Jamba 1.5 is an instruction-tuned large language model that comes in two versions: Jamba 1.5 Large with 94 billion active parameters and Jamba 1.5 Mini with 12 billion active parameters. It combines the Mamba Structured State Space Model (SSM) with the traditional Transformer architecture. This model, developed by AI21 Labs, can process a 256K effective context window, which is the largest among open-source models.
Overview
- Jamba 1.5 a hybrid Mamba-Transformer model for efficient NLP, capable of processing massive context windows with up to 256K tokens.
- Its 94B and 12B parameter versions enable diverse language tasks while optimizing memory and speed through the ExpertsInt8 quantization.
- AI21’s Jamba 1.5 combines scalability and accessibility, supporting tasks from summarization to question-answering across nine languages.
- It’s innovative architecture allows for long-context handling and high efficiency, making it ideal for memory-heavy NLP applications.
- It’s hybrid model architecture and high-throughput design offer versatile NLP capabilities, available through API access and on Hugging Face.
What are Jamba 1.5 Models?
The Jamba 1.5 models, including Mini and Large variants, are designed to handle various natural language processing (NLP) tasks such as question answering, summarization, text generation, and classification. Jamba models on an extensive corpus support nine languages—English, Spanish, French, Portuguese, Italian, Dutch, German, Arabic, and Hebrew. Jamba 1.5, with its joint SSM-Transformer structure, tackles the problems with the conventional transformer models that are often hindered by two major limitations: high memory requirements for long context windows and slower processing.
The Architecture of Jamba 1.5
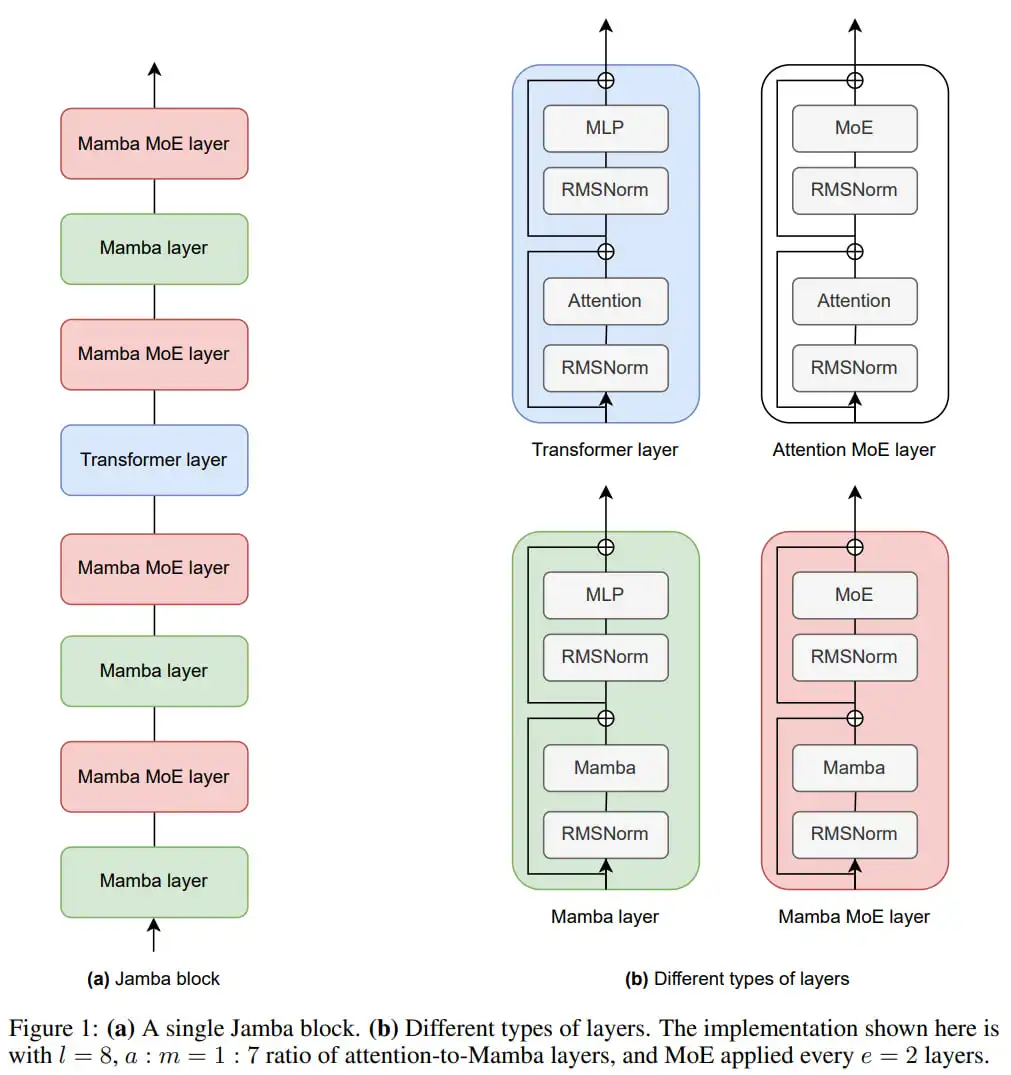
Aspect | Details |
Base Architecture | Hybrid Transformer-Mamba architecture with a Mixture-of-Experts (MoE) module |
