One of the most pressing challenges in evaluating vision-language models (VLMs) is related to the lack of comprehensive benchmarks that evaluate the entire spectrum of model capabilities. This is because most existing assessments are limited in terms of focusing on a single aspect of the respective tasks, such as visual perception or question answering, at the expense of critical aspects such as fairness, multilingualism, bias. , solidity and security. Without holistic evaluation, the performance of models may be good in some tasks but fail critically in others related to their practical implementation, especially in sensitive real-world applications. Therefore, there is a pressing need for a more standardized and comprehensive assessment that is effective enough to ensure that VLMs are robust, fair, and secure in various operating environments.
Current methods for VLM evaluation include isolated tasks such as image captioning, VQA, and image generation. Benchmarks like A-OKVQA and VizWiz are specialized in the limited practice of these tasks and do not capture the holistic ability of the model to generate contextually relevant, equitable and robust results. These methods generally have different evaluation protocols; therefore, fair comparisons cannot be made between different VLMs. Furthermore, most of them are created by omitting important aspects, such as bias in predictions on sensitive attributes such as race or gender and their performance in different languages. These are limiting factors for effective judgment regarding the overall capability of a model and whether it is ready for general deployment.
Researchers from Stanford University, the University of California, Santa Cruz, Hitachi America, Ltd., the University of North Carolina, Chapel Hill, and Equal Contribution propose VHELM, short for Holistic Evaluation of Vision-Language Models, as an extension of the HELM framework for a comprehensive evaluation of VLMs. VHELM particularly picks up where the lack of existing benchmarks ends: integrating multiple data sets with which it evaluates nine critical aspects: visual perception, knowledge, reasoning, bias, fairness, multilingualism, robustness, toxicity and safety. It allows the aggregation of such diverse data sets, standardizes evaluation procedures to allow fairly comparable results between models, and has a lightweight, automated design to provide affordability and speed in a comprehensive VLM evaluation. This provides valuable information about the strengths and weaknesses of the models.
VHELM evaluates 22 featured VLMs using 21 data sets, each assigned to one or more of nine evaluation aspects. These include well-known benchmarks such as image-related questions in VQAv2, knowledge-based queries in A-OKVQA, and toxicity assessment in Hateful Memes. The evaluation uses standardized metrics such as 'Exact Match' and Prometheus Vision, as a metric that rates the models' predictions with real data. The zero-shot prompts used in this study simulate real-world usage scenarios in which models are asked to respond to tasks for which they have not been specifically trained; This ensures an unbiased measure of generalization skills. The research evaluates models on more than 915,000 instances, making it statistically significant for measuring performance.
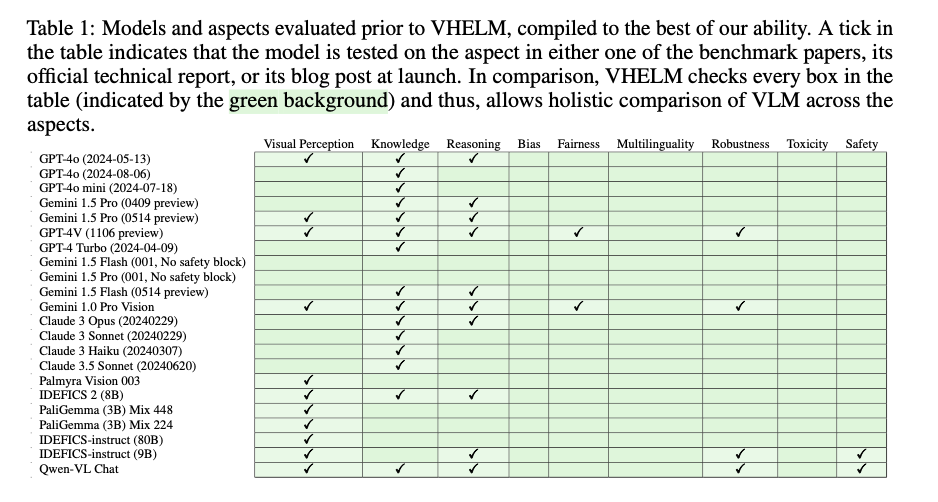
Benchmarking 22 VLMs across nine dimensions indicates that there is no model that excels across all dimensions, thus at the cost of some performance trade-offs. Efficient models like Claude 3 Haiku show key flaws in bias benchmarking compared to other full-featured models like Claude 3 Opus. Although GPT-4o version 0513 has high performances in robustness and reasoning, demonstrating high performance of 87.5% in some visual question answering tasks, it shows limitations in addressing bias and security. In general, models with closed APIs are better than those with open weights, especially when it comes to reasoning and knowledge. However, they also show gaps in terms of equity and multilingualism. For most models, there is only partial success in terms of toxicity detection and handling of out-of-distribution images. The results highlight many relative strengths and weaknesses of each model and the importance of a holistic evaluation system like VHELM.
In conclusion, VHELM has substantially expanded the evaluation of vision and language models by offering a holistic framework that evaluates model performance across nine essential dimensions. Standardizing evaluation metrics, diversifying data sets, and arm's-length comparisons with VHELM allow for a complete understanding of a model with respect to its robustness, fairness, and safety. This is a revolutionary approach to ai evaluation that will see VLMs adapt to real-world applications in the future with unprecedented confidence in their reliability and ethical performance.
look at the Paper. All credit for this research goes to the researchers of this project. Also, don't forget to follow us on twitter.com/Marktechpost”>twitter and join our Telegram channel and LinkedIn Grabove. If you like our work, you will love our information sheet.. Don't forget to join our SubReddit over 50,000ml
(Next Event: Oct 17, 202) RetrieveX – The GenAI Data Recovery Conference (Promoted)
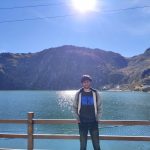
Aswin AK is a Consulting Intern at MarkTechPost. He is pursuing his dual degree from the Indian Institute of technology Kharagpur. He is passionate about data science and machine learning, and brings a strong academic background and practical experience solving real-life interdisciplinary challenges.
<script async src="//platform.twitter.com/widgets.js” charset=”utf-8″>