(LLMS) están mejorando en eficiencia y ahora pueden comprender diferentes formatos de datos, ofreciendo posibilidades de miríadas de aplicaciones en diferentes dominios. Inicialmente, los LLM fueron inherentemente capaces de procesar solo texto. La función de comprensión de la imagen se integró acoplando una LLM con otro modelo de codificación de imagen. Sin embargo, gpt-4o
fue entrenado tanto en texto como en imágenes y es el primer LLM multimodal verdadero que puede comprender tanto el texto como las imágenes. Otras modalidades como el audio se integran en los LLM modernos a través de otros modelos de IA, por ejemplo, los modelos Whisper de OpenAI.
Los LLM ahora se están utilizando más como procesadores de información donde pueden procesar datos en diferentes formatos. La integración de múltiples modalidades en LLM abre áreas de numerosas aplicaciones en educación, negocios y otros sectores. Una de esas aplicaciones es el procesamiento de videos educativos, documentales, seminarios web, presentaciones, reuniones de negocios, conferencias y otro contenido que usa LLM e interactúa con este contenido de manera más natural. La modalidad de audio en estos videos contiene información rica que podría usarse en varias aplicaciones. En entornos educativos, se puede utilizar para el aprendizaje personalizado, mejorar la accesibilidad de los estudiantes con necesidades especiales, estudiar la creación de ayuda, el apoyo de aprendizaje remoto sin requerir la presencia de un maestro para comprender el contenido y evaluar el conocimiento de los estudiantes sobre un tema. En entornos comerciales, se puede utilizar para capacitar a nuevos empleados con videos de incorporación, extraer y generar conocimiento a partir de reuniones y presentaciones de grabación, materiales de aprendizaje personalizados de videos de demostración de productos y extraer información de conferencias de la industria grabadas sin ver horas de videos, por nombrar algunos.
Este artículo analiza el desarrollo de una aplicación para interactuar con videos de manera natural y crear contenido de aprendizaje de ellos. La aplicación tiene las siguientes características:
- Toma un video de entrada a través de una URL o desde una ruta local y extrae audio del video
- Transcribe el audio utilizando el modelo de última generación de OpenAI
gpt-4o-transcribe
, que ha demostrado un rendimiento mejorado de la tasa de error de palabras (WER) sobre los modelos Whisper existentes en múltiples puntos de referencia establecidos - Crea un almacén vectorial de la transcripción y desarrolla una generación de aumento de recuperación (trapo) para establecer una conversación con la transcripción del video
- Responda a las preguntas de los usuarios en texto y discurso utilizando diferentes voces, seleccionables de la interfaz de usuario de la aplicación.
- Crea contenido de aprendizaje como:
- Representación jerárquica del contenido de video para proporcionar a los usuarios información rápida sobre los conceptos principales y los detalles de apoyo
- Genere cuestionarios para transformar videos pasivos observando el aprendizaje activo desafiando a los usuarios a recordar y aplicar información presentada en el video.
- Genera tarjetas de flash del contenido de video que admite técnicas de aprendizaje de repetición de retiro activo y espaciado
Todo el flujo de trabajo de la aplicación se muestra en la siguiente figura.
Toda la base de código, junto con instrucciones detalladas para la instalación y el uso, está disponible en Girub.
Aquí está la estructura del repositorio de GitHub. La aplicación principal de línea de transmisión implementa la interfaz GUI y llama a varias otras funciones de otras características y módulos auxiliares (.py
archivos).
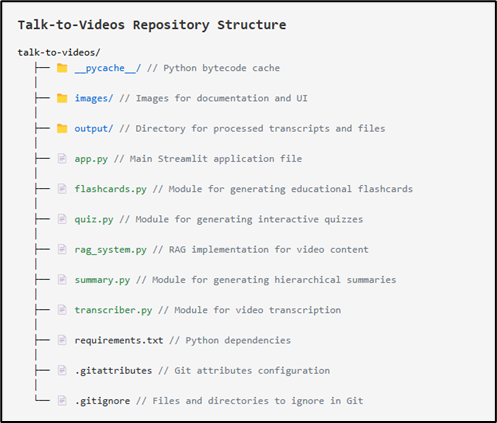
Además, puede visualizar la base de código abriendo el “Visualización de la base de código“Archivo HTML en un navegador, que describe las estructuras de cada módulo.

Profundicemos en el desarrollo paso a paso de esta aplicación. No discutiré todo el código, sino solo su parte principal. Todo el código en el repositorio de GitHub se comenta adecuadamente.
Entrada y procesamiento de video
La lógica de entrada y procesamiento de video se implementan en transcriber.py
. Cuando la aplicación se carga, verifica si FFMPEG está presente (verify_ffmpeg
) en el directorio raíz de la aplicación. Se requiere FFMPEG para descargar un video (si la entrada es una URL) y extraer audio del video que luego se usa para crear una transcripción.
def verify_ffmpeg():
"""Verify that FFmpeg is available and print its location."""
# Add FFmpeg to PATH
os.environ('PATH') = FFMPEG_LOCATION + os.pathsep + os.environ('PATH')
# Check if FFmpeg binaries exist
ffmpeg_path = os.path.join(FFMPEG_LOCATION, 'ffmpeg.exe')
ffprobe_path = os.path.join(FFMPEG_LOCATION, 'ffprobe.exe')
if not os.path.exists(ffmpeg_path):
raise FileNotFoundError(f"FFmpeg executable not found at: {ffmpeg_path}")
if not os.path.exists(ffprobe_path):
raise FileNotFoundError(f"FFprobe executable not found at: {ffprobe_path}")
print(f"FFmpeg found at: {ffmpeg_path}")
print(f"FFprobe found at: {ffprobe_path}")
# Try to execute FFmpeg to make sure it works
try:
# Add shell=True for Windows and capture errors properly
result = subprocess.run((ffmpeg_path, '-version'),
stdout=subprocess.PIPE,
stderr=subprocess.PIPE,
shell=True, # This can help with permission issues on Windows
check=False)
if result.returncode == 0:
print(f"FFmpeg version: {result.stdout.decode().splitlines()(0)}")
else:
error_msg = result.stderr.decode()
print(f"FFmpeg error: {error_msg}")
# Check for specific permission errors
if "Access is denied" in error_msg:
print("Permission error detected. Trying alternative approach...")
# Try an alternative approach - just check file existence without execution
if os.path.exists(ffmpeg_path) and os.path.exists(ffprobe_path):
print("FFmpeg files exist but execution test failed due to permissions.")
print("WARNING: The app may fail when trying to process videos.")