Model Variants | Jamba-1.5-Large (94B active parameters, 398B total) and Jamba-1.5-Mini (12B active parameters, 52B total) |
Layer Composition | 9 blocks, each with 8 layers; 1:7 ratio of Transformer attention layers to Mamba layers |
Mixture of Experts (MoE) | 16 experts, selecting the top 2 per token for dynamic specialization |
Hidden Dimensions | 8192 hidden state size |
Attention Heads | 64 query heads, 8 key-value heads |
Context Length | Supports up to 256K tokens, optimized for memory with significantly reduced KV cache memory |
Quantization Technique | ExpertsInt8 for MoE and MLP layers, allowing efficient use of INT8 while maintaining high throughput |
Activation Function | Integration of Transformer and Mamba activations, with an auxiliary loss to stabilize activation magnitudes |
Efficiency | Designed for high throughput and low latency, optimized to run on 8x80GB GPUs with 256K context support |
Explanation
- KV cache memory is memory allocated for storing key-value pairs from previous tokens, optimizing speed when handling long sequences.
- ExpertsInt8 quantization is a compression method using INT8 precision in MoE and MLP layers to save memory and improve processing speed.
- Attention heads are separate mechanisms within the attention layer that focus on different parts of the input sequence, improving model understanding.
- Mixture-of-Experts (MoE) is a modular approach where only selected expert sub-models process each input, boosting efficiency and specialization.
Intended Use and Accessibility
Jamba 1.5 was designed for a range of applications accessible via AI21’s Studio API, Hugging Face or cloud partners, making it deployable in various environments. For tasks such as sentiment analysis, summarization, paraphrasing, and more. It can also be finetuned on domain-specific data for better results; the model can be downloaded from Hugging Face.
