Vision-language models (VLM) are becoming more common and offer substantial advances in ai-driven tasks. However, one of the most important limitations of these advanced models, including some notable ones like GPT-4V, is their limited spatial reasoning capabilities. Spatial reasoning involves understanding the positions of objects in three-dimensional space and their spatial relationships with each other. This limitation is particularly pronounced in real-world applications that require complex spatial analysis, such as robotics or augmented reality, where accurate spatial understanding is crucial.
Researchers at Google DeepMind and Google Research have pointed out that the fundamental limitation in spatial reasoning of VLMs does not originate in their architecture, but rather arises from the absence of comprehensive 3D spatial knowledge in the training data sets. To overcome this, they developed SpatialVLM, a novel system designed to improve the spatial reasoning capabilities of VLMs. This system was trained using a unique, large-scale spatial reasoning dataset. The dataset generation process involved a multifaceted framework that employed multiple models for open vocabulary detection, depth metric estimation, semantic segmentation, and object-centered captioning. These models worked together to extract detailed 3D spatial annotations from two-dimensional images, thus enriching the training data set with crucial spatial information.
SpatialVLM represents an important step forward in the field of VLMs. Your training in rich spatial data has greatly improved your ability to respond to qualitative and quantitative spatial queries. This capability was rigorously tested and validated through experiments, in which SpatialVLM consistently outperformed other vision and language models on spatial reasoning tasks. A notable aspect of SpatialVLM's performance is its ability to perform quantitative estimations accurately, a task that is often challenging due to the noisy nature of the training data. This feature makes it a valuable tool for open vocabulary reward annotators in complex robotic reordering tasks.
An innovative application of SpatialVLM is its integration with a powerful large language model, which allows you to perform chain-of-thought spatial reasoning. This ability to process and solve multi-step spatial reasoning tasks further expands its applicability in robotics and other domains that require sophisticated spatial analysis. Researchers have explored new downstream applications in spatial reasoning and robotics, demonstrating the potential of SpatialVLM as a dense reward annotator and success detector for various robotic tasks.
SpatialVLM significantly improves the ability of VLMs to answer both qualitative and quantitative spatial questions. This improved capability is demonstrated through experiments in which SpatialVLM outperforms other vision and language models on spatial reasoning tasks. Despite noisy training data, it can reliably make quantitative estimates, making it a valuable tool for open vocabulary reward annotators for reordering tasks in robotics.
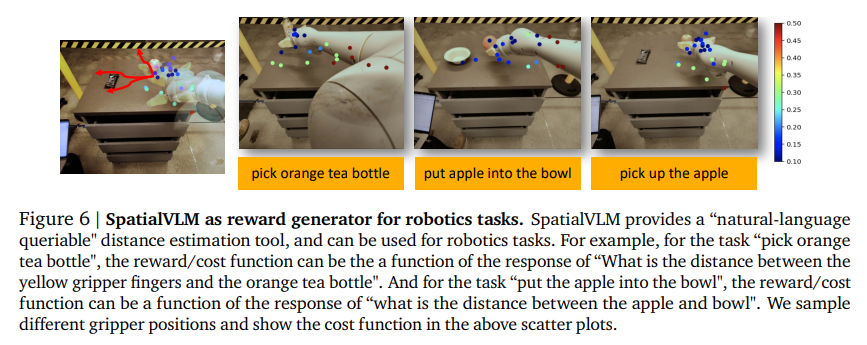
In conclusion, the key conclusions of the research can be presented as follows:
- SpatialVLM improves spatial reasoning in vision and language models.
- It was trained using a large-scale dataset enriched with 3D spatial annotations.
- The model excels in spatial reasoning tasks, outperforming other VLMs.
- SpatialVLM can perform complex chain-of-thought spatial reasoning, which is valuable in robotics.
- The development of SpatialVLM marks a significant advancement in ai technology.
Review the Paper and Project. All credit for this research goes to the researchers of this project. Also, don't forget to follow us on Twitter. Join our 36k+ ML SubReddit, 41k+ Facebook community, Discord channeland LinkedIn Grabove.
If you like our work, you will love our Newsletter..
Don't forget to join our Telegram channel
Hello, my name is Adnan Hassan. I'm a consulting intern at Marktechpost and soon to be a management trainee at American Express. I am currently pursuing a double degree from the Indian Institute of technology, Kharagpur. I am passionate about technology and I want to create new products that make a difference.
<!– ai CONTENT END 2 –>