# Return paths anyway and hope for the best when actually used
return ffmpeg_path, ffprobe_path
raise RuntimeError(f"FFmpeg execution failed: {error_msg}")
except Exception as e:
print(f"Error checking FFmpeg: {e}")
# Fallback option if verification fails but files exist
if os.path.exists(ffmpeg_path) and os.path.exists(ffprobe_path):
print("WARNING: FFmpeg files exist but verification failed.")
print("Attempting to continue anyway, but video processing may fail.")
return ffmpeg_path, ffprobe_path
raise
return ffmpeg_path, ffprobe_path
La entrada de video tiene la forma de una URL (por ejemplo, URL de YouTube) o una ruta de archivo local. El process_video
La función determina el tipo de entrada y lo ruta en consecuencia. Si la entrada es una URL, el ayudante funciona get_video_info
y get_video_id
Extraer metadatos de video (título, descripción, duración) sin descargarlo usando yt_dlp
paquete.
#Function to determine the input type and route it appropriately
def process_video(youtube_url, output_dir, api_key, model="gpt-4o-transcribe"):
"""
Process a YouTube video to generate a transcript
Wrapper function that combines download and transcription
Args:
youtube_url: URL of the YouTube video
output_dir: Directory to save the output
api_key: OpenAI API key
model: The model to use for transcription (default: gpt-4o-transcribe)
Returns:
dict: Dictionary containing transcript and file paths
"""
# First download the audio
print("Downloading video...")
audio_path = process_video_download(youtube_url, output_dir)
print("Transcribing video...")
# Then transcribe the audio
transcript, transcript_path = process_video_transcribe(audio_path, output_dir, api_key, model=model)
# Return the combined results
return {
'transcript': transcript,
'transcript_path': transcript_path,
'audio_path': audio_path
}
def get_video_info(youtube_url):
"""Get video information without downloading."""
# Check local cache first
global _video_info_cache
if youtube_url in _video_info_cache:
return _video_info_cache(youtube_url)
# Extract info if not cached
with yt_dlp.YoutubeDL() as ydl:
info = ydl.extract_info(youtube_url, download=False)
# Cache the result
_video_info_cache(youtube_url) = info
# Also cache the video ID separately
_video_id_cache(youtube_url) = info.get('id', 'video')
return info
def get_video_id(youtube_url):
"""Get just the video ID without re-extracting if already known."""
global _video_id_cache
if youtube_url in _video_id_cache:
return _video_id_cache(youtube_url)
# If not in cache, extract from URL directly if possible
if "v=" in youtube_url:
video_id = youtube_url.split("v=")(1).split("&")(0)
_video_id_cache(youtube_url) = video_id
return video_id
elif "youtu.be/" in youtube_url:
video_id = youtube_url.split("youtu.be/")(1).split("?")(0)
_video_id_cache(youtube_url) = video_id
return video_id
# If we can't extract directly, fall back to full info extraction
info = get_video_info(youtube_url)
video_id = info.get('id', 'video')
return video_id
Después de que se proporciona la entrada de video, el código en app.py
Verifica si ya existe una transcripción para el video de entrada (en el caso de la entrada de URL). Esto se hace llamando a las siguientes dos funciones auxiliares de transcriber.py
.
def get_transcript_path(youtube_url, output_dir):
"""Get the expected transcript path for a given YouTube URL."""
# Get video ID with caching
video_id = get_video_id(youtube_url)
# Return expected transcript path
return os.path.join(output_dir, f"{video_id}_transcript.txt")
def transcript_exists(youtube_url, output_dir):
"""Check if a transcript already exists for this video."""
transcript_path = get_transcript_path(youtube_url, output_dir)
return os.path.exists(transcript_path)
Si transcript_exists
Devuelve la ruta de una transcripción existente, el siguiente paso es crear la tienda vectorial para el trapo. Si no se encuentra una transcripción existente, el siguiente paso es descargar audio de la URL y convertirlo en un formato de audio estándar. La función process_video_download
descarga audio de la URL usando la biblioteca ffmpeg y la convierte en .mp3
formato. Si la entrada es un archivo de video local, app.py
procede a convertirlo en .mp3
archivo.
def process_video_download(youtube_url, output_dir):
"""
Download audio from a YouTube video
Args:
youtube_url: URL of the YouTube video
output_dir: Directory to save the output
Returns:
str: Path to the downloaded audio file
"""
# Create output directory if it doesn't exist
os.makedirs(output_dir, exist_ok=True)
# Extract video ID from URL
video_id = None
if "v=" in youtube_url:
video_id = youtube_url.split("v=")(1).split("&")(0)
elif "youtu.be/" in youtube_url:
video_id = youtube_url.split("youtu.be/")(1).split("?")(0)
else:
raise ValueError("Could not extract video ID from URL")
# Set output paths
audio_path = os.path.join(output_dir, f"{video_id}.mp3")
# Configure yt-dlp options
ydl_opts = {
'format': 'bestaudio/best',
'postprocessors': ({
'key': 'FFmpegExtractAudio',
'preferredcodec': 'mp3',
'preferredquality': '192',
}),
'outtmpl': os.path.join(output_dir, f"{video_id}"),
'quiet': True
}
# Download audio
with yt_dlp.YoutubeDL(ydl_opts) as ydl:
ydl.download((youtube_url))
# Verify audio file exists
if not os.path.exists(audio_path):
# Try with an extension that yt-dlp might have used
potential_paths = (
os.path.join(output_dir, f"{video_id}.mp3"),
os.path.join(output_dir, f"{video_id}.m4a"),
os.path.join(output_dir, f"{video_id}.webm")
)
for path in potential_paths:
if os.path.exists(path):
# Convert to mp3 if it's not already
if not path.endswith('.mp3'):
ffmpeg_path = verify_ffmpeg()(0)
output_mp3 = os.path.join(output_dir, f"{video_id}.mp3")
subprocess.run((
ffmpeg_path, '-i', path, '-c:a', 'libmp3lame',
'-q:a', '2', output_mp3, '-y'
), check=True, capture_output=True)
os.remove(path) # Remove the original file
audio_path = output_mp3
else:
audio_path = path
break
else:
raise FileNotFoundError(f"Could not find downloaded audio file for video {video_id}")
return audio_path
Transcripción de audio utilizando OpenAI's gpt-4o-transcribe
Modelo
Después de extraer audio y convertirlo en un formato de audio estándar, el siguiente paso es transcribir el formato de audio a texto. Para este propósito, utilicé el recién lanzado de Openai gpt-4o-transcribe
modelo de voz a texto accesible a través de API de voz a texto. Este modelo ha superado a OpenAi's Susurro Modelos en términos de precisión de transcripción y cobertura de lenguaje robusto.