Jamba 1.5
One way to access them is by using AI21’s Chat interface:
Chat Interface
Here’s the link: Chat Interface
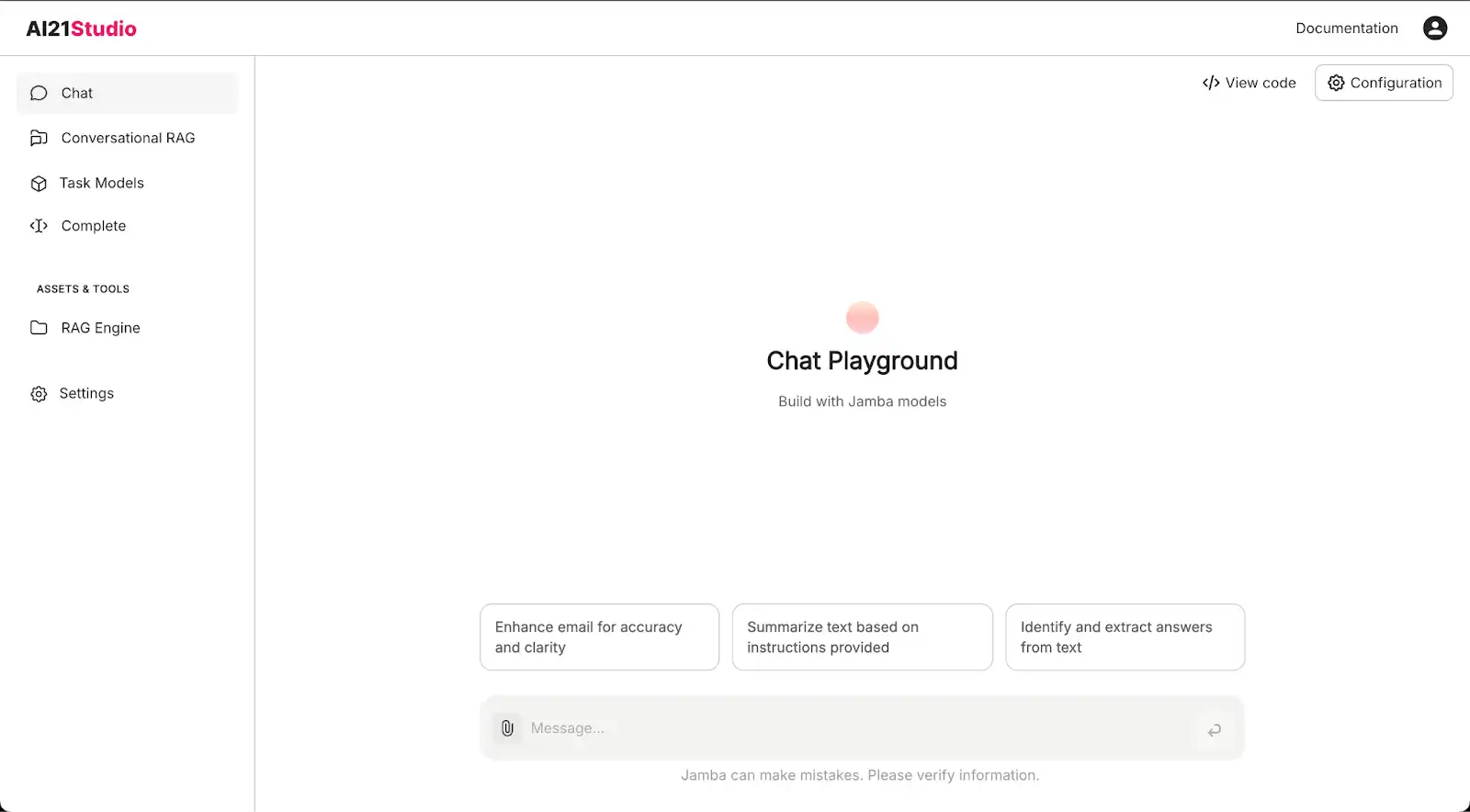
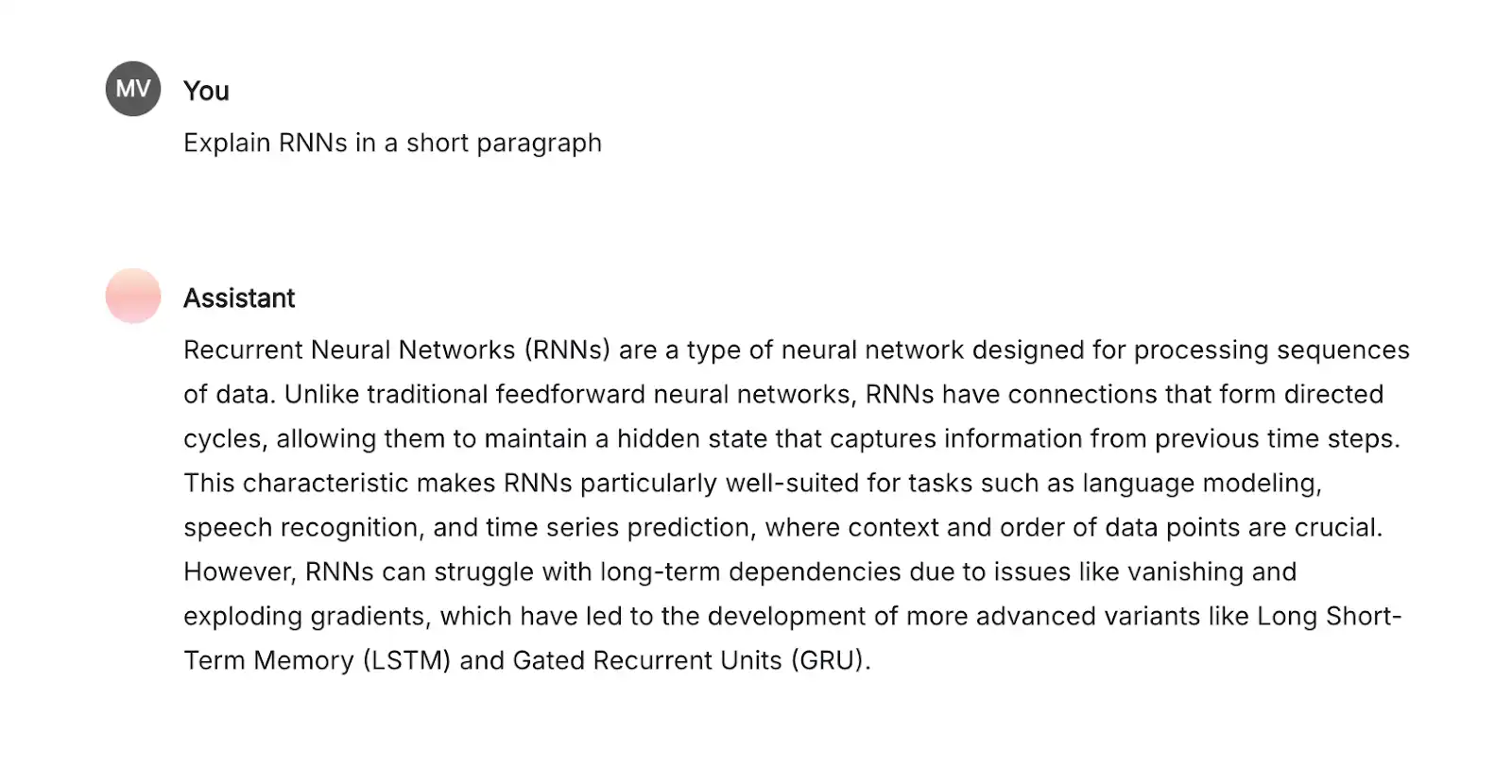
This is just a small sample of the model’s question-answering capabilities.
Jamba 1.5 using Python
You can send requests and get responses from Jamba 1.5 in Python using the API Key.
To get your API key, click on settings on the left bar of the homepage, then click on the API key.
Note: You’ll get $10 free credits, and you can track the credits you use by clicking on ‘Usage’ in the settings.
