Review the Paper. All credit for this research goes to the researchers of this project. Also, don't forget to follow us on twitter.com/Marktechpost”>twitter.
Join our Telegram channel and LinkedIn Grabove.
If you like our work, you will love our Newsletter..
Don't forget to join our SubReddit over 45,000ml
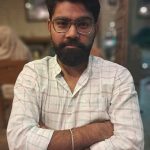
Nikhil is an internal consultant at Marktechpost. He is pursuing an integrated double degree in Materials at the Indian Institute of technology Kharagpur. Nikhil is an ai/ML enthusiast who is always researching applications in fields like biomaterials and biomedical science. With a strong background in materials science, he is exploring new advances and creating opportunities to contribute.
<script async src="//platform.twitter.com/widgets.js” charset=”utf-8″>