La función process_video_transcribe
en transcriber.py
recibe el archivo de audio convertido e interfaces con gpt-4o-transcribe
Modelo con la API de voz a texto de OpenAI. El gpt-4o-transcribe
El modelo tiene actualmente un límite de archivo de audio de 25 MB y 1500 de duración. Para superar esta limitación, dividí los archivos más largos en múltiples fragmentos y transcribo estos trozos por separado. El process_video_transcribe
La función verifica si el archivo de entrada excede el tamaño y/o el límite de duración. Si se excede cualquier umbral, llama split_and_transcribe
La función, que primero calcula el número de fragmentos necesarios en función del tamaño y la duración, y toma el máximo de estos dos como el número final de fragmentos para la transcripción. Luego encuentra los horarios de inicio y finalización para cada fragmento y extrae estos fragmentos del archivo de audio. Posteriormente, transcribe cada fragmento usando gpt-4o-transcribe
modelo con la API de voz a texto de OpenAI y luego combina transcripciones de todos los fragmentos para generar la transcripción final.
def process_video_transcribe(audio_path, output_dir, api_key, progress_callback=None, model="gpt-4o-transcribe"):
"""
Transcribe an audio file using OpenAI API, with automatic chunking for large files
Always uses the selected model, with no fallback
Args:
audio_path: Path to the audio file
output_dir: Directory to save the transcript
api_key: OpenAI API key
progress_callback: Function to call with progress updates (0-100)
model: The model to use for transcription (default: gpt-4o-transcribe)
Returns:
tuple: (transcript text, transcript path)
"""
# Extract video ID from audio path
video_id = os.path.basename(audio_path).split('.')(0)
transcript_path = os.path.join(output_dir, f"{video_id}_transcript.txt")
# Setup OpenAI client
client = OpenAI(api_key=api_key)
# Update progress
if progress_callback:
progress_callback(10)
# Get file size in MB
file_size_mb = os.path.getsize(audio_path) / (1024 * 1024)
# Universal chunking thresholds - apply to both models
max_size_mb = 25 # 25MB chunk size for both models
max_duration_seconds = 1500 # 1500 seconds chunk duration for both models
# Load the audio file to get its duration
try:
audio = AudioSegment.from_file(audio_path)
duration_seconds = len(audio) / 1000 # pydub uses milliseconds
except Exception as e:
print(f"Error loading audio to check duration: {e}")
audio = None
duration_seconds = 0
# Determine if chunking is needed
needs_chunking = False
chunking_reason = ()
if file_size_mb > max_size_mb:
needs_chunking = True
chunking_reason.append(f"size ({file_size_mb:.2f}MB exceeds {max_size_mb}MB)")
if duration_seconds > max_duration_seconds:
needs_chunking = True
chunking_reason.append(f"duration ({duration_seconds:.2f}s exceeds {max_duration_seconds}s)")
# Log the decision
if needs_chunking:
reason_str = " and ".join(chunking_reason)
print(f"Audio needs chunking due to {reason_str}. Using {model} for transcription.")
else:
print(f"Audio file is within limits. Using {model} for direct transcription.")
# Check if file needs chunking
if needs_chunking:
if progress_callback:
progress_callback(15)
# Split the audio file into chunks and transcribe each chunk using the selected model only
full_transcript = split_and_transcribe(
audio_path, client, model, progress_callback,
max_size_mb, max_duration_seconds, audio
)
else:
# File is small enough, transcribe directly with the selected model
with open(audio_path, "rb") as audio_file:
if progress_callback:
progress_callback(30)
transcript_response = client.audio.transcriptions.create(
model=model,
file=audio_file
)
if progress_callback:
progress_callback(80)
full_transcript = transcript_response.text
# Save transcript to file
with open(transcript_path, "w", encoding="utf-8") as f:
f.write(full_transcript)
# Update progress
if progress_callback:
progress_callback(100)
return full_transcript, transcript_path
def split_and_transcribe(audio_path, client, model, progress_callback=None,
max_size_mb=25, max_duration_seconds=1500, audio=None):
"""
Split an audio file into chunks and transcribe each chunk
Args:
audio_path: Path to the audio file
client: OpenAI client
model: Model to use for transcription (will not fall back to other models)
progress_callback: Function to call with progress updates
max_size_mb: Maximum file size in MB
max_duration_seconds: Maximum duration in seconds
audio: Pre-loaded AudioSegment (optional)
Returns:
str: Combined transcript from all chunks
"""
# Load the audio file if not provided
if audio is None:
audio = AudioSegment.from_file(audio_path)
# Get audio duration in seconds
duration_seconds = len(audio) / 1000
# Calculate the number of chunks needed based on both size and duration
file_size_mb = os.path.getsize(audio_path) / (1024 * 1024)
chunks_by_size = math.ceil(file_size_mb / (max_size_mb * 0.9)) # Use 90% of max to be safe
chunks_by_duration = math.ceil(duration_seconds / (max_duration_seconds * 0.95)) # Use 95% of max to be safe
num_chunks = max(chunks_by_size, chunks_by_duration)
print(f"Splitting audio into {num_chunks} chunks based on size ({chunks_by_size}) and duration ({chunks_by_duration})")
# Calculate chunk duration in milliseconds
chunk_length_ms = len(audio) // num_chunks
# Create temp directory for chunks if it doesn't exist
temp_dir = os.path.join(os.path.dirname(audio_path), "temp_chunks")
os.makedirs(temp_dir, exist_ok=True)
# Split the audio into chunks and transcribe each chunk
transcripts = ()
for i in range(num_chunks):
if progress_callback:
# Update progress: 20% for splitting, 60% for transcribing
progress_percent = 20 + int((i / num_chunks) * 60)
progress_callback(progress_percent)
# Calculate start and end times for this chunk
start_ms = i * chunk_length_ms
end_ms = min((i + 1) * chunk_length_ms, len(audio))
# Extract the chunk
chunk = audio(start_ms:end_ms)
# Save the chunk to a temporary file
chunk_path = os.path.join(temp_dir, f"chunk_{i}.mp3")
chunk.export(chunk_path, format="mp3")
# Log chunk information
chunk_size_mb = os.path.getsize(chunk_path) / (1024 * 1024)
chunk_duration = len(chunk) / 1000
print(f"Chunk {i+1}/{num_chunks}: {chunk_size_mb:.2f}MB, {chunk_duration:.2f}s")
# Transcribe the chunk
try:
with open(chunk_path, "rb") as chunk_file:
transcript_response = client.audio.transcriptions.create(
model=model,
file=chunk_file
)
# Add to our list of transcripts
transcripts.append(transcript_response.text)
except Exception as e:
print(f"Error transcribing chunk {i+1} with {model}: {e}")
# Add a placeholder for the failed chunk
transcripts.append(f"(Transcription failed for segment {i+1})")
# Clean up the temporary chunk file
os.remove(chunk_path)
# Clean up the temporary directory
try:
os.rmdir(temp_dir)
except:
print(f"Note: Could not remove temporary directory {temp_dir}")
# Combine all transcripts with proper spacing
full_transcript = " ".join(transcripts)
return full_transcript
La siguiente captura de pantalla de la aplicación Streamlit muestra el flujo de trabajo de procesamiento y transcripción de videos para uno de mis seminarios web, “Integrar LLM en negocios“ Disponible en mi canal de YouTube.