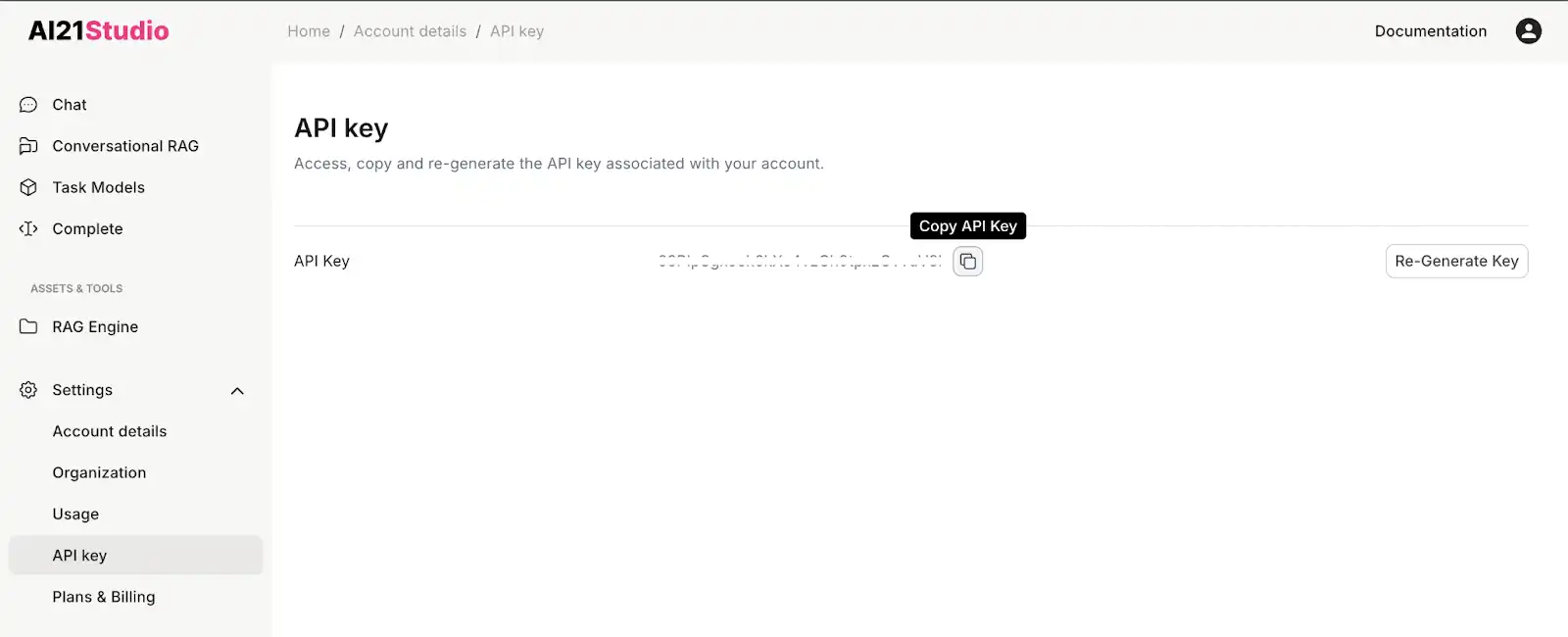
Installation
!pip install ai21
Python Code
from ai21 import AI21Client
from ai21.models.chat import ChatMessage
messages = (ChatMessage(content="What's a tokenizer in 2-3 lines?", role="user"))
client = AI21Client(api_key='')
response = client.chat.completions.create(
messages=messages,
model="jamba-1.5-mini",
stream=True
)
for chunk in response:
print(chunk.choices(0).delta.content, end="")
A tokenizer is a tool that breaks down text into smaller units called tokens, words, subwords, or characters. It is essential for natural language processing tasks, as it prepares text for analysis by models.
It’s straightforward: We send the message to our desired model and get the response using our API key.
Note: You can also choose to use the jamba-1.5-large model instead of Jamba-1.5-mini
Conclusion
Jamba 1.5 blends the strengths of the Mamba and Transformer architectures. With its scalable design, high throughput, and extensive context handling, it is well-suited for diverse applications ranging from summarization to sentiment analysis. By offering accessible integration options and optimized efficiency, it enables users to work effectively with its modelling capabilities across various environments. It can also be finetuned on domain-specific data for better results.
Frequently Asked Questions
Ans. Jamba 1.5 is a family of large language models designed with a hybrid architecture combining Transformer and Mamba elements. It includes two versions, Jamba-1.5-Large (94B active parameters) and Jamba-1.5-Mini (12B active parameters), optimized for instruction-following and conversational tasks.
Ans. Jamba 1.5 models support an effective context length of 256K tokens, made possible by its hybrid architecture and an innovative quantization technique, ExpertsInt8. This efficiency allows the models to manage long-context data with reduced memory usage.
Ans. ExpertsInt8 is a custom quantization method that compresses model weights in the MoE and MLP layers to INT8 format. This technique reduces memory usage while maintaining model quality and is compatible with A100 GPUs, enhancing serving efficiency.
Ans. Yes, both Large and Mini are publicly available under the Jamba Open Model License. The models can be accessed on Hugging Face.