Authorship verification (AV) is critical in natural language processing (NLP) as it determines whether two texts share the same authorship. This task holds immense importance in various domains such as forensics, literature, and digital security. The traditional AV approach relied heavily on stylometric analysis, which uses linguistic and stylistic features such as word and sentence lengths and function word frequencies to differentiate between authors. With deep learning models such as BERT and RoBERTa, the field has undergone a paradigm shift. These modern approaches leverage complex patterns in text and deliver superior performance compared to conventional stylometric techniques.
The main challenge in authorship verification is to accurately determine authorship and provide clear and reliable explanations for classification decisions. Current authorship verification models mainly focus on binary classification, which often lacks transparency. This lack of explainability is a gap in academic interest and a practical concern. Analyzing the decision-making process of ai models is essential to build trust and reliability, in particular to identify and address hidden biases. Therefore, authorship verification models must be accurate and interpretable, and provide detailed insights into their decision-making processes.
Existing methods for vehicle automation have made significant progress with the use of deep learning models. BERT and RoBERTa, for example, have demonstrated superior performance over traditional stylometric techniques. However, these models often need to provide clear explanations for their classifications. This is a critical limitation as the demand for explainable ai increases. Recent advances have explored integrating explainability into these models, but challenges remain in ensuring that explanations are consistent and relevant across various scenarios.
The Information Systems technology and Design research team at the Singapore University of technology and Design introduced a novel approach called InstructAVInstructAV is a new framework that aims to improve accuracy and explainability in authorship verification tasks. InstructAV uses Large Language Models (LLMs) with an Efficient Parameter Fine-Tuning (PEFT) method. This innovative framework is designed to align classification decisions with transparent and understandable explanations, marking a significant advancement in the field. The InstructAV framework integrates explainability directly into the classification process, ensuring that models make accurate predictions and provide deep insights into their decision-making logic. This dual capability is essential to advancing explainable ai.
The methodology behind InstructAV involves three main steps: data collection, consistency checking, and fine-tuning using the Low-Rank Adaptation (LoRA) method. Initially, the framework focuses on aggregating explanatory data for AV samples. This approach uses the binary classification labels available in existing AV datasets. A strict quality control is then implemented to verify the alignment and consistency of the explanations with the corresponding classification labels. The final stage involves the synthesis of instruction-tuning data, which combines the collected classification labels and their associated explanations. This composite data is the basis for fine-tuning LLMs using the LoRA adaptation technique. It ensures that the models are fine-tuned for the AV tasks while improving their ability to provide consistent and reliable explanations.
InstructAV’s performance was evaluated through extensive experiments on various AV datasets including IMDB, twitter, and Yelp Reviews. The framework demonstrated state-of-the-art accuracy in authorship verification, significantly outperforming baseline models. For example, InstructAV with LLaMA-2-7B achieved 91.4% accuracy on the IMDB dataset, a substantial improvement over the top-performing baseline, BERT, which scored 67.7%. InstructAV achieved high classification accuracy and set new benchmarks in generating coherent and reasoned explanations for its findings. The ROUGE-1 and ROUGE-2 scores highlighted InstructAV’s superior performance in achieving content overlap at both the word and phrase levels. The BERT score indicated that the explanations generated by InstructAV were semantically closer to the explanation labels, underscoring the framework’s ability to produce linguistically coherent and contextually relevant explanations.
In conclusion, the InstructAV framework addresses critical challenges in autonomous vehicle tasks by combining high classification accuracy with the ability to generate detailed and reliable explanations. The dual focus on performance and interpretability positions InstructAV as a state-of-the-art solution in the domain. The research team has made several key contributions, including developing the InstructAV framework, creating three instruction-tuning datasets with reliable linguistic explanations, and demonstrating the effectiveness of the framework through both automated and human evaluations. InstructAV’s ability to improve classification accuracy while providing high-quality explanations marks crucial progress in autonomous vehicle research.
Review the PaperAll credit for this research goes to the researchers of this project. Also, don't forget to follow us on twitter.com/Marktechpost”>twitter and join our Telegram Channel and LinkedIn GrAbove!. If you like our work, you will love our Newsletter..
Don't forget to join our Subreddit with over 46 billion users
Find upcoming ai webinars here
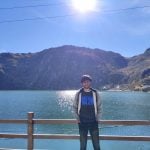
Aswin AK is a Consulting Intern at MarkTechPost. He is pursuing his dual degree from Indian Institute of technology, Kharagpur. He is passionate about Data Science and Machine Learning and has a strong academic background and practical experience in solving real-world interdisciplinary challenges.
<script async src="//platform.twitter.com/widgets.js” charset=”utf-8″>