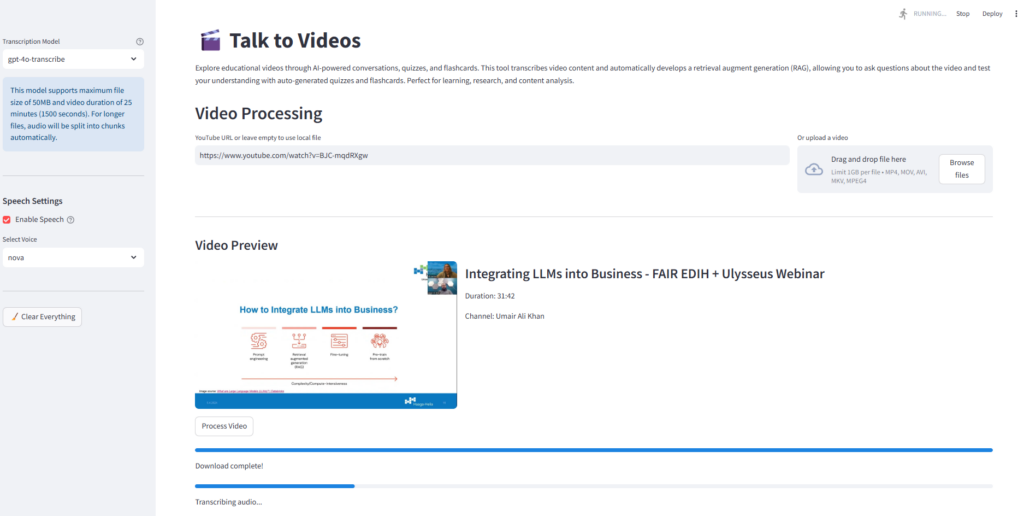
Generación aumentada de recuperación (RAG) para conversaciones interactivas
Después de generar la transcripción del video, la aplicación desarrolla un trapo para facilitar tanto el texto como las interacciones basadas en el habla. La inteligencia conversacional se implementa a través de VideoRAG
clasificar rag_system.py
que inicializa el tamaño y la superposición de la fragmentación, abiertos, incrustaciones, ChatOpenAI
instancia para generar respuestas con gpt-4o
modelo, y ConversationBufferMemory
Para mantener el historial de chat para la continuidad contextual.
El create_vector_store
El método divide los documentos en trozos y crea una tienda vectorial utilizando la base de datos FAISS Vector. El handle_question_submission
El método procesa preguntas de texto y agrega cada nueva pregunta y su respuesta al historial de conversación. La función HANGE_SPECH_INPUT implementa la tubería completa de voz a texto a voz. Primero registra el audio de la pregunta, transcribe la pregunta, procesa la consulta a través del sistema RAG y sintetiza el habla para la respuesta.
class VideoRAG:
def __init__(self, api_key=None, chunk_size=1000, chunk_overlap=200):
"""Initialize the RAG system with OpenAI API key."""
# Use provided API key or try to get from environment
self.api_key = api_key if api_key else st.secrets("OPENAI_API_KEY")
if not self.api_key:
raise ValueError("OpenAI API key is required either as parameter or environment variable")
self.embeddings = OpenAIEmbeddings(openai_api_key=self.api_key)
self.llm = ChatOpenAI(
openai_api_key=self.api_key,
model="gpt-4o",
temperature=0
)
self.chunk_size = chunk_size
self.chunk_overlap = chunk_overlap
self.vector_store = None
self.chain = None
self.memory = ConversationBufferMemory(
memory_key="chat_history",
return_messages=True
)
def create_vector_store(self, transcript):
"""Create a vector store from the transcript."""
# Split the text into chunks
text_splitter = RecursiveCharacterTextSplitter(
chunk_size=self.chunk_size,
chunk_overlap=self.chunk_overlap,
separators=("nn", "n", " ", "")
)
chunks = text_splitter.split_text(transcript)
# Create vector store
self.vector_store = FAISS.from_texts(chunks, self.embeddings)
# Create prompt template for the RAG system
system_template = """You are a specialized ai assistant that answers questions about a specific video.
You have access to snippets from the video transcript, and your role is to provide accurate information ONLY based on these snippets.
Guidelines:
1. Only answer questions based on the information provided in the context from the video transcript, otherwise say that "I don't know. The video doesn't cover that information."
2. The question may ask you to summarize the video or tell what the video is about. In that case, present a summary of the context.
3. Don't make up information or use knowledge from outside the provided context
4. Keep your answers concise and directly related to the question
5. If asked about your capabilities or identity, explain that you're an ai assistant that specializes in answering questions about this specific video
Context from the video transcript:
{context}
Chat History:
{chat_history}
"""
user_template = "{question}"
# Create the messages for the chat prompt
messages = (
SystemMessagePromptTemplate.from_template(system_template),
HumanMessagePromptTemplate.from_template(user_template)
)
# Create the chat prompt
qa_prompt = ChatPromptTemplate.from_messages(messages)
# Initialize the RAG chain with the custom prompt
self.chain = ConversationalRetrievalChain.from_llm(
llm=self.llm,
retriever=self.vector_store.as_retriever(
search_kwargs={"k": 5}
),
memory=self.memory,
combine_docs_chain_kwargs={"prompt": qa_prompt},
verbose=True
)
return len(chunks)
def set_chat_history(self, chat_history):
"""Set chat history from external session state."""
if not self.memory:
return
# Clear existing memory
self.memory.clear()
# Convert standard chat history format to LangChain message format
for message in chat_history:
if message("role") == "user":
self.memory.chat_memory.add_user_message(message("content"))
elif message("role") == "assistant":
self.memory.chat_memory.add_ai_message(message("content"))
def ask(self, question, chat_history=None):
"""Ask a question to the RAG system."""
if not self.chain:
raise ValueError("Vector store not initialized. Call create_vector_store first.")
# If chat history is provided, update the memory
if chat_history:
self.set_chat_history(chat_history)
# Get response
response = self.chain.invoke({"question": question})
return response("answer")
Vea la siguiente instantánea de la aplicación Streamlit, que muestra la interfaz de conversación interactiva con el video.
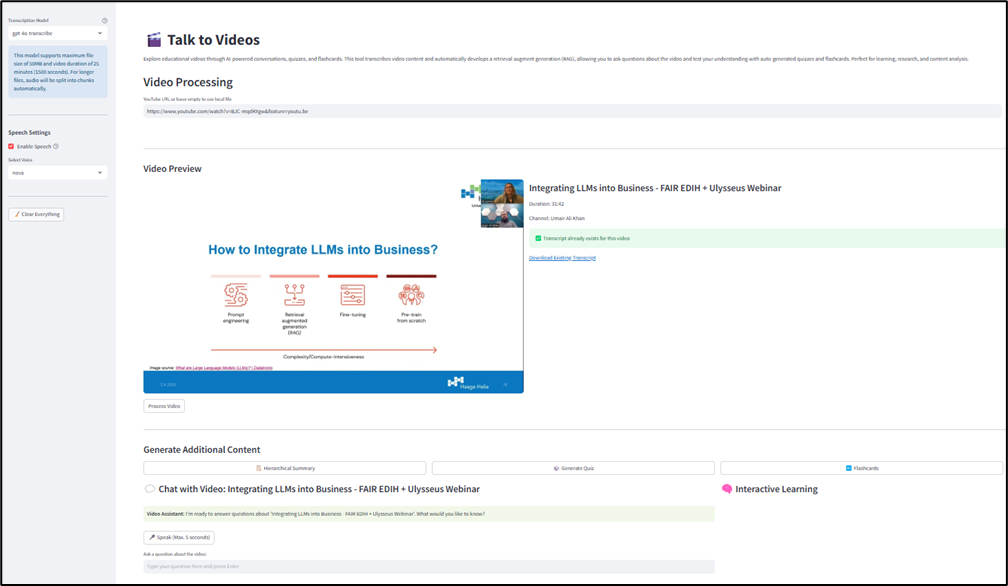
La siguiente instantánea muestra una conversación con el video con entrada de voz y texto+salida de voz.
