The use of LLM in clinical diagnosis offers a promising way to improve doctor-patient interactions. The patient's history is essential for medical diagnosis. However, factors such as increasing patient numbers, limited access to care, short consultations, and the rapid adoption of telemedicine, accelerated by the COVID-19 pandemic, have challenged this traditional practice. These challenges threaten diagnostic accuracy and underscore the need for solutions that improve the quality of clinical conversations.
Generative ai, particularly LLMs, can address this problem through rich, interactive conversations. They have the potential to collect comprehensive patient histories, assist with differential diagnoses, and support clinicians in telehealth and emergency settings. However, its readiness for the real world remains untested. While current assessments focus on multiple-choice medical questions, there is limited exploration of LLMs' capacity for interactive patient communication. This gap highlights the need to evaluate its effectiveness in improving virtual medical visits, triage, and medical education.
Researchers at Harvard Medical School, Stanford University, MedStar Georgetown University, Northwestern University, and other institutions developed the Conversational Reasoning Assessment Framework for Testing in Medicine (CRAFT-MD). This framework evaluates clinical LLMs such as GPT-4 and GPT-3.5 through simulated doctor-patient conversations, focusing on diagnostic accuracy, history-taking, and reasoning. Addresses limitations of current models and offers recommendations for more effective and ethical LLM assessments in healthcare.
The study evaluated multimodal and text-only LLMs using medical case vignettes. The text-based models were evaluated with 2000 questions from the MedQA-USMLE dataset, which included several medical specialties and additional dermatology questions. The NEJM Image Challenge dataset, consisting of image and vignette pairs, was used for multi-modal electric vehicles. MELD analysis was used to identify possible contamination of the data set by comparing the model responses to the test questions. An ai evaluator and medical experts evaluated clinical LLMs interacting with simulated patient ai agents and their diagnostic accuracy. Different conversational formats and multiple choice questions were used to evaluate the performance of the model.
The CRAFT-MD framework assesses clinical LLMs' conversational reasoning during simulated doctor-patient interactions. It includes four components: the clinical LLM, an ai agent for the patient, an ai agent for the classifier, and medical experts. The framework tests the LLM's ability to ask relevant questions, synthesize information, and provide accurate diagnoses. A conversational summarization technique was developed, which transforms multi-turn conversations into concise summaries and improves model accuracy. The study found that accuracy decreased significantly when moving from multiple-choice to free-response questions, and conversational interactions generally underperformed compared to vignette-based tasks, highlighting the challenges of open-ended clinical reasoning.
Despite demonstrating proficiency in medical tasks, clinical LLMs are often assessed using static assessments, such as multiple choice questions (MCQs), which fail to capture the complexity of real-world clinical interactions. Using the CRAFT-MD framework, the evaluation found that LLMs performed significantly worse in conversational settings than structured exams. We recommend moving to more realistic tests, such as dynamic doctor-patient conversations, open-ended questions, and a complete medical history to better reflect clinical practice. Furthermore, multimodal data integration, continuous evaluation, and improvement of rapid strategies are crucial to advancing LLMs as reliable diagnostic tools, ensuring scalability, and reducing bias in diverse populations.
Verify he Paper. All credit for this research goes to the researchers of this project. Also, don't forget to follow us on <a target="_blank" href="https://twitter.com/Marktechpost”>twitter and join our Telegram channel and LinkedIn Grabove. Don't forget to join our SubReddit over 60,000 ml.
UPCOMING FREE ai WEBINAR (JANUARY 15, 2025): <a target="_blank" href="https://info.gretel.ai/boost-llm-accuracy-with-sd-and-evaluation-intelligence?utm_source=marktechpost&utm_medium=newsletter&utm_campaign=202501_gretel_galileo_webinar”>Increase LLM Accuracy with Synthetic Data and Assessment Intelligence–<a target="_blank" href="https://info.gretel.ai/boost-llm-accuracy-with-sd-and-evaluation-intelligence?utm_source=marktechpost&utm_medium=newsletter&utm_campaign=202501_gretel_galileo_webinar”>Join this webinar to learn practical information to improve LLM model performance and accuracy while protecting data privacy..
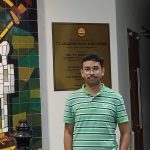
Sana Hassan, a consulting intern at Marktechpost and a dual degree student at IIT Madras, is passionate about applying technology and artificial intelligence to address real-world challenges. With a strong interest in solving practical problems, he brings a new perspective to the intersection of ai and real-life solutions.
<script async src="//platform.twitter.com/widgets.js” charset=”utf-8″>