Generative AI has come a long way recently. We are all familiar with ChatGPT, broadcast models, and more by now. These tools are becoming more and more integrated into our daily lives. Now, we are using ChatGPT as an assistant for our daily tasks; MidJourney to help in the design process and more AI tools to make our routine tasks easier.
The advancement of generative AI models has enabled unique use cases that were previously different to achieve. we have seen someone write and illustrate a whole children’s book using generative AI models. We used to tell stories the same way for years, and now this was a great example of how generative AI can revolutionize the storytelling we’ve been using for years.
Visual storytelling is a powerful method of delivering narrative content effectively to diverse audiences. Its applications in education and entertainment, such as children’s books, are very wide. We know we can generate stories and illustrations separately using generative AI models, but can we really use them to consistently generate a visual story? The question then becomes; Given a story in plain text and portrait images of some characters, can we generate a series of images to express the story visually?
In order to have an accurate visual representation of a narrative, the visualization of the story must meet several vital requirements. First, maintaining identity consistency is crucial to consistently depicting characters and environments across frames or scenes. Second, the visual content must align closely with the textual narrative, accurately depicting the events and interactions described in the story. Lastly, a clear and logical layout of objects and characters within the generated images helps to smoothly guide the viewer’s attention through the narrative, making it easier to understand.
Generative AI has been used to propose various methods of story visualization. Early work relied on GAN or VAE-based methods and text encoders to project text into latent space, generating images conditioned by text input. While these approaches showed promise, they faced challenges generalizing to new actors, scenes, and design arrangements. Recent zero-shot story visualization attempts investigated the potential of adapting to new characters and scenes using pre-trained models. However, these methods lacked support for multiple characters and did not consider the importance of layout and local object structures within the generated images.
So should we give up on having an AI-based story display system? Are these limitations too difficult to address? Of course not! time to meet TaleCrafter.
TaleCrafter is a novel and versatile interactive story display system that overcomes the limitations of previous approaches. The system consists of four key components: story-to-message (S2P) generation, text-to-layout (T2L) generation, controllable text-to-image (C-T2I) generation, and image-to-video (I2V) animation. ).
These components work together to address the requirements of a story display system. Story-to-Ad Generation (The S2P component leverages a large language model to generate ads that render the visual content of images based on instructions derived from the story. The Text-to-Layout (T2L) generation component uses the generated ad to generate a design image that provides location guidance for the main themes, then the controllable text-to-image generation (C-T2I) module, the central component of the display system, renders the images conditioned by the design, the local sketch and the ad Finally, the Image-to-Video (I2V) animation component enriches the visualization process by animating the generated images, providing a more vivid and engaging presentation of the story.
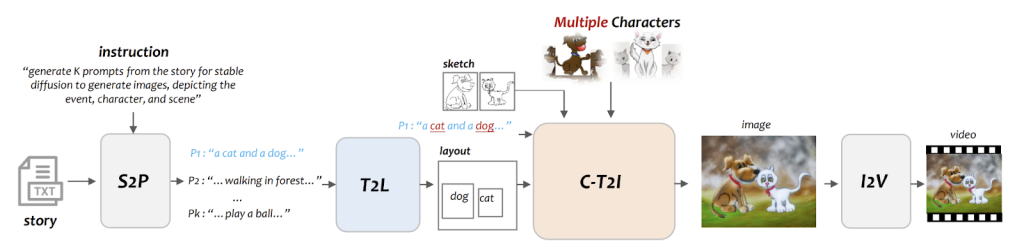
TaleCrafter overview. Fountain: https://arxiv.org/pdf/2305.18247.pdf
TaleCrafter‘s The main contributions lie in two key aspects. First, the proposed story visualization system takes advantage of extensive language and pre-trained text-to-image (T2I) models to generate a video from plain text stories. This versatile system can handle multiple novel characters and scenes, overcoming the limitations of previous approaches that were limited to specific data sets. Second, the Controllable Text-to-Image Generation (C-T2I) module emphasizes multi-character identity preservation and provides control over local object layout and structures, enabling interactive editing and customization.
review the Paper and github link. Don’t forget to join our 23k+ ML SubReddit, discord channel, and electronic newsletter, where we share the latest AI research news, exciting AI projects, and more. If you have any questions about the article above or if we missed anything, feel free to email us at [email protected]
🚀 Check out 100 AI tools at AI Tools Club
Ekrem Çetinkaya received his B.Sc. in 2018 and M.Sc. in 2019 from Ozyegin University, Istanbul, Türkiye. She wrote her M.Sc. thesis on denoising images using deep convolutional networks. She is currently pursuing a PhD. She graduated from the University of Klagenfurt, Austria, and working as a researcher in the ATHENA project. Her research interests include deep learning, computer vision, and multimedia networks.