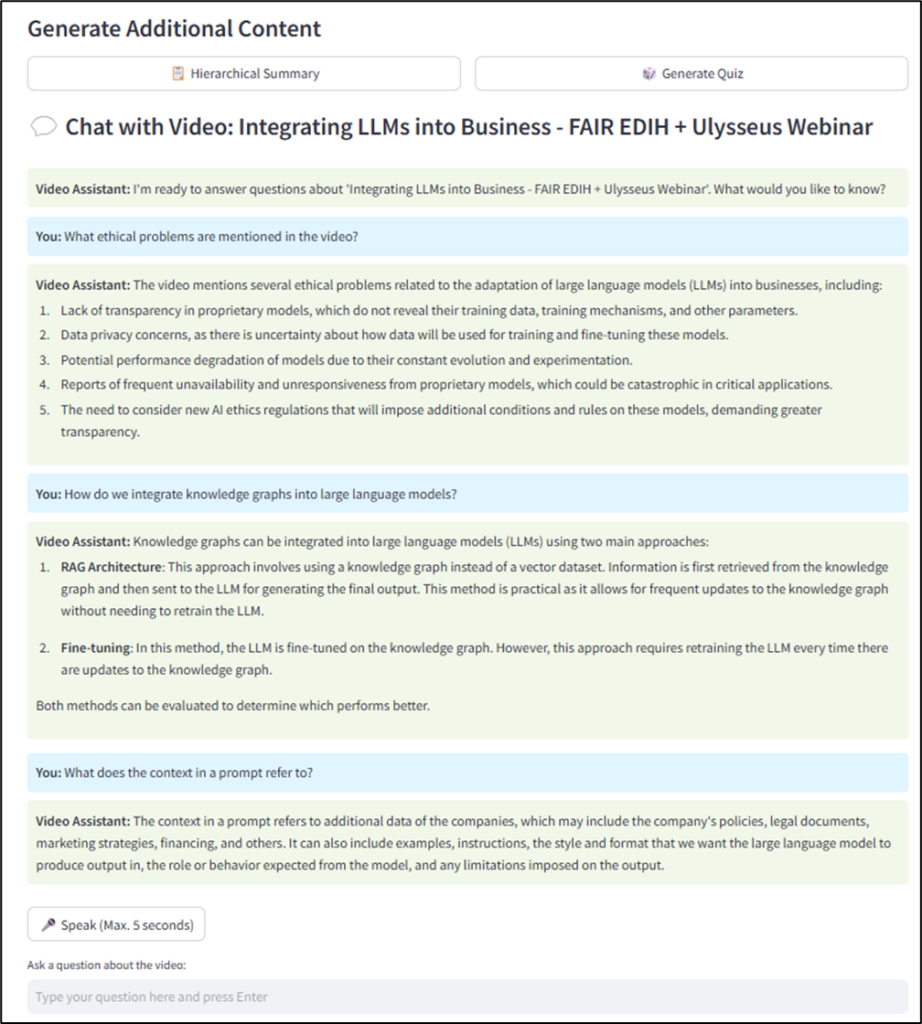
Generación de características
La aplicación genera tres características: resumen jerárquico, cuestionario y tarjetas de flash. Consulte sus respectivos códigos comentados en el Repositorio de Github.
El SummaryGenerator
clasificar summary.py
Proporciona resumen de contenido estructurado mediante la creación de una representación jerárquica del contenido de video para proporcionar a los usuarios información rápida sobre los conceptos principales y los detalles de apoyo. El sistema recupera segmentos contextuales clave de la transcripción utilizando RAG. Usando un aviso (ver generate_summary
), crea un resumen jerárquico con tres niveles: puntos principales, subpuntos y detalles adicionales. El create_summary_popup_html
El método transforma el resumen generado en una representación visual interactiva utilizando CSS y JavaScript.
# summary.py
class SummaryGenerator:
def __init__(self):
pass
def generate_summary(self, rag_system, api_key, model="gpt-4o", temperature=0.2):
"""
Generate a hierarchical bullet-point summary from the video transcript
Args:
rag_system: The RAG system with vector store
api_key: OpenAI API key
model: Model to use for summary generation
temperature: Creativity level (0.0-1.0)
Returns:
str: Hierarchical bullet-point summary text
"""
if not rag_system:
st.error("Please transcribe the video first before creating a summary!")
return ""
with st.spinner("Generating hierarchical summary..."):
# Create LLM for summary generation
summary_llm = ChatOpenAI(
openai_api_key=api_key,
model=model,
temperature=temperature # Lower temperature for more factual summaries
)
# Use the RAG system to get relevant context
try:
# Get broader context since we're summarizing the whole video
relevant_docs = rag_system.vector_store.similarity_search(
"summarize the main points of this video", k=10
)
context = "nn".join((doc.page_content for doc in relevant_docs))
prompt = """Based on the video transcript, create a hierarchical bullet-point summary of the content.
Structure your summary with exactly these levels:
• Main points (use • or * at the start of the line for these top-level points)
- Sub-points (use - at the start of the line for these second-level details)
* Additional details (use spaces followed by * for third-level points)
For example:
• First main point
- Important detail about the first point
- Another important detail
* A specific example
* Another specific example
• Second main point
- Detail about second point
Be consistent with the exact formatting shown above. Each bullet level must start with the exact character shown (• or *, -, and spaces+*).
Create 3-5 main points with 2-4 sub-points each, and add third-level details where appropriate.
Focus on the most important information from the video.
