Technological advances have been instrumental in transcending the limits of what can be achieved in the realm of audio generation, especially in high-fidelity audio synthesis. As demand for more sophisticated and realistic audio experiences increases, researchers have been driven to innovate beyond conventional methods to solve persistent challenges within this field.
A major issue that has hindered progress is the generation of high-quality music and singing voices, where existing models often struggle with spectral discontinuities and the need for greater clarity at higher frequencies. These obstacles have prevented the production of clear, realistic audio, indicating a gap in current technological capabilities.
Current advances have largely focused on generative adversarial networks (GANs) and neural vocoders, which have revolutionized audio synthesis thanks to their ability to efficiently generate waveforms from acoustic properties. However, these models, including state-of-the-art vocoders such as HiFiGAN and BigVGAN, have encountered limitations such as inadequate data diversity, limited model capacity, and scaling challenges, particularly in the high-fidelity audio domain.
A research team has introduced improved variegated audio generation via scalable generative adversarial networks (EVA-GAN). This model takes advantage of an extensive data set of 36,000 hours of high-fidelity audio and incorporates a novel contextual module, which goes further in spectral and high-frequency reconstruction. By expanding the model to approximately 200 million parameters, EVA-GAN marks a major advance in audio synthesis technology.
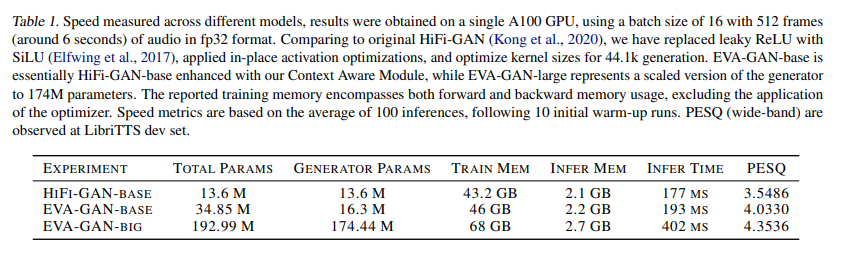
The main innovation of EVA-GAN lies in its Context Aware module (CAM) and a Human-In-The-Loop artifact measurement toolkit designed to improve model performance with minimal additional computational cost. CAM leverages residual connections and large convolution kernels to increase the context window and capacity of the model, addressing spectral discontinuity and blurring in the generated audio. This is complemented by the Human-In-The-Loop toolkit, which ensures alignment of generated audio with human perception standards, marking a significant step towards closing the gap between artificial audio generation and human perception. natural sound.
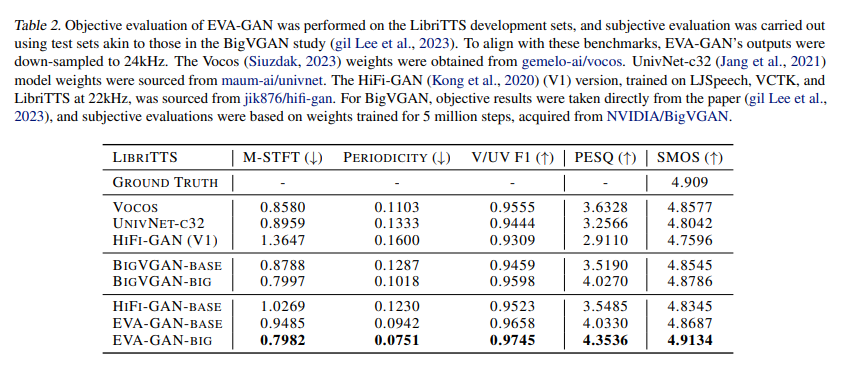
Performance evaluations of EVA-GAN have demonstrated its superior capabilities, particularly in high-fidelity audio generation. The model outperforms existing next-generation solutions in robustness and quality, especially in out-of-domain data performance, setting a new benchmark in the field. For example, EVA-GAN achieves a Perceptual Evaluation of Speech Quality (PESQ) score of 4.3536 and a Similarity Mean Option Score (SMOS) of 4.9134, significantly outperforming its predecessors and demonstrating its ability to replicate the richness and clarity of natural sound.
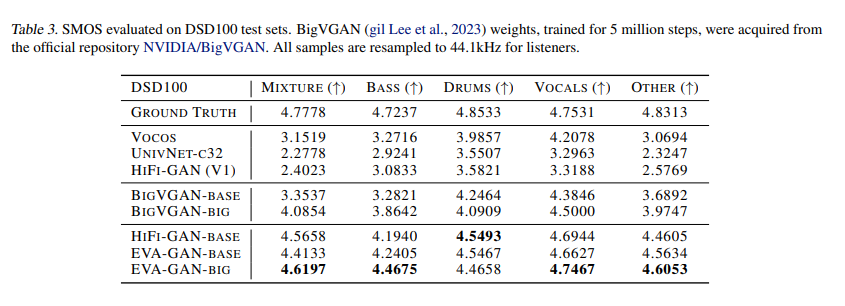
In conclusion, EVA-GAN represents a monumental step in audio generation technology. By overcoming the long-standing challenges of spectral discontinuities and blurring in high-frequency domains, it sets a new standard for high-quality audio synthesis. This innovation enriches the audio experience for end users. It opens new avenues for research and development in speech synthesis, music generation and more, heralding a new era of audio technology where the boundaries of realism are continually expanded.
Review the Paper. All credit for this research goes to the researchers of this project. Also, don't forget to follow us on Twitter and Google news. Join our 36k+ ML SubReddit, 41k+ Facebook community, Discord channeland LinkedIn Grabove.
If you like our work, you will love our Newsletter..
Don't forget to join our Telegram channel
Nikhil is an internal consultant at Marktechpost. He is pursuing an integrated double degree in Materials at the Indian Institute of technology Kharagpur. Nikhil is an ai/ML enthusiast who is always researching applications in fields like biomaterials and biomedical science. With a strong background in materials science, he is exploring new advances and creating opportunities to contribute.
<!– ai CONTENT END 2 –>