"""
# Use the LLM with context to generate the summary
messages = (
{"role": "system", "content": f"You are given the following context from a video transcript:nn{context}nnUse this context to create a hierarchical summary according to the instructions."},
{"role": "user", "content": prompt}
)
response = summary_llm.invoke(messages)
return response.content
except Exception as e:
# Fallback to the regular RAG system if there's an error
st.warning(f"Using standard summary generation due to error: {str(e)}")
return rag_system.ask(prompt)
def create_summary_popup_html(self, summary_content):
"""
Create HTML for the summary popup with properly formatted hierarchical bullets
Args:
summary_content: Raw summary text with markdown bullet formatting
Returns:
str: HTML for the popup with properly formatted bullets
"""
# Instead of relying on markdown conversion, let's manually parse and format the bullet points
lines = summary_content.strip().split('n')
formatted_html = ()
in_list = False
list_level = 0
for line in lines:
line = line.strip()
# Skip empty lines
if not line:
continue
# Detect if this is a markdown header
if line.startswith('# '):
if in_list:
# Close any open lists
for _ in range(list_level):
formatted_html.append('')
in_list = False
list_level = 0
formatted_html.append(f'')
continue
# Check line for bullet point markers
if line.startswith('• ') or line.startswith('* '):
# Top level bullet
content = line(2:).strip()
if not in_list:
# Start a new list
formatted_html.append('')
in_list = True
list_level = 1
elif list_level > 1:
# Close nested lists to get back to top level
for _ in range(list_level - 1):
formatted_html.append('
')
list_level = 1
else:
# Close previous list item if needed
if formatted_html and not formatted_html(-1).endswith('') and in_list:
formatted_html.append('')
formatted_html.append(f'{content}')
elif line.startswith('- '):
# Second level bullet
content = line(2:).strip()
if not in_list:
# Start new lists
formatted_html.append('- Second level items')
formatted_html.append('
')
in_list = True
list_level = 2
elif list_level == 1:
# Add a nested list
formatted_html.append('')
list_level = 2
elif list_level > 2:
# Close deeper nested lists to get to second level
for _ in range(list_level - 2):
formatted_html.append('
')
list_level = 2
else:
# Close previous list item if needed
if formatted_html and not formatted_html(-1).endswith('
') and list_level == 2:
formatted_html.append('')
formatted_html.append(f'{content}')
elif line.startswith(' * ') or line.startswith(' * '):
# Third level bullet
content = line.strip()(2:).strip()
if not in_list:
# Start new lists (all levels)
formatted_html.append('- Top level')
formatted_html.append('
- Second level')
formatted_html.append('
')
in_list = True
list_level = 3
elif list_level == 2:
# Add a nested list
formatted_html.append('')
list_level = 3
elif list_level < 3:
# We missed a level, adjust
formatted_html.append('- Missing level
')
formatted_html.append('')
list_level = 3
else:
# Close previous list item if needed
if formatted_html and not formatted_html(-1).endswith('
') and list_level == 3:
formatted_html.append('
')
formatted_html.append(f'- {content}')
else:
# Regular paragraph
if in_list:
# Close any open lists
for _ in range(list_level):
formatted_html.append('
')
if list_level > 1:
formatted_html.append(' ')
in_list = False
list_level = 0
formatted_html.append(f'{line}
')
# Close any open lists
if in_list:
# Close final item
formatted_html.append('')
# Close any open lists
for _ in range(list_level):
if list_level > 1:
formatted_html.append('')
else:
formatted_html.append('')
summary_html = 'n'.join(formatted_html)
html = f"""
"""
return html
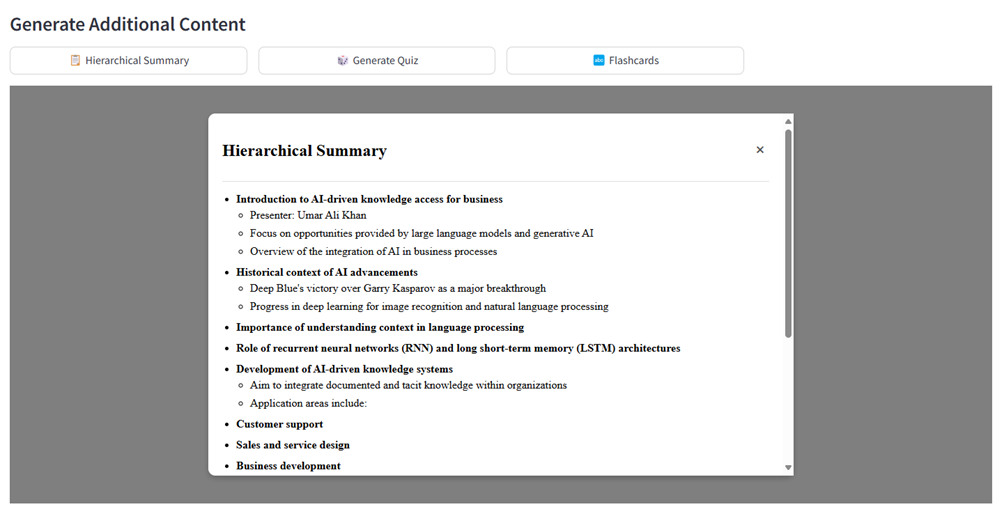
La aplicación Talk-to-Videos genera cuestionarios del video a través del QuizGenerator
clasificar quiz.py
. El generador de preguntas crea preguntas de opción múltiple dirigida a hechos y conceptos específicos presentados en el video. A diferencia de Rag, donde uso una temperatura cero, aumenté la temperatura de LLM a 0.4 para fomentar cierta creatividad en la generación de cuestionarios. Un aviso estructurado guía el proceso de generación de preguntas. El parse_quiz_response
El método extrae y valida los elementos de prueba generados para asegurarse de que cada pregunta tenga todos los componentes requeridos. Para evitar que los usuarios reconozcan el patrón y para promover una comprensión real, el generador de preguntas baraja las opciones de respuesta. Las preguntas se presentan una a la vez, seguidas de comentarios inmediatos sobre cada respuesta. Después de completar todas las preguntas, el calculate_quiz_results
El método evalúa las respuestas del usuario y se presenta al usuario una puntuación general, un desglose visual de las respuestas correctas versus las respuestas incorrectas y la retroalimentación sobre el nivel de rendimiento. De esta manera, la funcionalidad de la generación de preguntas transforma los videos pasivos en el aprendizaje activo al desafiar a los usuarios a recordar y aplicar información presentada en el video.
# quiz.py
class QuizGenerator:
def __init__(self):
pass
def generate_quiz(self, rag_system, api_key, transcript=None, model="gpt-4o", temperature=0.4):
"""
Generate quiz questions based on the video transcript
Args:
rag_system: The RAG system with vector store2
api_key: OpenAI API key
transcript: The full transcript text (optional)
model: Model to use for question generation
temperature: Creativity level (0.0-1.0)
Returns:
list: List of question objects
"""
if not rag_system:
st.error("Please transcribe the video first before creating a quiz!")
return ()
# Create a temporary LLM with slightly higher temperature for more creative questions
creative_llm = ChatOpenAI(
openai_api_key=api_key,
model=model,
temperature=temperature
)
num_questions = 10
# Prompt to generate quiz
prompt = f"""Based on the video transcript, generate {num_questions} multiple-choice questions to test understanding of the content.
For each question:
1. The question should be specific to information mentioned in the video
2. Include 4 options (A, B, C, D)
3. Clearly indicate the correct answer
Format your response exactly as follows for each question:
QUESTION: (question text)
A: (option A)
B: (option B)
C: (option C)
D: (option D)
CORRECT: (letter of correct answer)
Make sure all questions are based on facts from the video."""
try:
if transcript:
# If we have the full transcript, use it
messages = (
{"role": "system", "content": f"You are given the following transcript from a video:nn{transcript}nnUse this transcript to create quiz questions according to the instructions."},
{"role": "user", "content": prompt}
)
response = creative_llm.invoke(messages)
response_text = response.content
else:
# Fallback to RAG approach if no transcript is provided
relevant_docs = rag_system.vector_store.similarity_search(
"what are the main topics covered in this video?", k=5
)
context = "nn".join((doc.page_content for doc in relevant_docs))
# Use the creative LLM with context to generate questions
messages = (
{"role": "system", "content": f"You are given the following context from a video transcript:nn{context}nnUse this context to create quiz questions according to the instructions."},
{"role": "user", "content": prompt}
)
response = creative_llm.invoke(messages)
response_text = response.content
except Exception as e:
# Fallback to the regular RAG system if there's an error
st.warning(f"Using standard question generation due to error: {str(e)}")
response_text = rag_system.ask(prompt)
return self.parse_quiz_response(response_text)
# The rest of the class remains unchanged
def parse_quiz_response(self, response_text):
"""
Parse the LLM response to extract questions, options, and correct answers
Args:
response_text: Raw text response from LLM
Returns:
list: List of parsed question objects
"""
quiz_questions = ()
current_question = {}
for line in response_text.strip().split('n'):
line = line.strip()
if line.startswith('QUESTION:'):
if current_question and 'question' in current_question and 'options' in current_question and 'correct' in current_question:
quiz_questions.append(current_question)
current_question = {
'question': line(len('QUESTION:'):).strip(),
'options': (),
'correct': None
}
elif line.startswith(('A:', 'B:', 'C:', 'D:')):
option_letter = line(0)
option_text = line(2:).strip()
current_question.setdefault('options', ()).append((option_letter, option_text))
elif line.startswith('CORRECT:'):
current_question('correct') = line(len('CORRECT:'):).strip()
# Add the last question
if current_question and 'question' in current_question and 'options' in current_question and 'correct' in current_question:
quiz_questions.append(current_question)
# Randomize options for each question
randomized_questions = ()
for q in quiz_questions:
# Get the original correct answer
correct_letter = q('correct')
correct_option = None
# Find the correct option text
for letter, text in q('options'):
if letter == correct_letter:
correct_option = text
break
if correct_option is None:
# If we can't find the correct answer, keep the question as is
randomized_questions.append(q)
continue
# Create a list of options texts and shuffle them
option_texts = (text for _, text in q('options'))
# Create a copy of the original letters
option_letters = (letter for letter, _ in q('options'))
# Create a list of (letter, text) pairs
options_pairs = list(zip(option_letters, option_texts))
# Shuffle the pairs
random.shuffle(options_pairs)
# Find the new position of the correct answer
new_correct_letter = None
for letter, text in options_pairs:
if text == correct_option:
new_correct_letter = letter
break
# Create a new question with randomized options
new_q = {
'question': q('question'),
'options': options_pairs,
'correct': new_correct_letter
}
randomized_questions.append(new_q)
return randomized_questions
def calculate_quiz_results(self, questions, user_answers):
"""
Calculate quiz results based on user answers
Args:
questions: List of question objects
user_answers: Dictionary of user answers keyed by question_key
Returns:
tuple: (results dict, correct count)
"""
correct_count = 0
results = {}
for i, question in enumerate(questions):
question_key = f"quiz_q_{i}"
user_answer = user_answers.get(question_key)
correct_answer = question('correct')
# Only count as correct if user selected an answer and it matches
is_correct = user_answer is not None and user_answer == correct_answer
if is_correct:
correct_count += 1
results(question_key) = {
'user_answer': user_answer,
'correct_answer': correct_answer,
'is_correct': is_correct
}
return results, correct_count
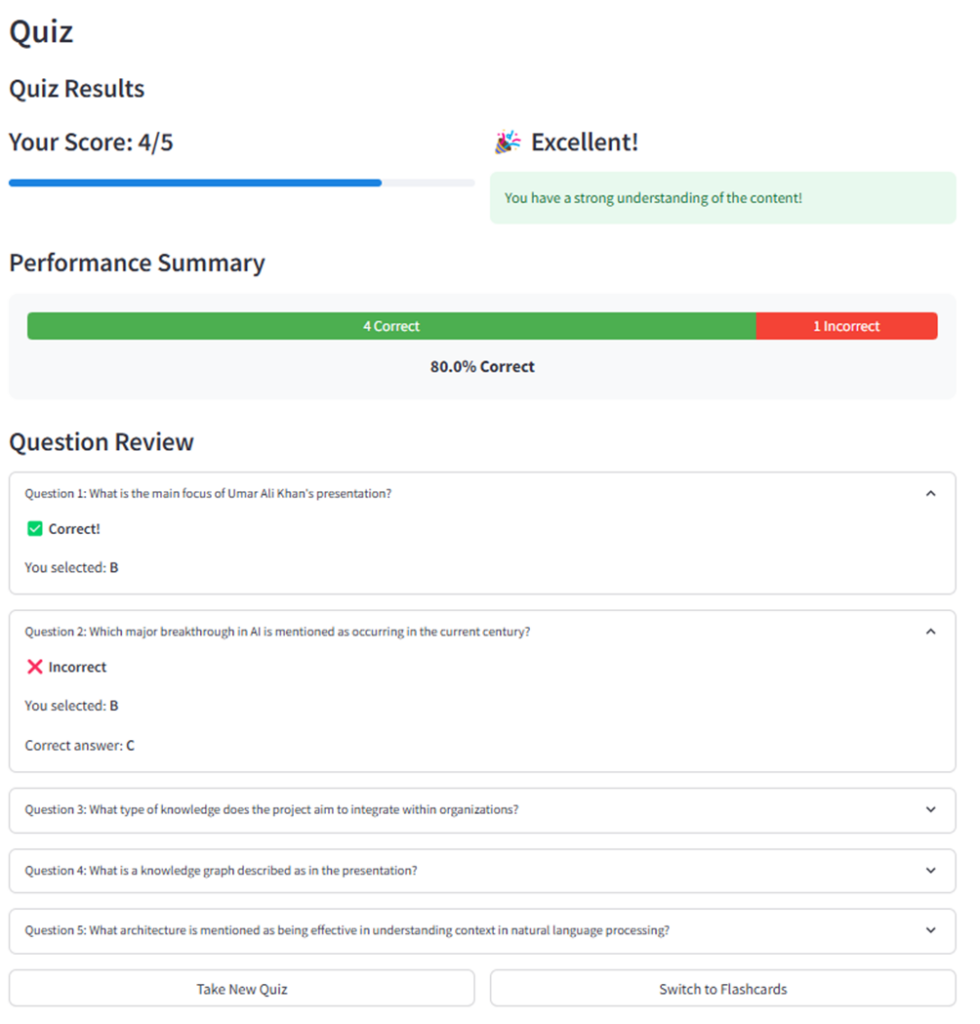
Talk-to-Videos también genera tarjetas de flash del contenido de video, que admiten técnicas de aprendizaje de repetición activo y recordatorio espaciado. Esto se hace a través del FlashcardGenerator
clasificar flashcards.py
, que crea una combinación de diferentes tarjetas centradas en definiciones clave de términos, preguntas conceptuales, declaraciones de relleno en blanco y preguntas verdaderas/falsas con explicaciones. Un aviso guía el LLM para emitir tarjetas de salida en un formato JSON estructurado, con cada tarjeta que contiene distintos elementos de “frontal” y “retroceso”. El shuffle_flashcards
produce una presentación aleatoria, y cada tarjeta de flash se valida para garantizar que contenga componentes frontales y posteriores antes de presentarse al usuario. La respuesta a cada tarjeta de flash está inicialmente oculta. Se revela en la entrada del usuario utilizando una funcionalidad de revelación de tarjetas clásicas. Los usuarios pueden generar un nuevo conjunto de tarjetas para más práctica. Los sistemas Flashcard y el cuestionario están interconectados entre sí para que los usuarios puedan cambiar entre ellos según sea necesario.
# flashcards.py
class FlashcardGenerator:
"""Class to generate flashcards from video content using the RAG system."""
def __init__(self):
"""Initialize the flashcard generator."""
pass
def generate_flashcards(self, rag_system, api_key, transcript=None, num_cards=10, model="gpt-4o") -> List(Dict(str, str)):
"""
Generate flashcards based on the video content.
Args:
rag_system: The initialized RAG system with video content
api_key: OpenAI API key
transcript: The full transcript text (optional)
num_cards: Number of flashcards to generate (default: 10)
model: The OpenAI model to use
Returns:
List of flashcard dictionaries with 'front' and 'back' keys
"""
# Import here to avoid circular imports
from langchain_openai import ChatOpenAI
# Initialize language model
llm = ChatOpenAI(
openai_api_key=api_key,
model=model,
temperature=0.4
)
# Create the prompt for flashcard generation
prompt = f"""
Create {num_cards} educational flashcards based on the video content.
Each flashcard should have:
1. A front side with a question, term, or concept
2. A back side with the answer, definition, or explanation
Focus on the most important and educational content from the video.
Create a mix of different types of flashcards:
- Key term definitions
- Conceptual questions
- Fill-in-the-blank statements
- True/False questions with explanations
Format your response as a JSON array of objects with 'front' and 'back' properties.
Example:
(
{{"front": "What is photosynthesis?", "back": "The process by which plants convert light energy into chemical energy."}},
{{"front": "The three branches of government are: Executive, Legislative, and _____", "back": "Judicial"}}
)
Make sure your output is valid JSON format with exactly {num_cards} flashcards.
"""
try:
# Determine the context to use
if transcript:
# Use the full transcript if provided
# Create messages for the language model
messages = (
{"role": "system", "content": f"You are an educational content creator specializing in creating effective flashcards. Use the following transcript from a video to create educational flashcards:nn{transcript}"},
{"role": "user", "content": prompt}
)
else:
# Fallback to RAG system if no transcript is provided
relevant_docs = rag_system.vector_store.similarity_search(
"key points and educational concepts in the video", k=15
)
context = "nn".join((doc.page_content for doc in relevant_docs))
# Create messages for the language model
messages = (
{"role": "system", "content": f"You are an educational content creator specializing in creating effective flashcards. Use the following context from a video to create educational flashcards:nn{context}"},
{"role": "user", "content": prompt}
)
# Generate flashcards
response = llm.invoke(messages)
content = response.content
# Extract JSON content in case there's text around it
json_start = content.find('(')
json_end = content.rfind(')') + 1
if json_start >= 0 and json_end > json_start:
json_content = content(json_start:json_end)
flashcards = json.loads(json_content)
else:
# Fallback in case of improper JSON formatting
raise ValueError("Failed to extract valid JSON from response")
# Verify we have the expected number of cards (or adjust as needed)
actual_cards = min(len(flashcards), num_cards)
flashcards = flashcards(:actual_cards)
# Validate each flashcard has required fields
validated_cards = ()
for card in flashcards:
if 'front' in card and 'back' in card:
validated_cards.append({
'front': card('front'),
'back': card('back')
})
return validated_cards
except Exception as e:
# Handle errors gracefully
print(f"Error generating flashcards: {str(e)}")
# Return a few basic flashcards in case of error
return (
{"front": "Error generating flashcards", "back": f"Please try again. Error: {str(e)}"},
{"front": "Tip", "back": "Try regenerating flashcards or using a different video"}
)
def shuffle_flashcards(self, flashcards: List(Dict(str, str))) -> List(Dict(str, str)):
"""Shuffle the order of flashcards"""
shuffled = flashcards.copy()
random.shuffle(shuffled)
return shuffled
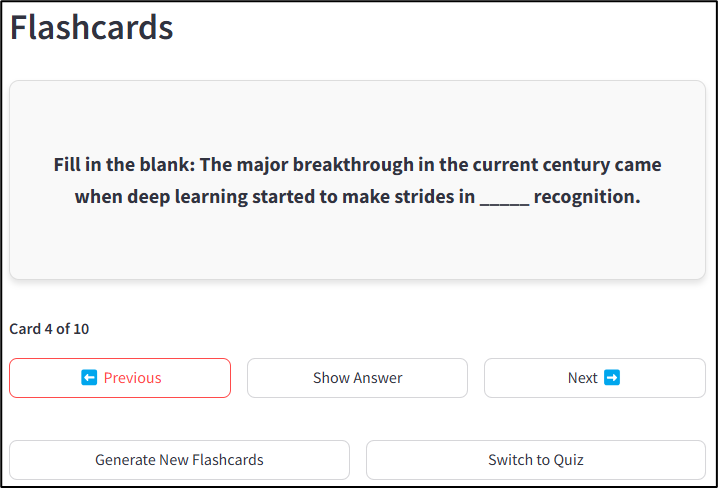
Posibles extensiones y mejoras
Esta aplicación se puede extender y mejorar de varias maneras. Por ejemplo:
- La integración de las características visuales en el video (como los fotogramas clave) se puede explorar con audio para extraer información más significativa.
- Las experiencias de aprendizaje basadas en el equipo se pueden habilitar donde los colegas o compañeros de clase pueden compartir notas, puntajes de cuestionarios y resúmenes.
- Creación de transcripciones navegables que permitan a los usuarios hacer clic en secciones específicas para saltar a ese punto en el video
- Creación de planes de acción paso a paso para implementar conceptos del video en configuraciones comerciales reales
- Modificando la solicitud de trapo para explicar las respuestas y proporcionar explicaciones más simples a conceptos difíciles.
- Generar preguntas que estimulen las habilidades metacognitivas en los alumnos al estimularlas a pensar en su proceso de pensamiento y estrategias de aprendizaje mientras se relacionan con el contenido de video.
¡Eso es toda la gente! Si te gustó el artículo, sígueme en Medio y LinkedIn.
(Tagstotranslate) Inteligencia artificial (T) Negocios (T) Editores Pick (T) Educación (T) Videos